Towards a Greener Electrosynthesis: Pairing Machine Learning and 3D Printing for Rapid Optimisation of Anodic Trifluoromethylation
RSC sustainability(2024)
摘要
Applying electro-organic synthesis in flow configuration can potentially reduce the pharmaceutical industry's carbon footprint and simplify the reaction scale-up. However, the optimisation of such reactions has remained challenging due to the convoluted interplay between various input experimental parameters. Herein, we demonstrate the advantage of integrating a machine learning (ML) algorithm within an automated flow microreactor setup to assist in the optimisation of anodic trifluoromethylation without transition metal catalysts. The ML algorithm is able to optimise six reaction parameters concurrently and increase the reaction yield of anodic trifluoromethylation by >270% within two iterations. Furthermore, we discovered that suppression of electrode fouling and even higher reaction yields could be achieved by integrating 3D-printed metal electrodes into the microreactor. By coupling multiple analytical tools such as AC voltammetry, kinetic modelling, and gas chromatography, we gained holistic insights into the trifluoromethylation reaction mechanism, including potential sources of faradaic efficiency and reactant losses. More importantly, multiple electrochemical and non-electrochemical steps involved in this process are elucidated. Our findings highlight the potential of synergistically combining ML-assisted flow systems with advanced analytical tools to rapidly optimise complex electrosynthetic reactions sustainably.
更多查看译文
AI 理解论文
溯源树
样例
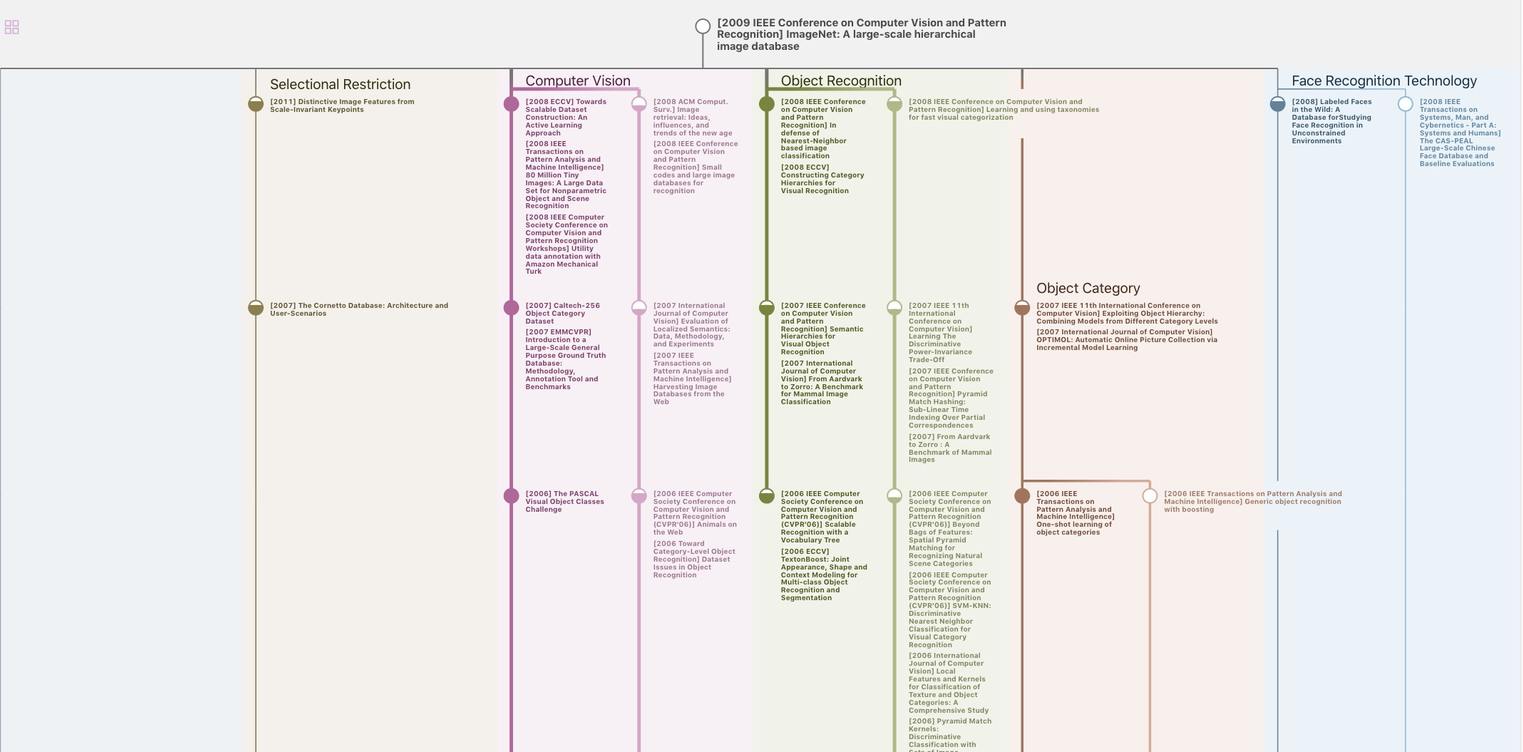
生成溯源树,研究论文发展脉络
Chat Paper
正在生成论文摘要