Advancing TTP Analysis: Harnessing the Power of Encoder-Only and Decoder-Only Language Models with Retrieval Augmented Generation
arXiv (Cornell University)(2024)
摘要
Tactics, Techniques, and Procedures (TTPs) outline the methods attackers use to exploit vulnerabilities. The interpretation of TTPs in the MITRE ATT&CK framework can be challenging for cybersecurity practitioners due to presumed expertise, complex dependencies, and inherent ambiguity. Meanwhile, advancements with Large Language Models (LLMs) have led to recent surge in studies exploring its uses in cybersecurity operations. This leads us to question how well encoder-only (e.g., RoBERTa) and decoder-only (e.g., GPT-3.5) LLMs can comprehend and summarize TTPs to inform analysts of the intended purposes (i.e., tactics) of a cyberattack procedure. The state-of-the-art LLMs have shown to be prone to hallucination by providing inaccurate information, which is problematic in critical domains like cybersecurity. Therefore, we propose the use of Retrieval Augmented Generation (RAG) techniques to extract relevant contexts for each cyberattack procedure for decoder-only LLMs (without fine-tuning). We further contrast such approach against supervised fine-tuning (SFT) of encoder-only LLMs. Our results reveal that both the direct-use of decoder-only LLMs (i.e., its pre-trained knowledge) and the SFT of encoder-only LLMs offer inaccurate interpretation of cyberattack procedures. Significant improvements are shown when RAG is used for decoder-only LLMs, particularly when directly relevant context is found. This study further sheds insights on the limitations and capabilities of using RAG for LLMs in interpreting TTPs.
更多查看译文
关键词
Attack Graphs,Source Code Analysis,Topic Modeling
AI 理解论文
溯源树
样例
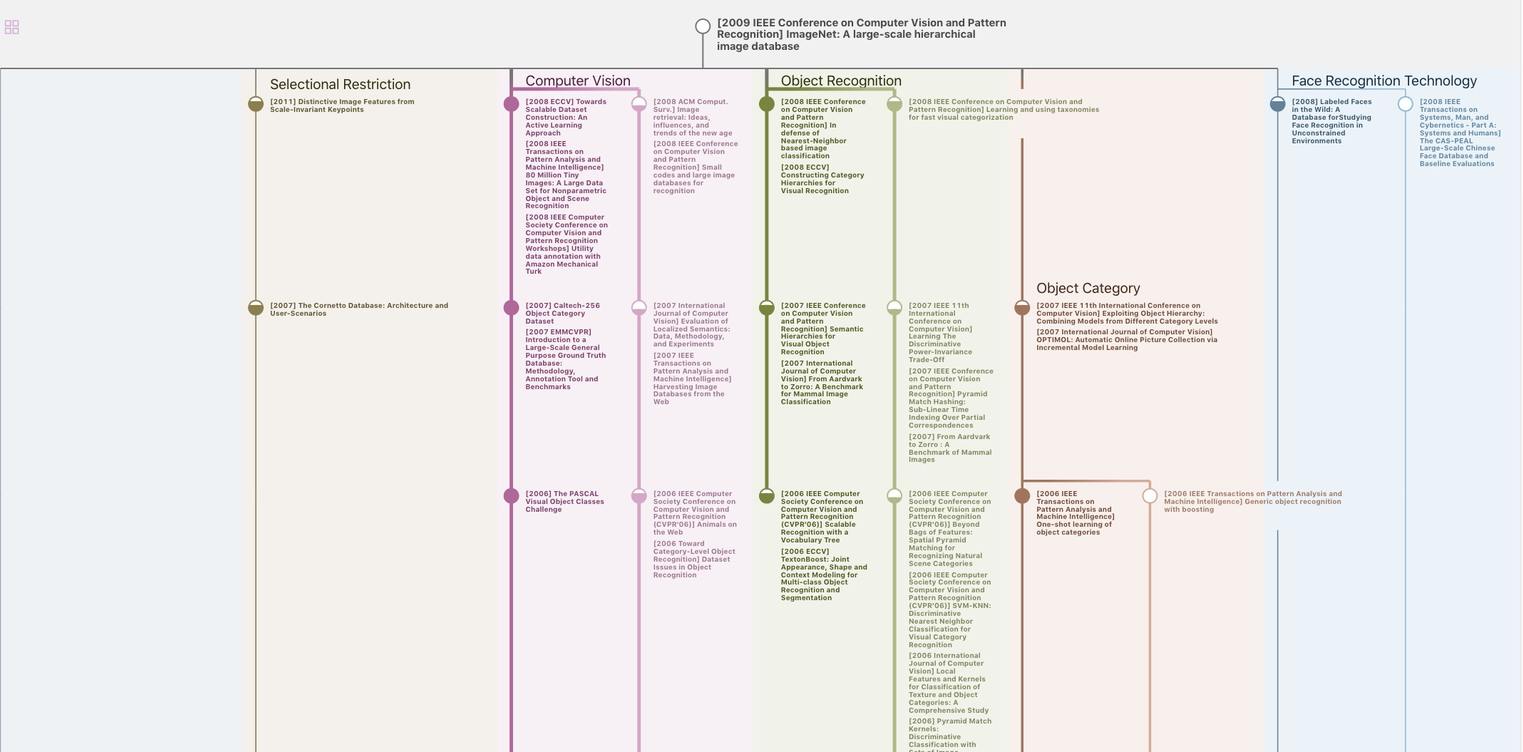
生成溯源树,研究论文发展脉络
Chat Paper
正在生成论文摘要