Autopilot Controller of Fixed-Wing Planes Based on Curriculum Reinforcement Learning Scheduled by Adaptive Learning Curve
IEEE TRANSACTIONS ON EMERGING TOPICS IN COMPUTATIONAL INTELLIGENCE(2024)
摘要
In this paper, we present a novel curriculum reinforcement learning method that can automatically generate a high-performance autopilot controller for a 6-degree-of-freedom (6-DOF) aircraft with an unknown dynamic model, which is difficult to be handled using traditional control methods. In this method, a sigmoid-like learning curve is elegantly introduced to generate goals (the desired heading, altitude, and velocity) from easy to hard for autopilot. The shape of the learning curve can be intelligently adjusted to adapt to the training process of Proximal Policy Optimization (PPO). In addition, the conflict between multiple goals in autopilot training is solved by designing an adaptive reward function. Furthermore, the control inputs can avoid large oscillations by filtering the outputs from PPO with a first-order filter to ensure the smoothness. A series of simulation results show that the proposed method can not only observably improve the success rate and stability of training but also has superior performance in settling time and robustness compared with the traditional PID control and a state-of-the-art (SOTA) method. In the end, the applications of the controller, including the navigation task, pursuit-evasion, and dogfighting, are demonstrated to prove its feasibility to multiple tasks.
更多查看译文
关键词
Autopilot control,deep reinforcement learning,fixed-wing plane,learning curve
AI 理解论文
溯源树
样例
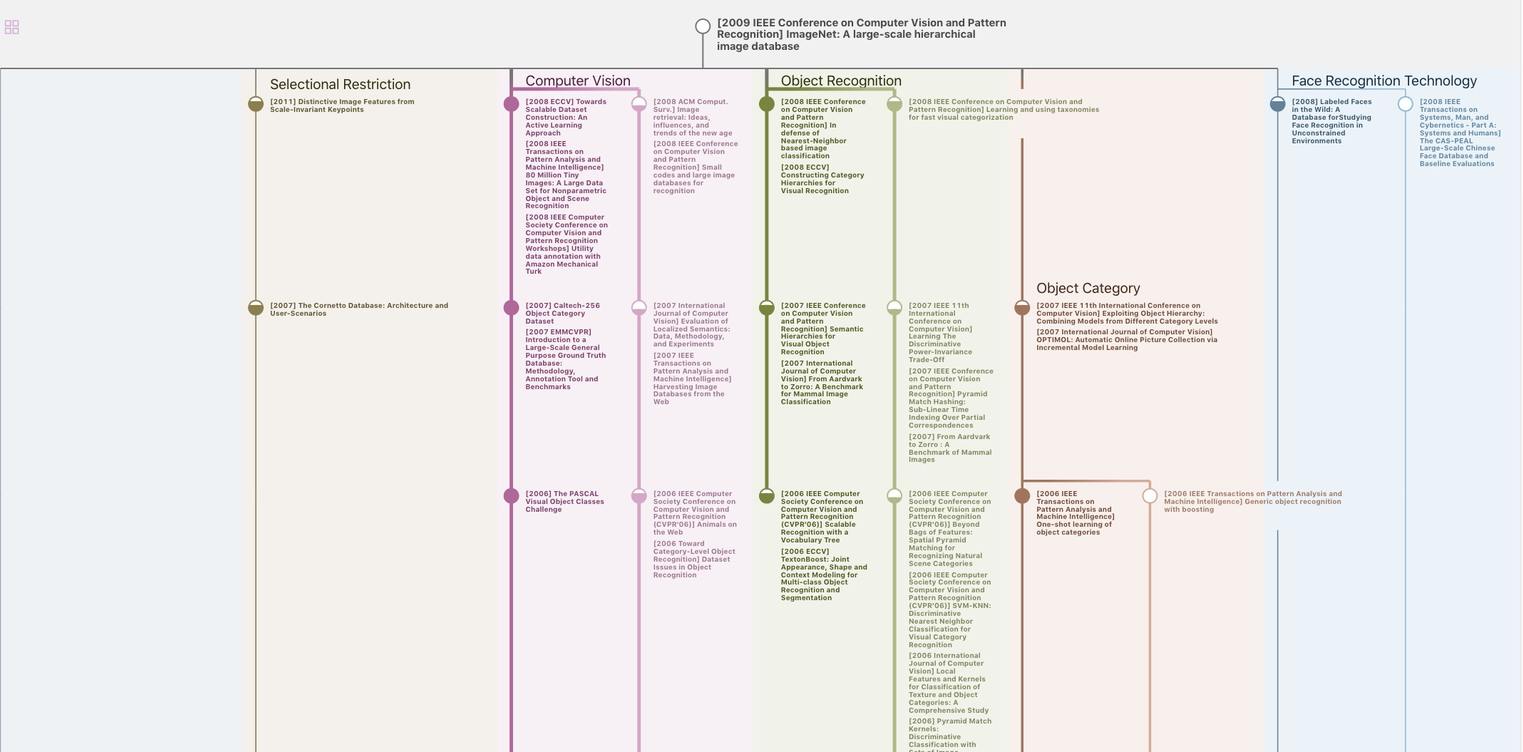
生成溯源树,研究论文发展脉络
Chat Paper
正在生成论文摘要