Abstract 1615: Balanced Molecular Feature Selection Unveils Universally Tumor-Lineage Informative Methylation Sites in Colorectal Cancer
Cancer research(2024)
摘要
In the era of precision medicine, performing comparative analysis over diverse patient populations is a fundamental step towards tailoring healthcare interventions. However, the critical aspect of equitably selecting molecular features across multiple patients is often overlooked. To address this challenge, we introduce FALAFL (FAir muLti-sAmple Feature seLection), an algorithmic approach based on combinatorial optimization. FALAFL is designed to bridge the gap between molecular feature selection and algorithmic fairness, ensuring a fair selection of molecular features from all patient samples in a cohort.We have applied FALAFL to the problem of selecting lineage-informative CpG sites within a cohort of colorectal cancer patients subjected to low read coverage single-cell methylation sequencing. Our results demonstrate that FALAFL can rapidly and robustly determine the optimal set of CpG sites, which are each well covered by cells across the vast majority of the patients, while ensuring that in each patient a high proportion of these sites have good read coverage. An analysis of the FALAFL-selected sites reveals that their tumor lineage-informativeness exhibits a strong correlation across a spectrum of diverse patient profiles. Furthermore, these universally lineage-informative sites are highly enriched in the inter CpG island regions.FALAFL integrates equity considerations into the molecular feature selection from single-cell sequencing data obtained from a patient cohort. We hope that it will help propel equitable healthcare data science practices and contribute to the advancement of our understanding of complex diseases.### Competing Interest StatementThe authors have declared no competing interest.
更多查看译文
AI 理解论文
溯源树
样例
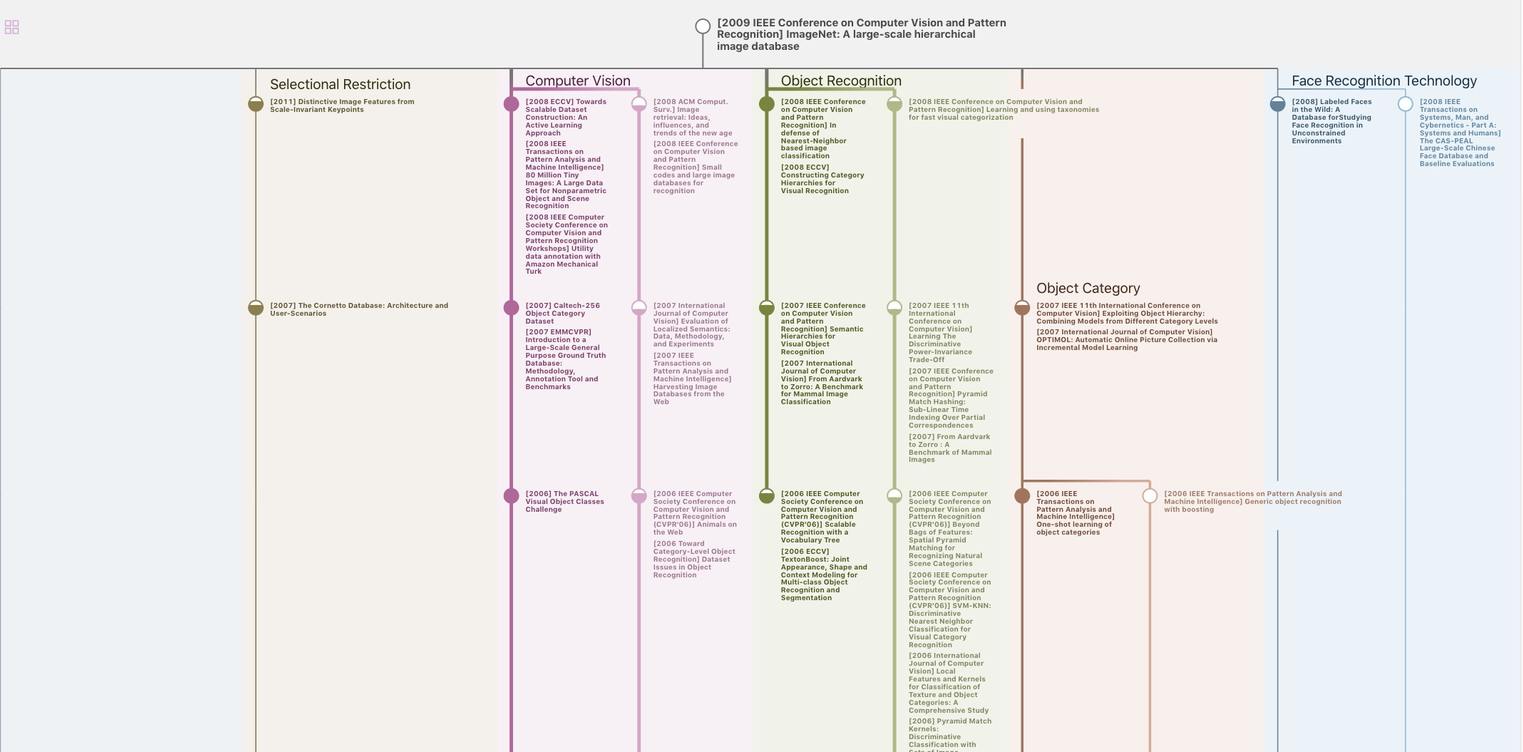
生成溯源树,研究论文发展脉络
Chat Paper
正在生成论文摘要