Dynamic System Modeling Using a Multisource Transfer Learning-Based Modular Neural Network for Industrial Application.
IEEE transactions on industrial informatics(2024)
Abstract
Establishing an accurate model of dynamic systems poses a challenge for complex industrial processes. Due to the ability to handle complex tasks, modular neural networks (MNN) have been widely applied to industrial process modeling. However, the phenomenon of domain drift caused by operating conditions may lead to a cold start of the model, which affects the performance of MNN. For this reason, a multisource transfer learning-based MNN (MSTL-MNN) is proposed in this study. First, the knowledge-driven transfer learning process is performed with domain similarity evaluation, knowledge extraction, and fusion, aiming to form an initial subnetwork in the target domain. Then, the positive transfer process of effective knowledge can avoid the cold start problem of MNN. Second, during the data-driven fine-tuning process, a regularized self-organizing long short-term memory algorithm is designed to fine-tune the structure and parameters of the initial subnetwork, which can improve the prediction performance of MNN. Meanwhile, relevant theoretical analysis is given to ensure the feasibility of MSTL-MNN. Finally, the effectiveness of the proposed method is confirmed by two benchmark simulations and a real industrial dataset of a municipal solid waste incineration process. Experimental results demonstrate the merits of MSTL-MNN for industrial applications.
MoreTranslated text
Key words
Multi-layer neural network,Task analysis,Neurons,Dynamical systems,Computational modeling,Prediction algorithms,Mathematical models,Dynamic system,long short-term memory (LSTM),modular neural network (MNN),multisource transfer learning
AI Read Science
Must-Reading Tree
Example
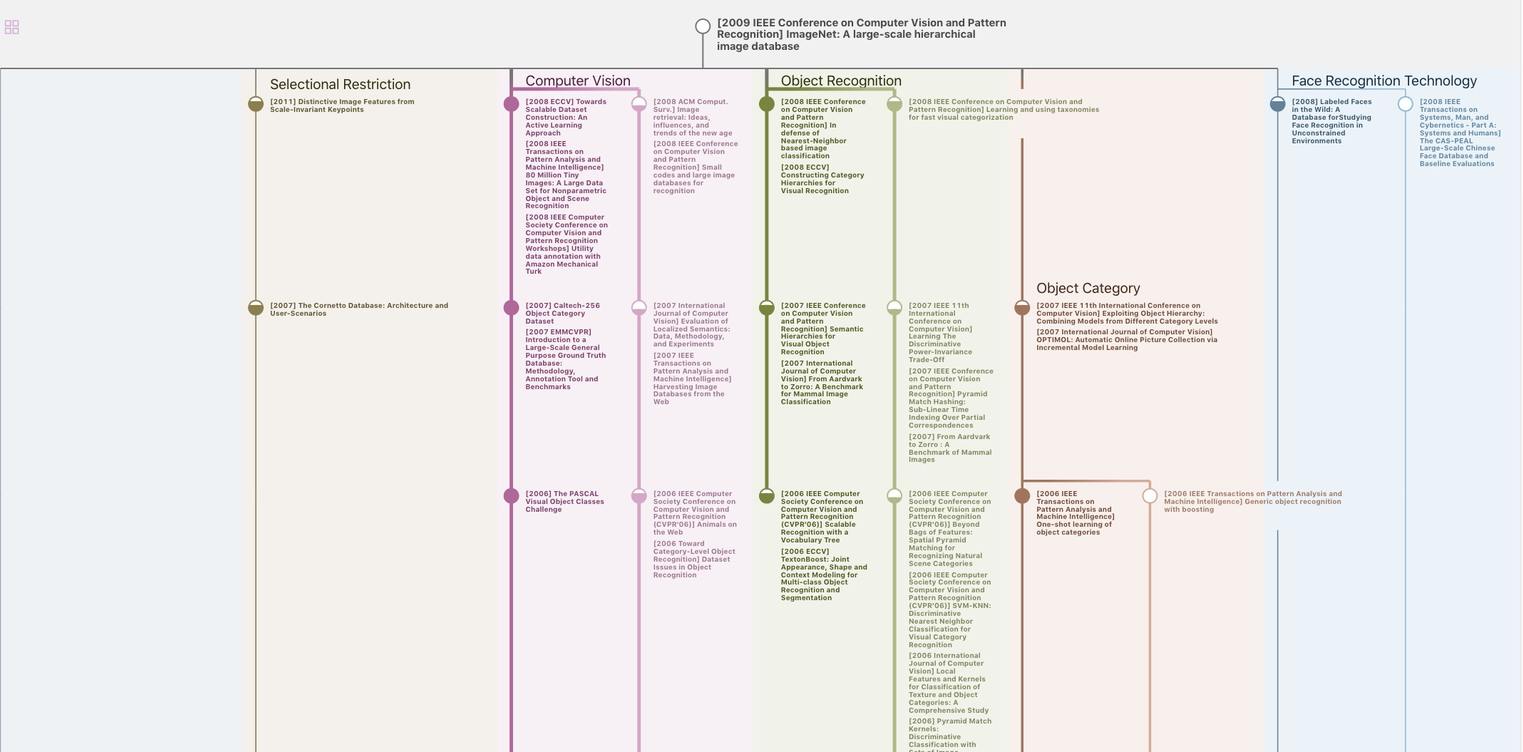
Generate MRT to find the research sequence of this paper
Chat Paper
Summary is being generated by the instructions you defined