Accurate Modeling of GaN HEMTs Oriented to Analysis of Kink Effects in S22 and H21: an Effective Machine Learning Approach
IEEE JOURNAL OF THE ELECTRON DEVICES SOCIETY(2024)
Abstract
In this work, for the first time, a machine learning behavioral modeling methodology based on gate recurrent unit (GRU) is developed and used to model and then analyze the kink effects (KEs) in the output reflection coefficient (S22) and the short-circuit current gain (h21) of an advanced microwave transistor. The device under test (DUT) is a 0.25-μm gallium nitride (GaN) high electron mobility transistor (HEMT) on silicon carbide (SiC) substrate, which has a large gate periphery of 1.5 mm. The scattering (S-) parameters of the DUT are measured at a frequency up to 65 GHz and at an ambient temperature up to 200∘C. The proposed model can accurately reproduce the KEs in S22 and in h21, enabling an effective analysis of their dependence on the operating conditions, bias point and ambient temperature. It is worth noticing that the proposed transistor model shows also good performance in both interpolation and extrapolation test.
MoreTranslated text
Key words
GaN HEMT,GRU,kink effect,machine learning methods,semiconductor device modeling,scattering parameter measurements,temperature
求助PDF
上传PDF
View via Publisher
AI Read Science
Must-Reading Tree
Example
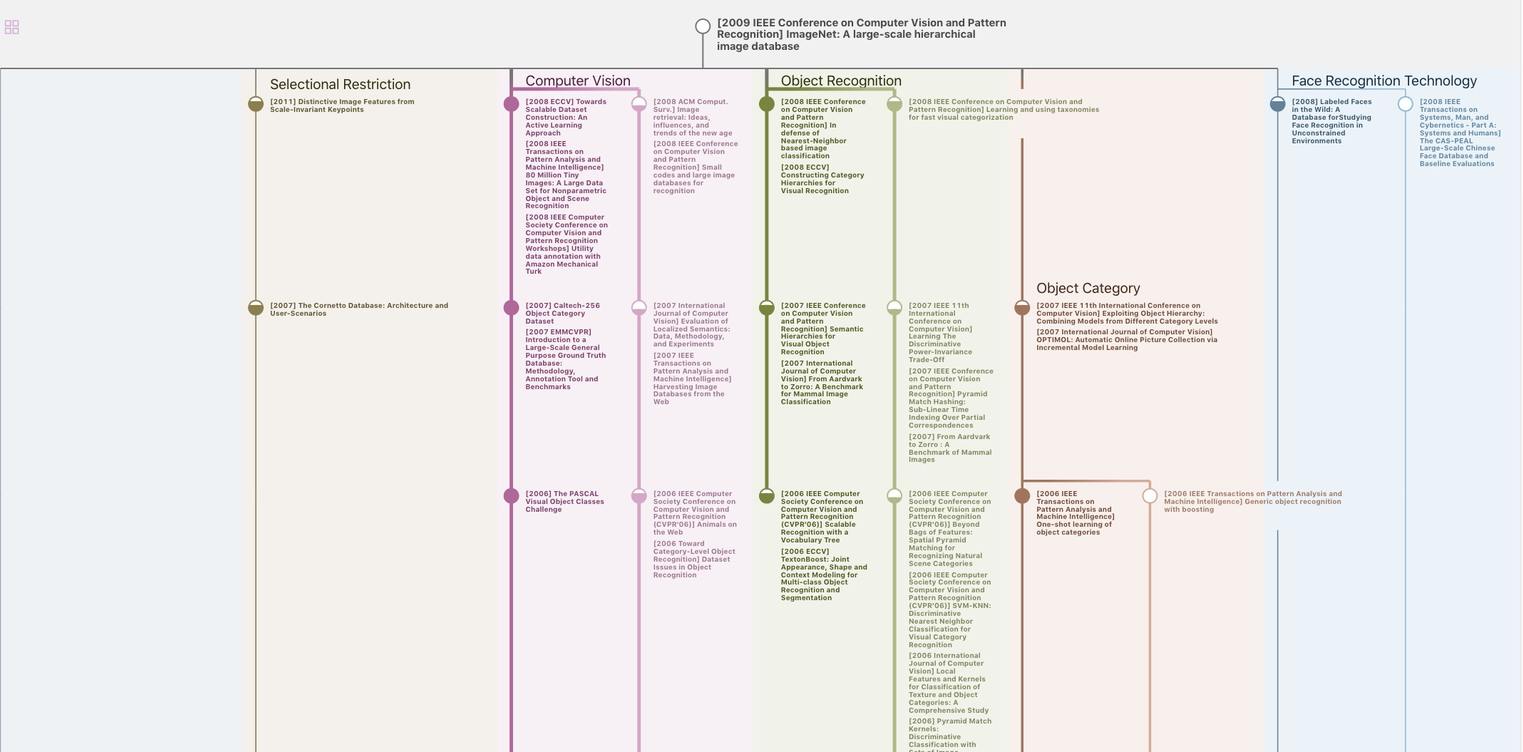
Generate MRT to find the research sequence of this paper
Related Papers
Data Disclaimer
The page data are from open Internet sources, cooperative publishers and automatic analysis results through AI technology. We do not make any commitments and guarantees for the validity, accuracy, correctness, reliability, completeness and timeliness of the page data. If you have any questions, please contact us by email: report@aminer.cn
Chat Paper
GPU is busy, summary generation fails
Rerequest