An ARCAD Database Nomogram for Prognostic Prediction of Treatment Refractory Metastatic Colorectal Cancer.
JOURNAL OF CLINICAL ONCOLOGY(2024)
关键词
Survival Analysis,Cancer Imaging,Predictive Modeling
AI 理解论文
溯源树
样例
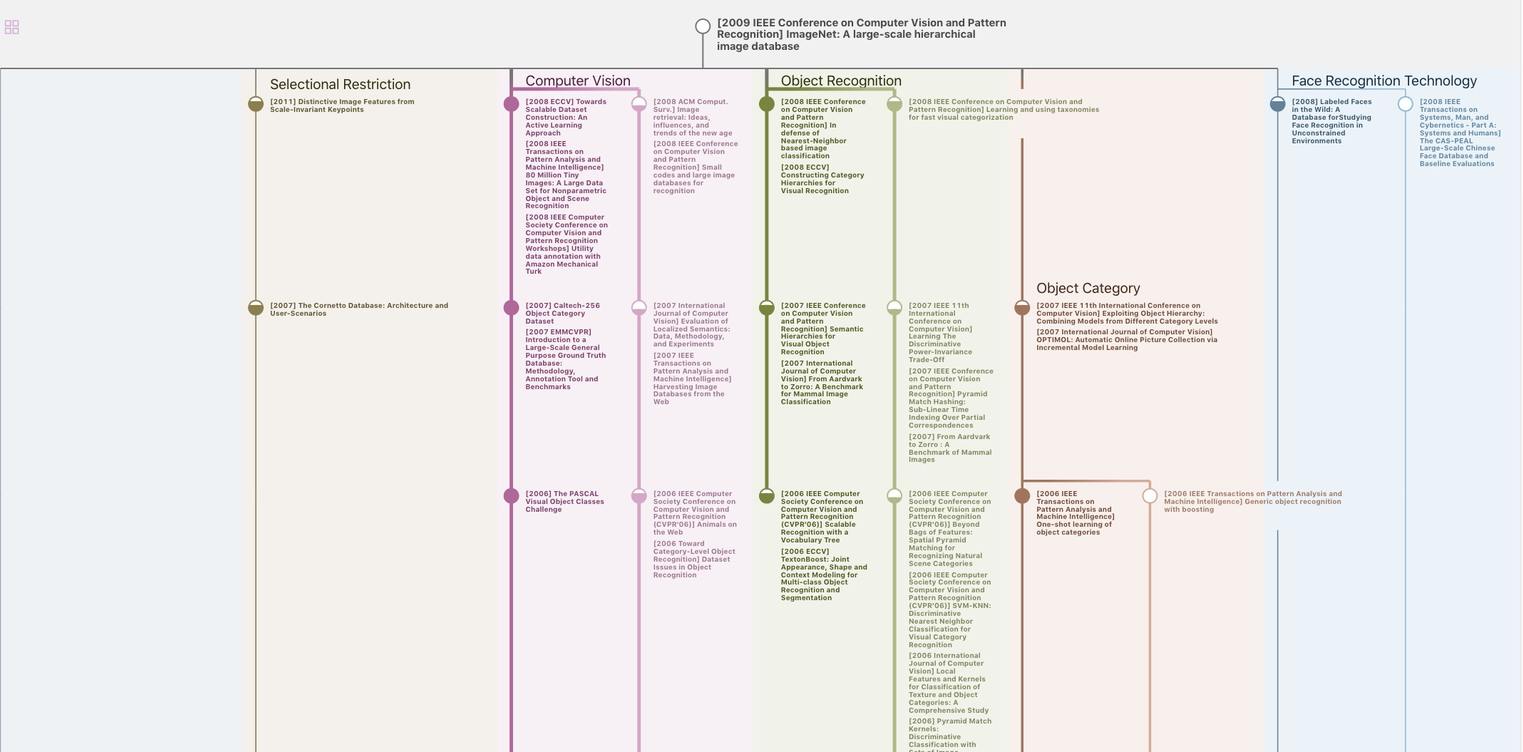
生成溯源树,研究论文发展脉络
Chat Paper
正在生成论文摘要
JOURNAL OF CLINICAL ONCOLOGY(2024)