Whose LLM is It Anyway? Linguistic Comparison and LLM Attribution for GPT-3.5, GPT-4 and Bard
arXiv (Cornell University)(2024)
Bar Ilan University Department of Information Science | University College London Department of Cancer Biology
Abstract
Large Language Models (LLMs) are capable of generating text that is similarto or surpasses human quality. However, it is unclear whether LLMs tend toexhibit distinctive linguistic styles akin to how human authors do. Through acomprehensive linguistic analysis, we compare the vocabulary, Part-Of-Speech(POS) distribution, dependency distribution, and sentiment of texts generatedby three of the most popular LLMS today (GPT-3.5, GPT-4, and Bard) to diverseinputs. The results point to significant linguistic variations which, in turn,enable us to attribute a given text to its LLM origin with a favorable 88%accuracy using a simple off-the-shelf classification model. Theoretical andpractical implications of this intriguing finding are discussed.
MoreTranslated text
Key words
Language Modeling,Semantic Similarity,Topic Modeling,Part-of-Speech Tagging,Machine Translation
PDF
View via Publisher
AI Read Science
AI Summary
AI Summary is the key point extracted automatically understanding the full text of the paper, including the background, methods, results, conclusions, icons and other key content, so that you can get the outline of the paper at a glance.
Example
Background
Key content
Introduction
Methods
Results
Related work
Fund
Key content
- Pretraining has recently greatly promoted the development of natural language processing (NLP)
- We show that M6 outperforms the baselines in multimodal downstream tasks, and the large M6 with 10 parameters can reach a better performance
- We propose a method called M6 that is able to process information of multiple modalities and perform both single-modal and cross-modal understanding and generation
- The model is scaled to large model with 10 billion parameters with sophisticated deployment, and the 10 -parameter M6-large is the largest pretrained model in Chinese
- Experimental results show that our proposed M6 outperforms the baseline in a number of downstream tasks concerning both single modality and multiple modalities We will continue the pretraining of extremely large models by increasing data to explore the limit of its performance
Try using models to generate summary,it takes about 60s
Must-Reading Tree
Example
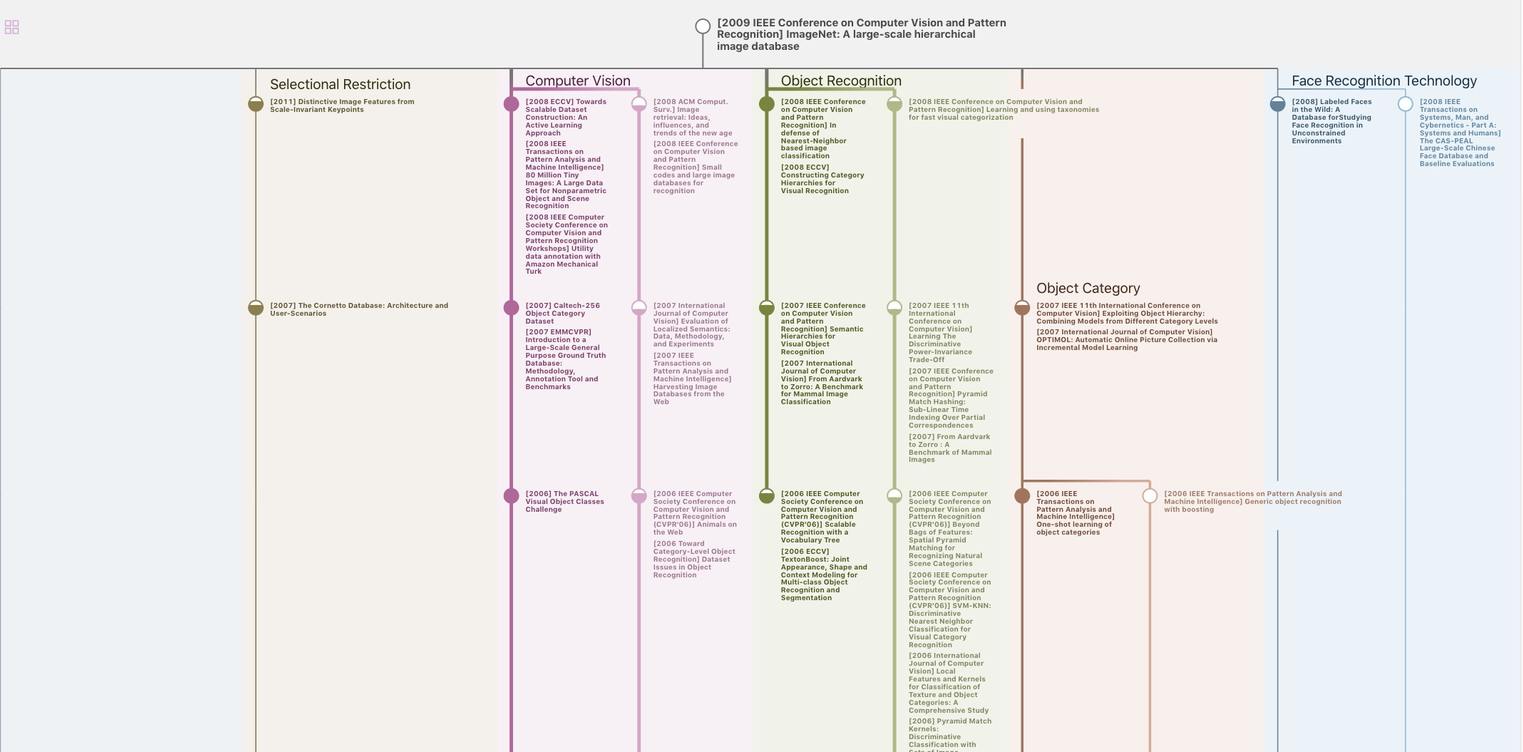
Generate MRT to find the research sequence of this paper
Related Papers
Voice in academic writing: The rhetorical construction of author identity in blind manuscript review
2007
被引用207 | 浏览
2003
被引用23 | 浏览
2001
被引用38 | 浏览
2024
被引用29 | 浏览
2023
被引用106 | 浏览
2024
被引用78 | 浏览
2023
被引用32 | 浏览
2024
被引用57 | 浏览
2023
被引用17 | 浏览
Data Disclaimer
The page data are from open Internet sources, cooperative publishers and automatic analysis results through AI technology. We do not make any commitments and guarantees for the validity, accuracy, correctness, reliability, completeness and timeliness of the page data. If you have any questions, please contact us by email: report@aminer.cn
Chat Paper
去 AI 文献库 对话