Compressing Multiple Sensor Signals for Distributed Sensing by Autoencoder Preprocessing
2023 5TH INTERNATIONAL CONFERENCE ON CONTROL AND ROBOTICS, ICCR(2023)
摘要
This paper proposes an autoencoder-based approach to effectively extract sensor features by leveraging an autoencoder as a data preprocessing method. The autoencoder constrains the hidden units in a bottleneck structure, resulting in a compressed knowledge representation of sensor readings. In the latent space representation, the encoded data learns and describes the most prominent latent attributes of sensor readings. The algorithm is experimentally validated in a real-world setting, demonstrating its effectiveness in accurately extracting relevant features from sensor data. Nine flexible bending sensors are utilized for posture sensing of a bellow-shaped fluidic elastomer actuator. Compared to previous studies, the results demonstrate that valuable features can be extracted without employing a large dropout rate for overfitting prevention, while maintaining prediction accuracy and reducing the entire sensor signals to half. Additionally, the training time is reduced by 7.2%. By providing a reduced and featured input to the regression neural network, the proposed approach not only prevents overfitting but also alleviates the computational redundancy and complexity brought by an increasing number of sensors.
更多查看译文
关键词
autoencoder,flexible sensor,distributed sensing,soft robotics,deep learning
AI 理解论文
溯源树
样例
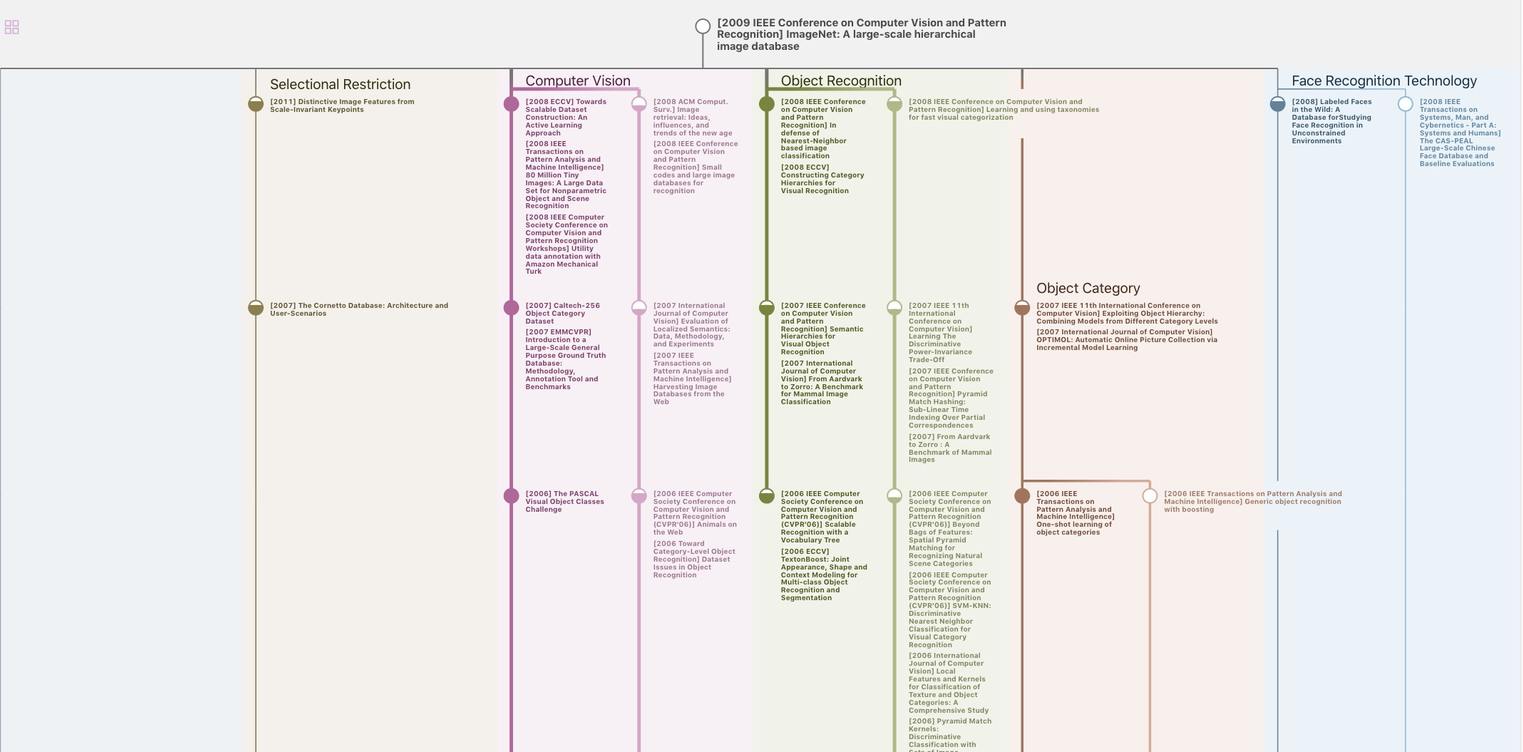
生成溯源树,研究论文发展脉络
Chat Paper
正在生成论文摘要