Sugarcane Yield Estimation in Thailand at Multiple Scales Using the Integration of UAV and Sentinel-2 Imagery
PRECISION AGRICULTURE(2024)
关键词
Yield estimation,Sugarcane,UAV,Sentinel-2,Earth observation,Remote sensing,Upscaling,Data augmentation
AI 理解论文
溯源树
样例
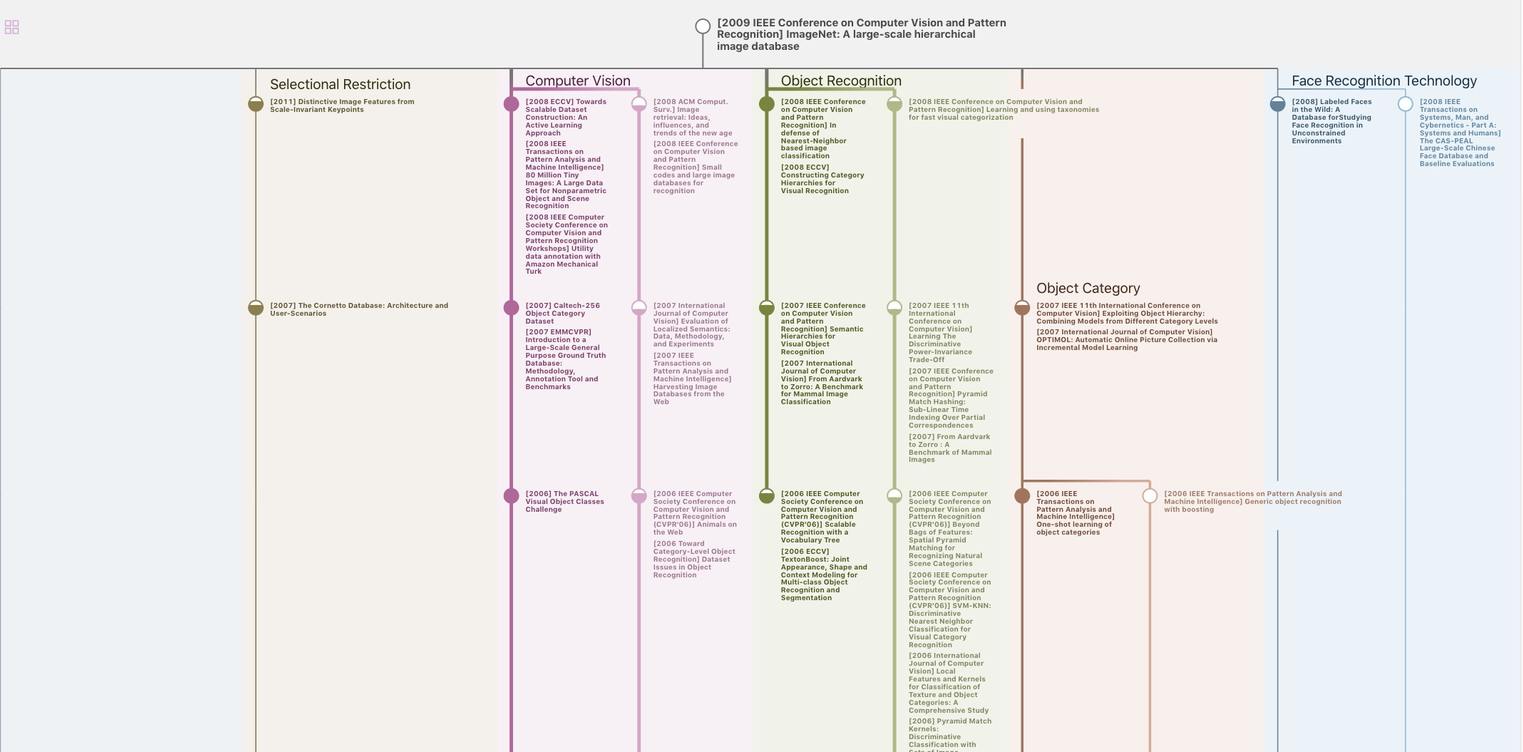
生成溯源树,研究论文发展脉络
Chat Paper
正在生成论文摘要