Machine Learning-Based Approach for Bandwidth and Frequency Prediction for N77 Band 5G Antenna
PHYSICA SCRIPTA(2024)
摘要
Yagi antennas are useful for wireless communications because of the directional gain they provide, allowing the antenna to concentrate the signal in either the transmission or reception direction. It is built on a substrate made of FR-4, this antenna has a return loss of -46.85 dB at 3.6 GHz and a bandwidth of 3.3-4.2 GHz within a -10 dB range, making it ideal for use in the n77 bands. Not only is it small, with a size of 0.642 lambda 0 x 0.583 lambda 0, but it also has a maximum gain of 7.95 dB and a maximum directivity of 8.58 dB. This study investigates several approaches to estimating the performance of an antenna. These approaches include simulation with a variety of software tools, including as CST, HFSS, and Altair Feko; curve fitting technology; and the RLC equivalent circuit model. After that, simulation with CST MWS is used to collect a large amount of data samples, and then supervised regression machine learning (ML) methods are used to determine the resonance frequency and bandwidth of the antenna. When it comes to predicting bandwidth and frequency, Random Forest Regression demonstrates an exceptional level of performance, particularly when comparing with the results produced by curve-fitting tools, neural networks, and regression machine learning models. When all of these considerations are taken into account, it is clear that the antenna is an outstanding option for the n77 band of a 5G communication system.
更多查看译文
关键词
Yagi-Uda,CST,MATLAB,curve fitting,machine learning,neural network,ADS
AI 理解论文
溯源树
样例
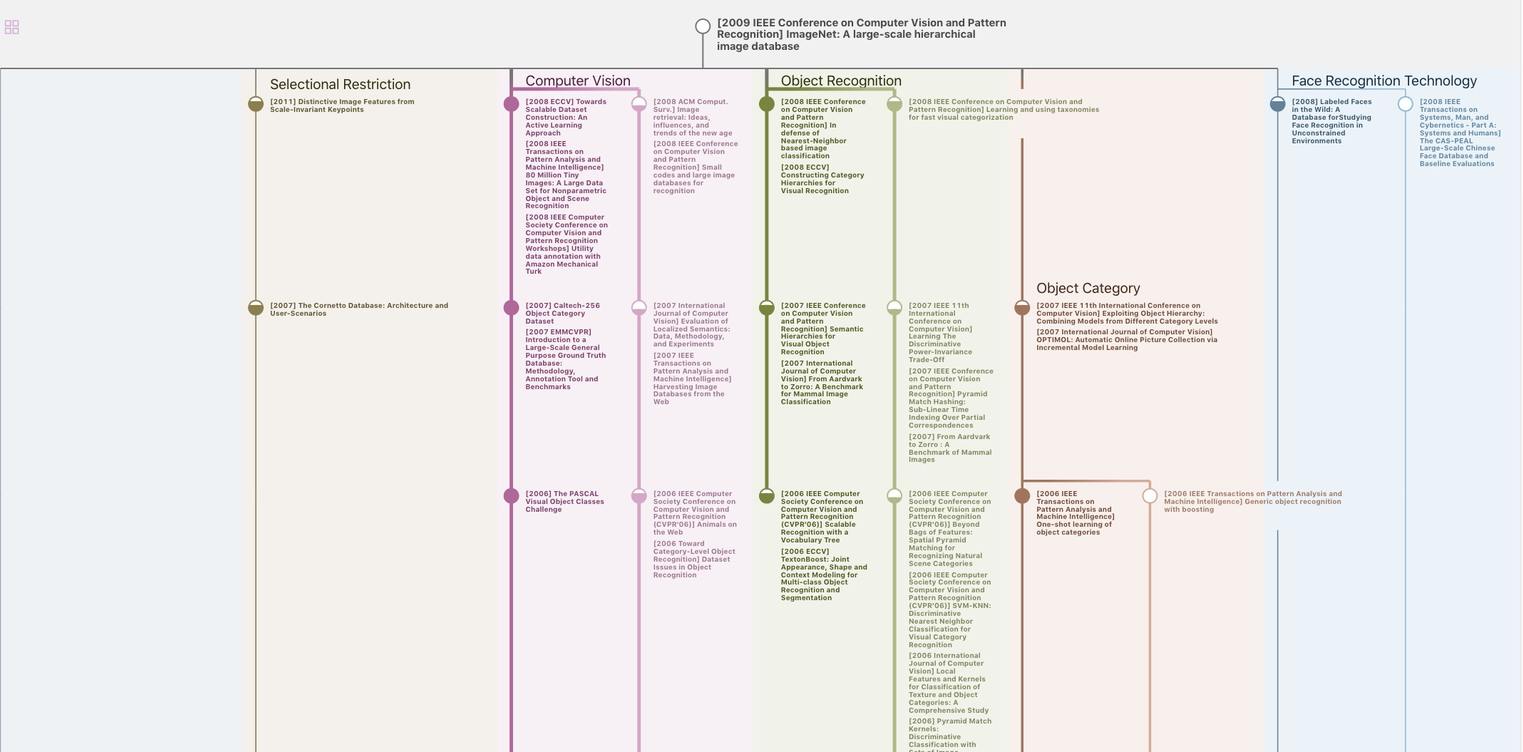
生成溯源树,研究论文发展脉络
Chat Paper
正在生成论文摘要