Towards a digital twin framework in additive manufacturing: Machine learning and bayesian optimization for time series process optimization
Journal of Manufacturing Systems(2024)
摘要
Laser directed-energy deposition (DED) offers notable advantages in additive manufacturing (AM) for producing intricate geometries and facilitating material functional grading. However, inherent challenges such as material property inconsistencies and part variability persist, predominantly due to its layer-wise fabrication approach. Critical to these challenges is heat accumulation during DED, influencing the resultant material microstructure and properties. Although closed-loop control methods for managing heat accumulation and temperature regulation are prevalent in DED literature, few approaches integrate real-time monitoring, physics-based modeling, and control simultaneously in a cohesive framework. To address this, we present a digital twin (DT) framework for real-time model predictive control of process parameters of the DED for achieving a specific process design objective. To enable its implementation, we detail the development of a surrogate model utilizing Long Short-Term Memory (LSTM)-based machine learning which uses Bayesian Inference to predict temperatures across various spatial locations of the DED-built part. This model offers real-time predictions of future temperature states. In addition, we introduce a Bayesian Optimization (BO) method for Time Series Process Optimization (BOTSPO). Its foundational principles align with traditional BO, and its novelty lies in our unique time series process profile generator with a reduced dimensional representation. BOTSPO is used for dynamic process optimization in which we deploy BOTSPO to determine the optimal laser power profile, aiming to achieve desired mechanical properties in a DED build. The identified profile establishes a process trajectory that online process optimizations aim to match or exceed in performance. This paper elucidates components of the digital twin framework, advocating its prospective consolidation into a comprehensive digital twin system for AM.
更多查看译文
关键词
Directed energy deposition,Additive manufacturing,Digital twin,Process optimization,Long-short term memory,Recurrent neural network,Bayesian optimization
AI 理解论文
溯源树
样例
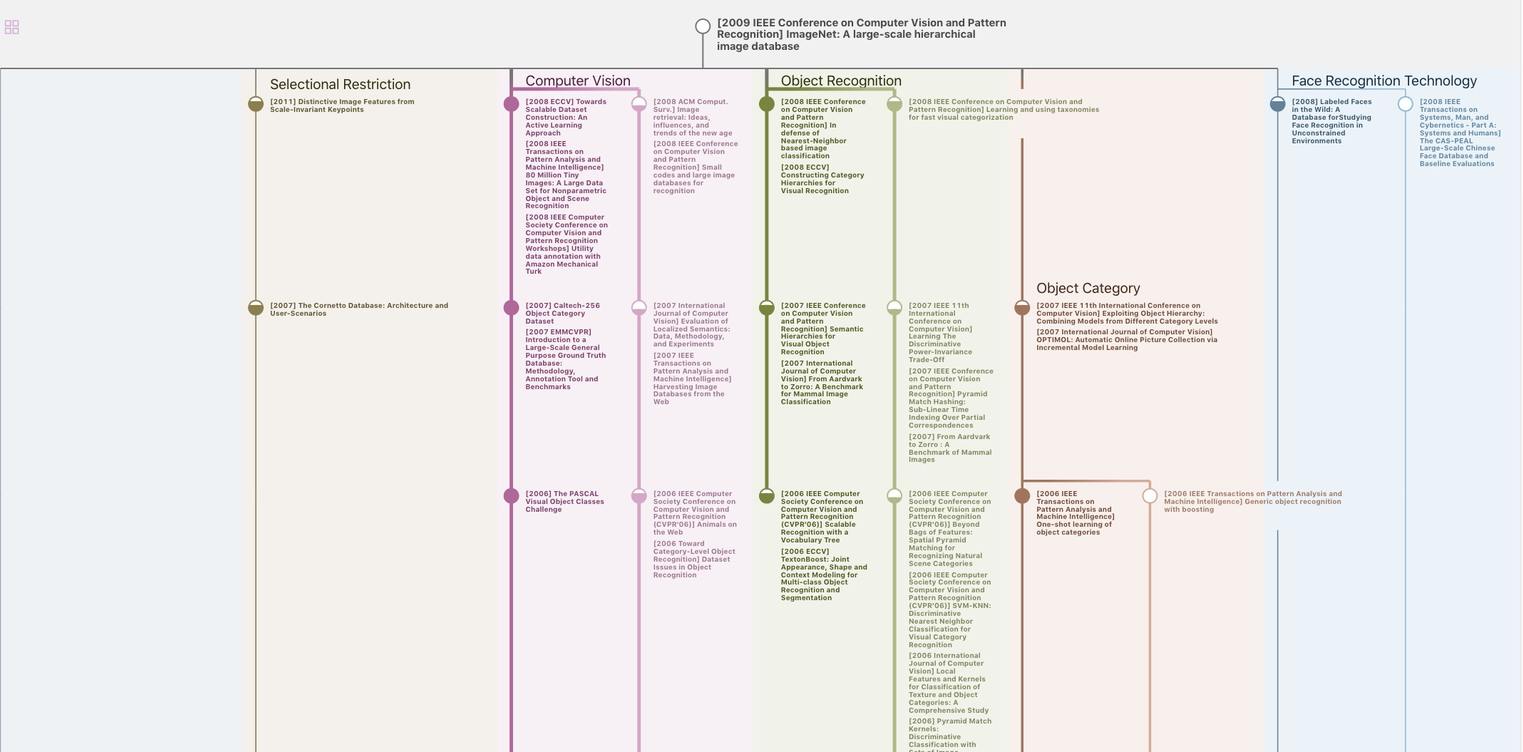
生成溯源树,研究论文发展脉络
Chat Paper
正在生成论文摘要