Electrical Impedance Tomography Deep Imaging with Dual-branch U-Net Based on Deformable Convolution and Hyper-Convolution
IEEE TRANSACTIONS ON INSTRUMENTATION AND MEASUREMENT(2024)
摘要
Electrical impedance tomography (EIT) is a new imaging modality for nonionizing radiation that uses a safe current applied to the surface and measures the response voltage on a boundary sensor to solve an inverse problem of determining the conductivity distribution in the region of interest (ROI). Due to the "soft-field" nature of the electrical field, the boundaries of the inclusions are usually blurred and the conductivity parameters are inaccurate in the reconstructions. To address the above problems, this article proposes a learning-based two-branch U-Net deep imaging architecture, named DHU-Net, for the accurate and sharp reconstruction of EIT images. Specifically, deformable convolution layers are introduced to improve the representation of shape and spatial information by a small convolutional kernel, while SE-Attention is used to recalibrate the channel-wise features for global distributions; on the other hand, an implicit hyper-convolutional network with coordinate attention (CA) is used to construct the relationship between the spatial coordinates of the convolutional kernel and corresponding weights, so that the large convolutional kernel for conductivity recovery has a relatively lower parameter while having better convolutional robustness. DHU-Net is trained and fine-tuned using a large number of simulation samples and validated on tank experiments. The metrics show that the RMSE is 2.0637, the SSIM is 0.9522, and the RSNR is 46.4597 (the metrics are lower than those of the TR method by 68.76%, 56.53%, and 61.00%) which provides better reconstruction visualization and consistency between the quantification metrics and reconstruction results compared to the state-of-the-art models. The experimental results show that the DHU-Net proposed in this article has better robustness and reconstruction consistency, suggesting that deformable convolution and implicit neural networks have better expressive capabilities in shape modeling, as well as less computational costs and faster inference than dense-based models, which can drive real-time applications of EIT for structural and functional imaging.
更多查看译文
关键词
Attention methods,deformable convolution,electrical impedance tomography (EIT),hyper-convolution,U-Net
AI 理解论文
溯源树
样例
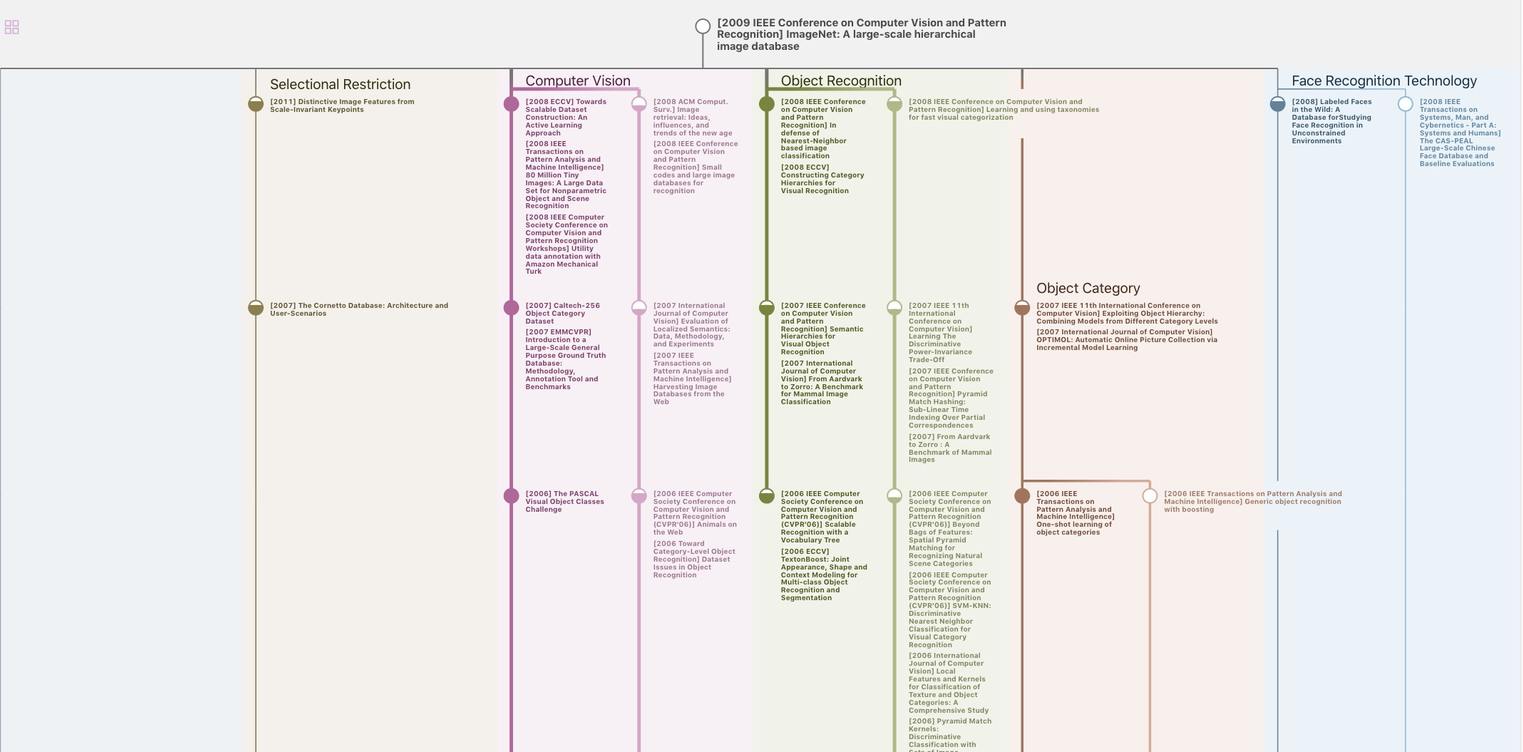
生成溯源树,研究论文发展脉络
Chat Paper
正在生成论文摘要