HyperSDFusion: Bridging Hierarchical Structures in Language and Geometry for Enhanced 3D Text2Shape Generation
2024 IEEE/CVF Conference on Computer Vision and Pattern Recognition (CVPR)(2024)
摘要
3D shape generation from text is a fundamental task in 3D representationlearning. The text-shape pairs exhibit a hierarchical structure, where ageneral text like "chair" covers all 3D shapes of the chair, while moredetailed prompts refer to more specific shapes. Furthermore, both text and 3Dshapes are inherently hierarchical structures. However, existing Text2Shapemethods, such as SDFusion, do not exploit that. In this work, we proposeHyperSDFusion, a dual-branch diffusion model that generates 3D shapes from agiven text. Since hyperbolic space is suitable for handling hierarchical data,we propose to learn the hierarchical representations of text and 3D shapes inhyperbolic space. First, we introduce a hyperbolic text-image encoder to learnthe sequential and multi-modal hierarchical features of text in hyperbolicspace. In addition, we design a hyperbolic text-graph convolution module tolearn the hierarchical features of text in hyperbolic space. In order to fullyutilize these text features, we introduce a dual-branch structure to embed textfeatures in 3D feature space. At last, to endow the generated 3D shapes with ahierarchical structure, we devise a hyperbolic hierarchical loss. Our method isthe first to explore the hyperbolic hierarchical representation fortext-to-shape generation. Experimental results on the existing text-to-shapepaired dataset, Text2Shape, achieved state-of-the-art results.
更多查看译文
关键词
3D shape generation,Diffusion model,Hyperbolic representaion learning
AI 理解论文
溯源树
样例
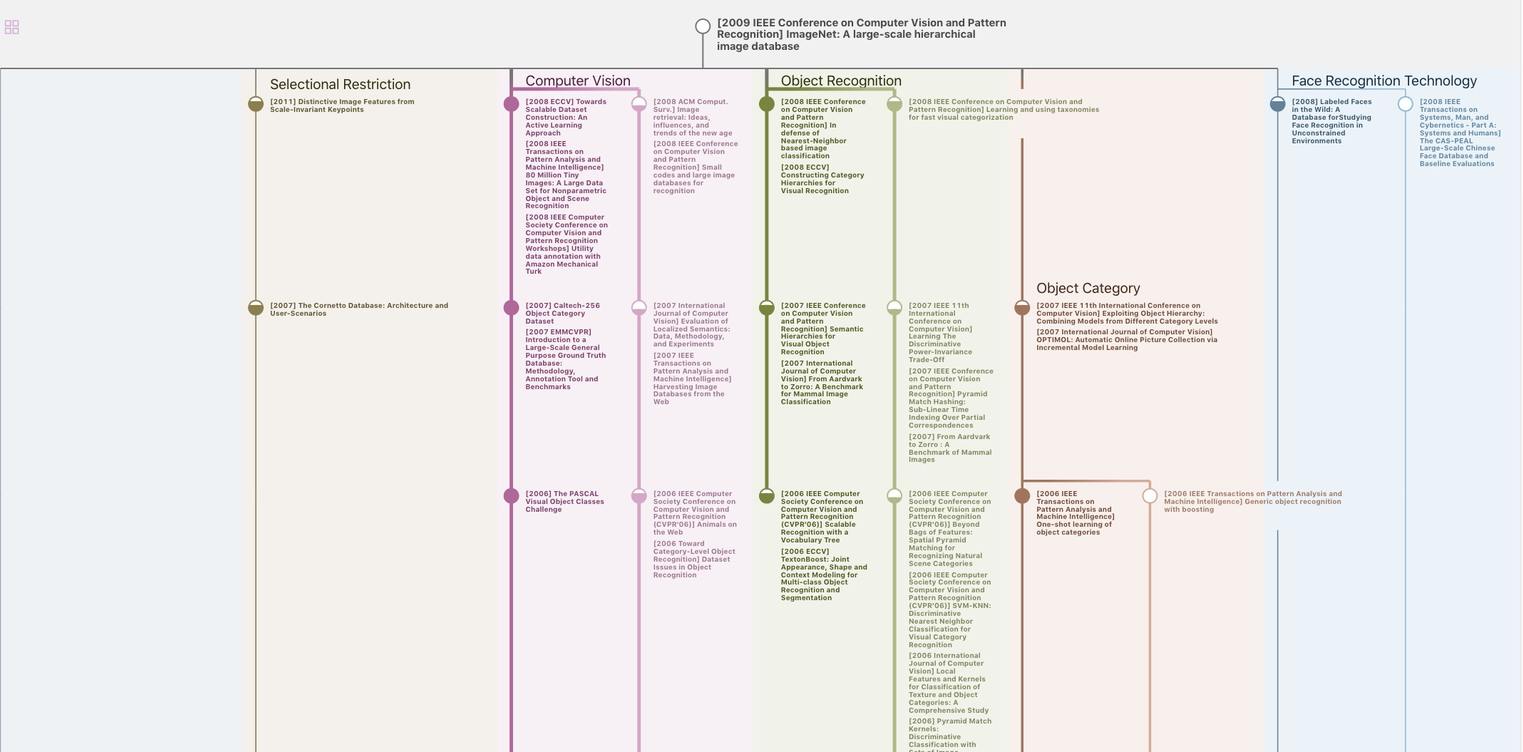
生成溯源树,研究论文发展脉络
Chat Paper
正在生成论文摘要