Medicine Package Recommendation Via Dual-level Interaction Aware Heterogeneous Graph
IEEE journal of biomedical and health informatics(2024)
摘要
Medicine package recommendation aims to assist doctors in clinical decision-making by recommending appropriate packages of medicines for patients. Current methods model this task as a multi-label classification or sequence generation problem, focusing on learning relationships between individual medicines and other medical entities. However, these approaches uniformly overlook the interactions between medicine packages and other medical entities, potentially resulting in a lack of completeness in recommended medicine packages. Furthermore, medicine commonsense knowledge considered by current methods is notably limited, making it challenging to delve into the decision-making processes of doctors. To solve these problems, we propose DIAGNN, a Dual-level Interaction Aware heterogeneous Graph Neural Network for medicine package recommendation. Specifically, DIAGNN explicitly models interactions of medical entities within electronic health records(EHRs) at two levels, individual medicine and medicine package, leveraging a heterogeneous graph. A dual-level interaction aware graph convolutional network is utilized to capture semantic information in the medical heterogeneous graph. Additionally, we incorporate medication indications into the medical heterogeneous graph as medicine commonsense knowledge. Extensive experimental results on real-world datasets validate the effectiveness of the proposed method.
更多查看译文
关键词
Recommendation system,healthcare,heterogeneous graph,representation learning
AI 理解论文
溯源树
样例
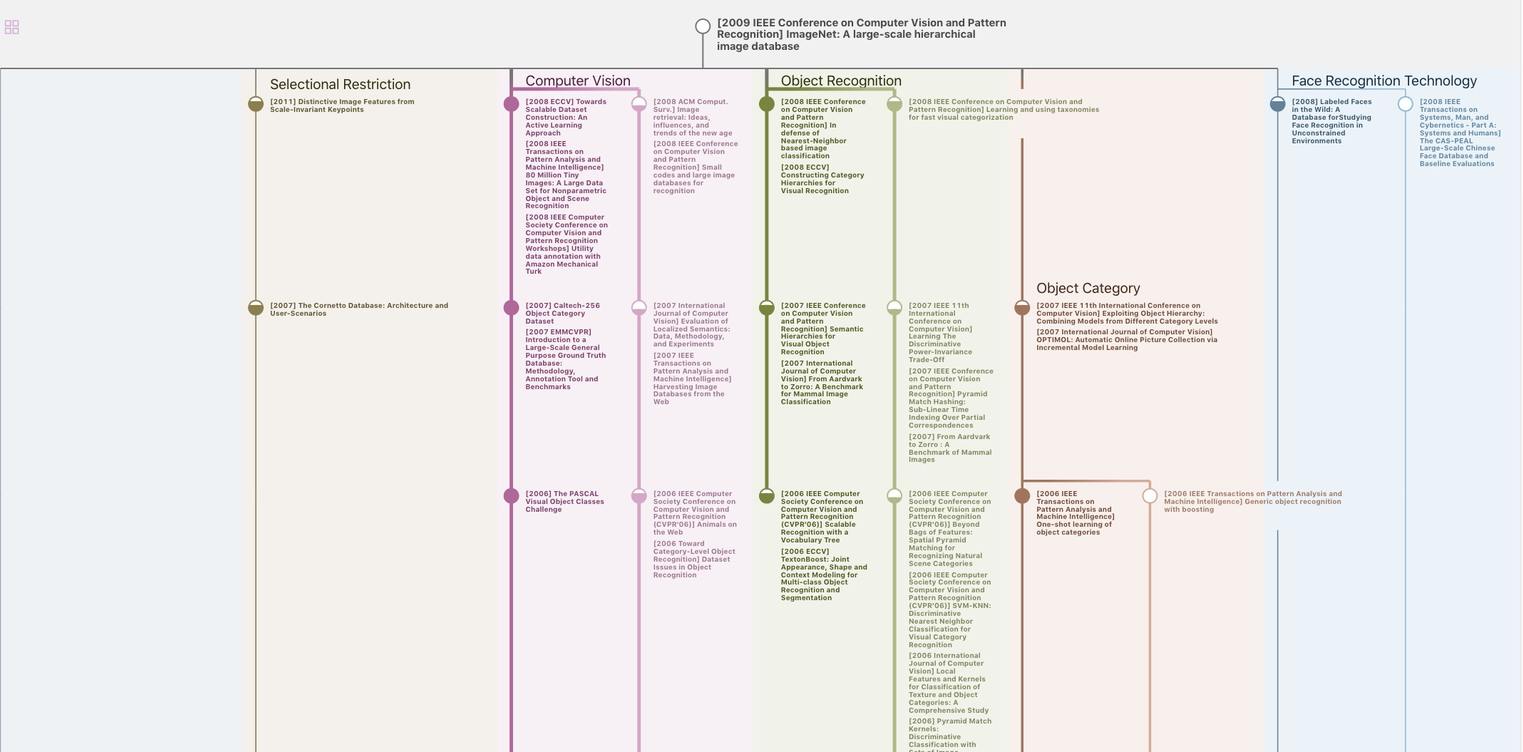
生成溯源树,研究论文发展脉络
Chat Paper
正在生成论文摘要