Predicting Cognitive Decline in Short‐term As a Viable Method to Improve Recruitment in Clinical Trials of Alzheimer’s Disease
Alzheimer's & dementia(2023)
摘要
Background Success of Alzheimer’s disease (AD) trials is partially dependent on enrollment of participants who show cognitive decline (CD) during treatment with placebo. We investigated whether trials could be enriched for CD using machine‐learning based predictive models. Method We used data from the placebo arm of trial of Solanezumab for mild dementia due to AD (EXPEDITION‐3 trial; N = 894) as well as individuals at the same stage of disease from Alzheimer’s Disease Neuroimaging Initiative (ADNI; N = 86). Participants were classified as showing CD vs stable cognition (SC) based on their scores on the cognitive sub‐scale of the Alzheimer’s Disease Assessment Scale (ADAS‐Cog11; Range 0‐70). The CD group included individuals who showed a minimal clinically important difference (MCID of 3 or more points increase in their ADAS‐Cog11 scores from baseline to week 80) while the SC group had a change of less than 3 points. Participants were classified into CD and SC groups using different baseline feature‐sets including volumetric MRI and neuropsychological tests (Table 2). Models were trained using 70% of the EXPEDITION‐3 data and were validated using the remaining 30%. Models trained in EXPEDITION‐3 were then externally validated using the ADNI sample. Result Only 56% of EXPEDITION‐3 participants treated with placebo showed MCID at 80 weeks (Table 1). Performance of the different models is summarized in Table 2. Model 4 using the random forest algorithm, which included all features and data from 6‐month follow‐up, had the best overall performance in the internal validation sample (AUC: 0.75±0.03). Models 3 and 4 performed similarly when validated in the independent ADNI sample (AUCs: 0.70±0.05, 0.66±0.06, respectively). Both Models had positive predictive values approximately 15% higher than the base prevalence of cognitive decline in the ADNI subset (∼62%). The negative predictive value of Model 3 was 23% higher than the base prevalence of stable cognition in the participants, slightly higher than that of Model 4. Conclusion Enrichment of trials through predictive models may increase the chance of success. Using 6‐month follow‐up data improves accuracy of decliner identification and may provide a basis for early exclusion of a subset unlikely to discriminate active treatment from placebo.
更多查看译文
AI 理解论文
溯源树
样例
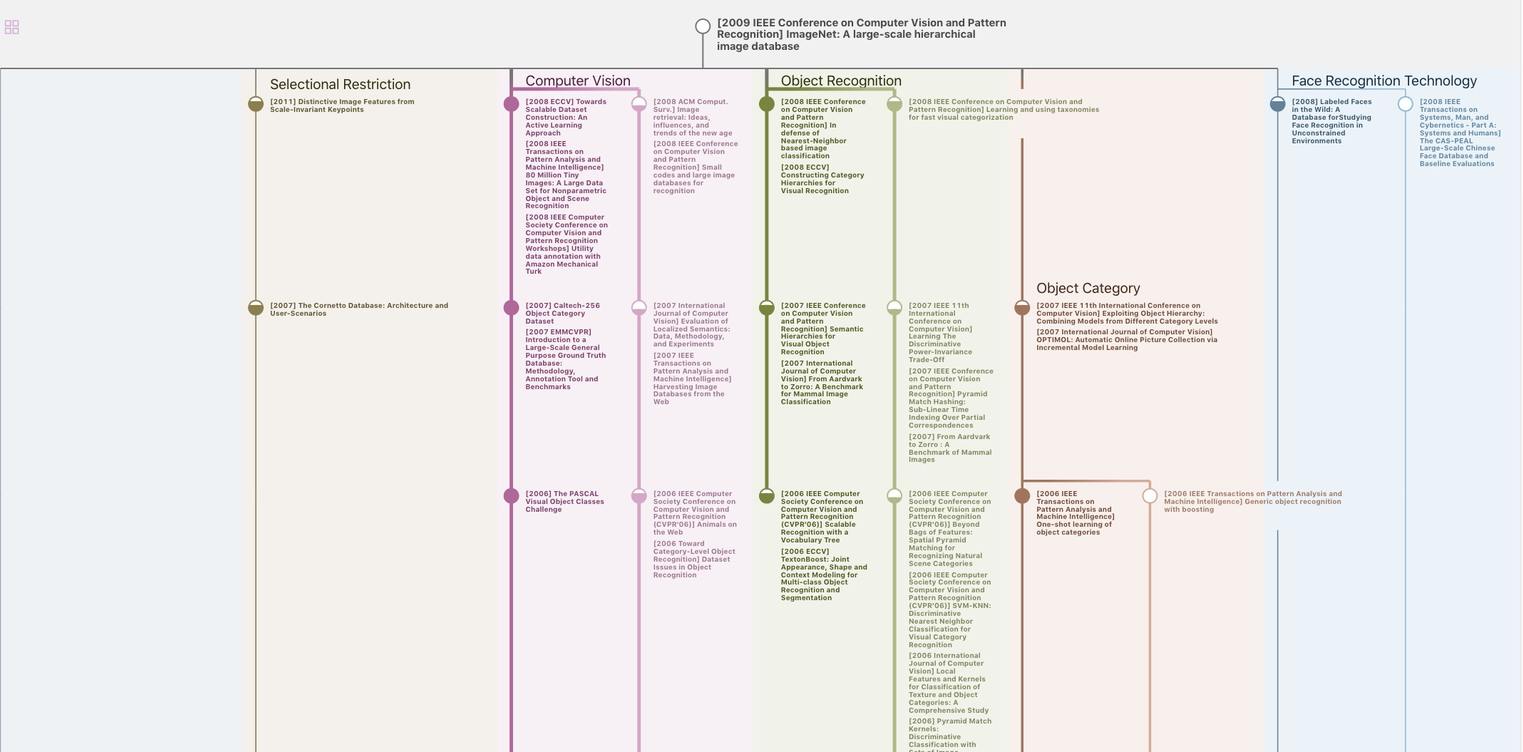
生成溯源树,研究论文发展脉络
Chat Paper
正在生成论文摘要