An Integration of Meta-Heuristic Approach Utilizing Kernel Principal Component Analysis for Multimodal Medical Image Registration
Cluster Computing(2024)
摘要
Medical image registration is vital for precise healthcare diagnosis, treatment planning, and disease progression tracking, but traditional methods fail to capture complex spatial transformations and anatomical variations. A Kernel Principal Component Analysis (KPCA) driven Teaching Learning based optimization (TLBO) approach is proposed to overcome these limitations. The proposed approach is categorized into three phases, i.e., pre-processed phase, contour extraction phase with feature extraction using KPCA, and evaluating robust affine transformation parameters leveraging TLBO for accurate alignment. In the pre-processing phase, gaussian filter is applied to remove noise from source and target images, followed by normalization process. Afterwards, contour extraction is carried out to create a feature image that represents the boundaries of an image. Centroid localization is then utilized to compute translation parameters, which determine the spatial alignment between the images. By utilizing KPCA, this method captures non-linear relationships in the data to enhance the representation of image features. TLBO is employed to optimize the rigid transformation parameters to improve the accurate alignment of source and target images. Extensive experiments are carried out on monomodal and multimodal medical images such as CT and MRI taken from the Harvard Brain ATLAS, Kaggle as well as in-house clinical dataset to demonstrate the effectiveness of the proposed approach. The proposed approach significantly outperforms state-of-the-art methods, with improvements of 44.37% in Root Mean Square Error (RMSE), 19.93% in Structural Similarity Index Measure (SSIM), 20.01% in Peak Signal-to-Noise Ratio (PSNR), and 16.21% in Cross Correlation (CC) quality assessment metrics.
更多查看译文
关键词
Medical image registration,KPCA,TLBO,PCA,Feature extraction,Rigid transformation
AI 理解论文
溯源树
样例
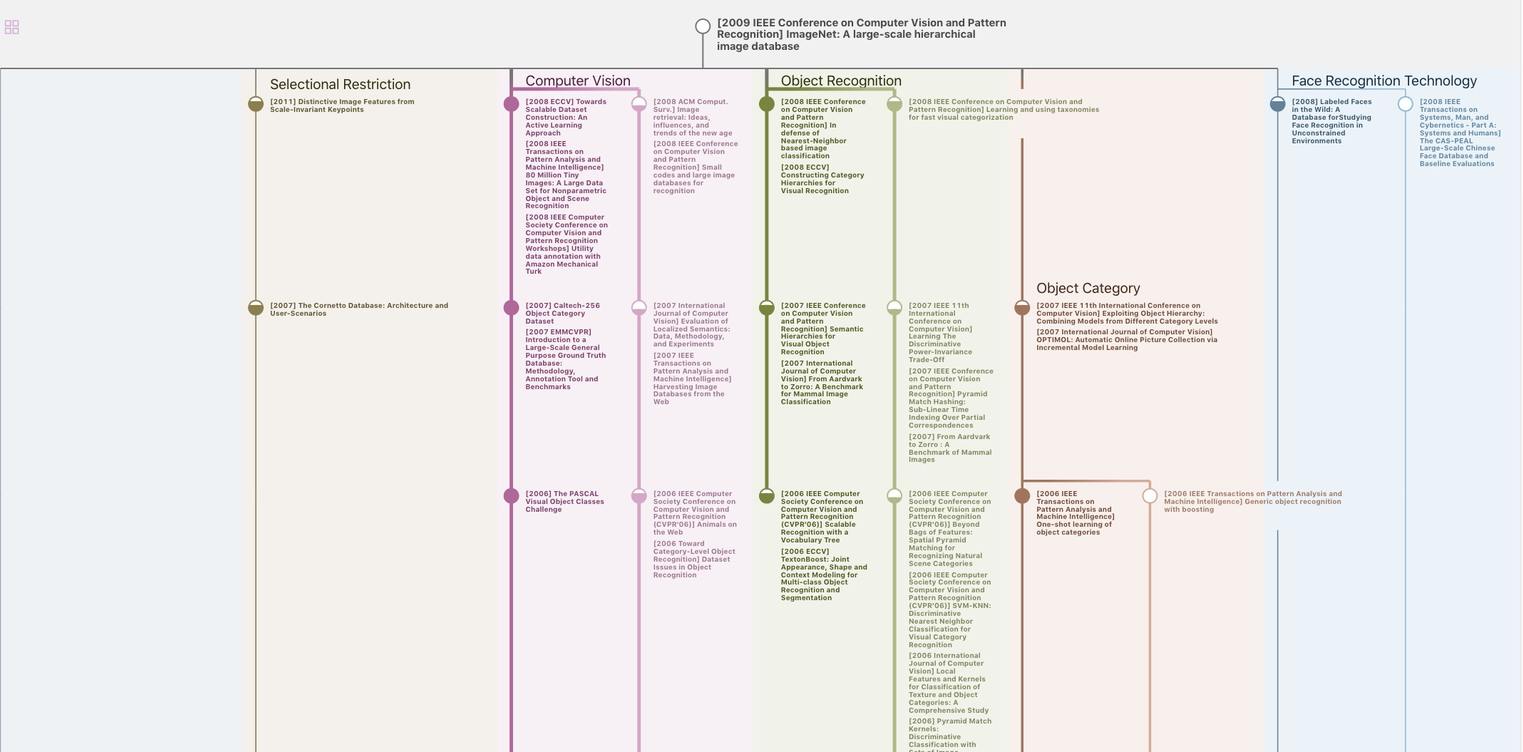
生成溯源树,研究论文发展脉络
Chat Paper
正在生成论文摘要