GRU-CP: Non-stationary Time Series Forecasting with Change Point Detection.
2023 International Conference on Cyber-Enabled Distributed Computing and Knowledge Discovery (CyberC)(2023)
Abstract
Time series forecasting finds numerous applications in real-world scenarios such as finance, healthcare, and weather prediction. However, a significant portion of real-world time series data exhibits non-stationarity. While some methods like Prophet and STL can decompose non-stationary time series due to seasonality and periodicity for accurate predictions, they may falter when faced with unforeseeable factors, such as structural breaks induced by government interventions in exchange rates. This study asserts that non-stationary time series can be segmented into multiple stationary segments via change points, and these segments may possess latent temporal characteristics. By employing change point detection algorithms to identify non-stationary features, these features can be incorporated into prediction models. The proposed GRU-CP model is introduced in this context. Compared to traditional models, GRU-CP yields lower errors when forecasting time series data.
MoreTranslated text
Key words
Time Series Forecasting,Change Point Detection,Non-stationarity,Gated Recurrent Unit
AI Read Science
Must-Reading Tree
Example
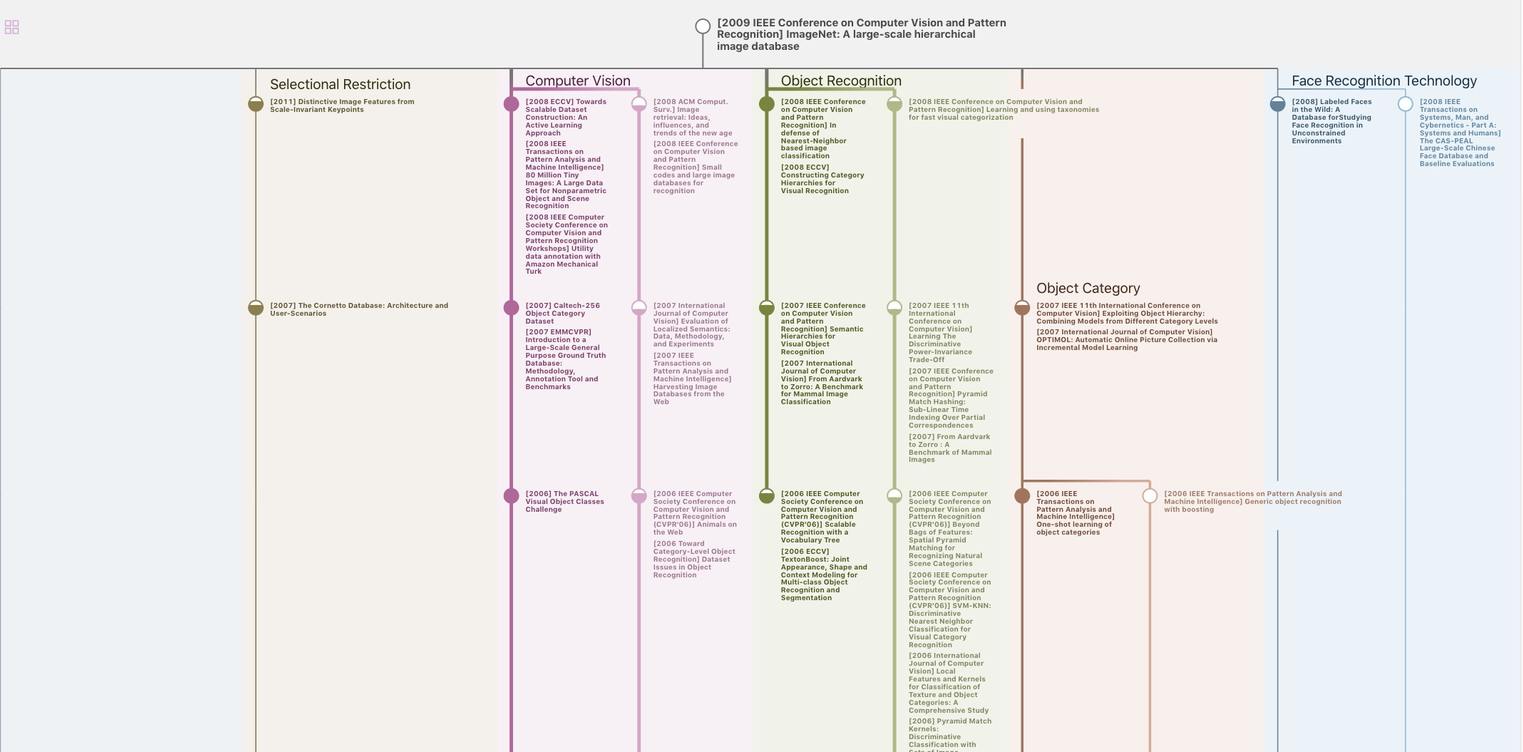
Generate MRT to find the research sequence of this paper
Chat Paper
Summary is being generated by the instructions you defined