An Efficient FrWT and IPCA Tools for an Automated Healthcare CAD System
Wireless Personal Communications(2023)
摘要
Continuous monitoring of physiological parameters is critical in order to minimize casualties, therefore automated healthcare computer-assisted diagnostic systems require efficient signal processing techniques. It is currently the primary concern upon which the majority of research is concentrated. It is becoming a way of life to reach long age by which accurate and error-free treatments are done. Electrocardiograms (ECGs) are crucial in the current era for minimizing the global mortality rate. An essential clinical feature of electrocardiograms (ECGs) is the determination of heart rate. It is measured in beats per minute. It differs based on age and a number of other associated variables, including body position, temperature, emotion, smoke, and hormonal imbalances, among others. Arrhythmia of the heart, characterised by an irregular heart rate, is currently the leading cause of increased mortality. The complete diagnosis of ECG is accomplished using the blocks such as pre-processing, feature extraction, and detection/classification. Unfortunately, the analysis of ECG becomes complex under the wide variety of the recording datasets which is influenced by variety of noises and artifacts. It motivates to choose a novel combination of above-mentioned blocks which can serve the purpose. The fractional wavelet transform (FrWT) is chosen in conjunction with independent principal component analysis (IPCA) in this article. FrWT, which combines the fractional Fourier transform and the wavelet transform, satisfies the requirements for feature extraction and pre-processing, respectively, whereas IPCA satisfies the requirement for detection and classification. It also helps to understand the cardiac and non-cardiac behaviour of the electrical recording of the specific critical cases.
更多查看译文
关键词
Computer aided diagnostic (CAD) system,Electrocardiogram (ECG),Fractional wavelet transform (FrWT),Pre-processing,Feature extraction and detection
AI 理解论文
溯源树
样例
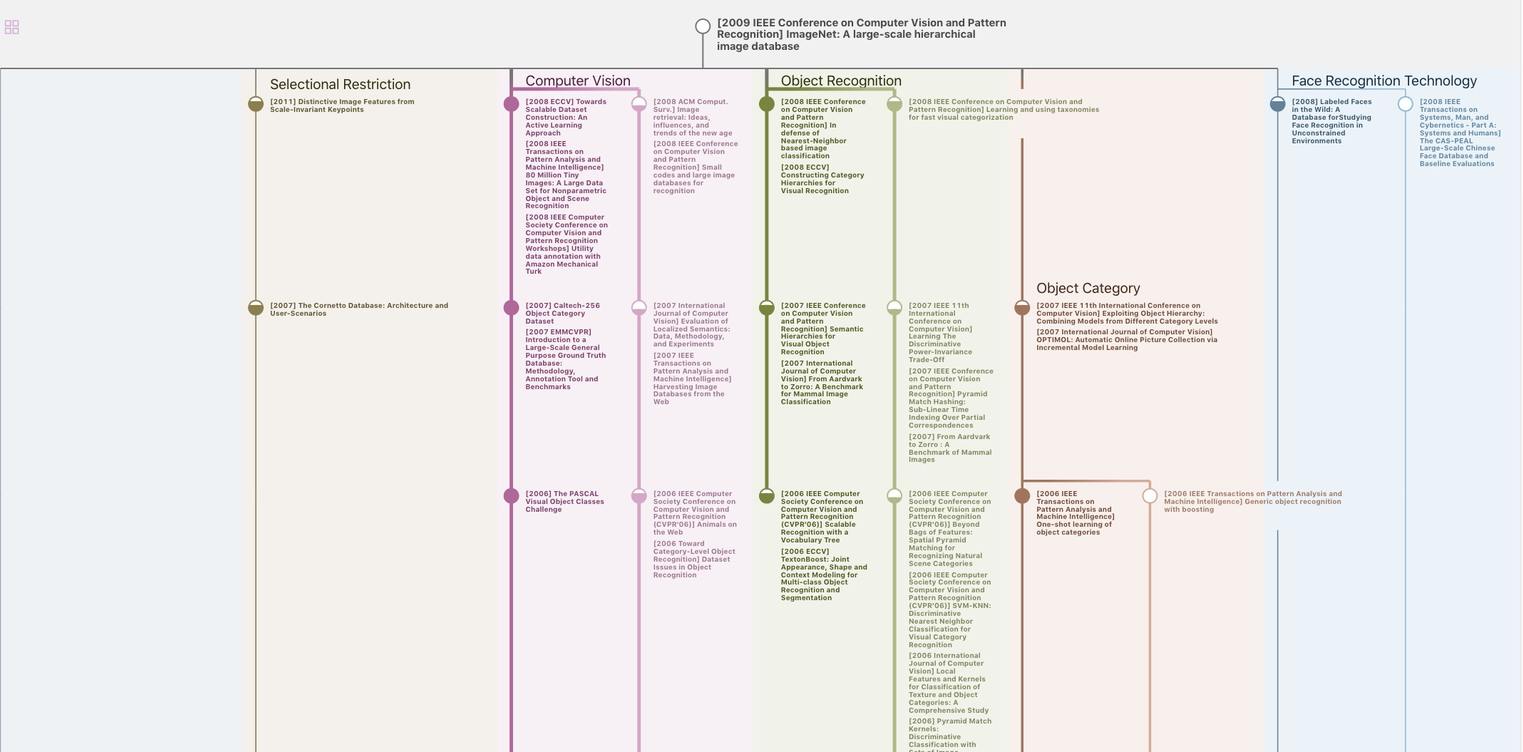
生成溯源树,研究论文发展脉络
Chat Paper
正在生成论文摘要