Improved Coronary Artery Calcium (CAC) Detection in Conventional CT with Deep-Learning Image De-Blurring
MEDICAL IMAGING 2024 PHYSICS OF MEDICAL IMAGING, PT 1(2024)
摘要
We investigated the impact of a CNN-based deep-learning (DL) image de-blurring algorithm on coronary artery calcium (CAC) detection performance in conventional CT imaging. Our approach comprises first de-noising the image with a state-of-the-art CNN-based image de-noising algorithm. With improved SNR, it is then possible to sharpen the image with a CNN-based image de-blurring algorithm. We train such networks using natural images, i.e., a large set of diverse photographs. The de-noising strength in the final image can be adjusted by blending back the estimated noise from the first step to the desired degree. To assess the impact of the de-blurring algorithm, we scanned an anthropomorphic phantom containing 100 small calcifications on a CT system using a CAC scoring protocol. Data were acquired at clinical and high dose, and subsequently reconstructed with and without the DL de-blurring algorithm, using 25% of the maximum de-noising strength. For each small CAC, detectability was defined as the ability to calculate an Agatston score (at least 3 adjacent voxels exceeding 130 HU). For the high dose scans, CAC detectability increased from 39% for the standard reconstruction to 49% with de-blurring. The same 39% CAC detectability at high dose without de-blurring was obtained with routine dose with de-blurring. In this work, we also show some visual impressions of applying our DL de-blurring method to clinical cardiac data.
更多查看译文
关键词
Cardiac Imaging,Coronary Calcium,Dual-Energy CT,Computed Tomography Angiography,Multi-Energy CT
AI 理解论文
溯源树
样例
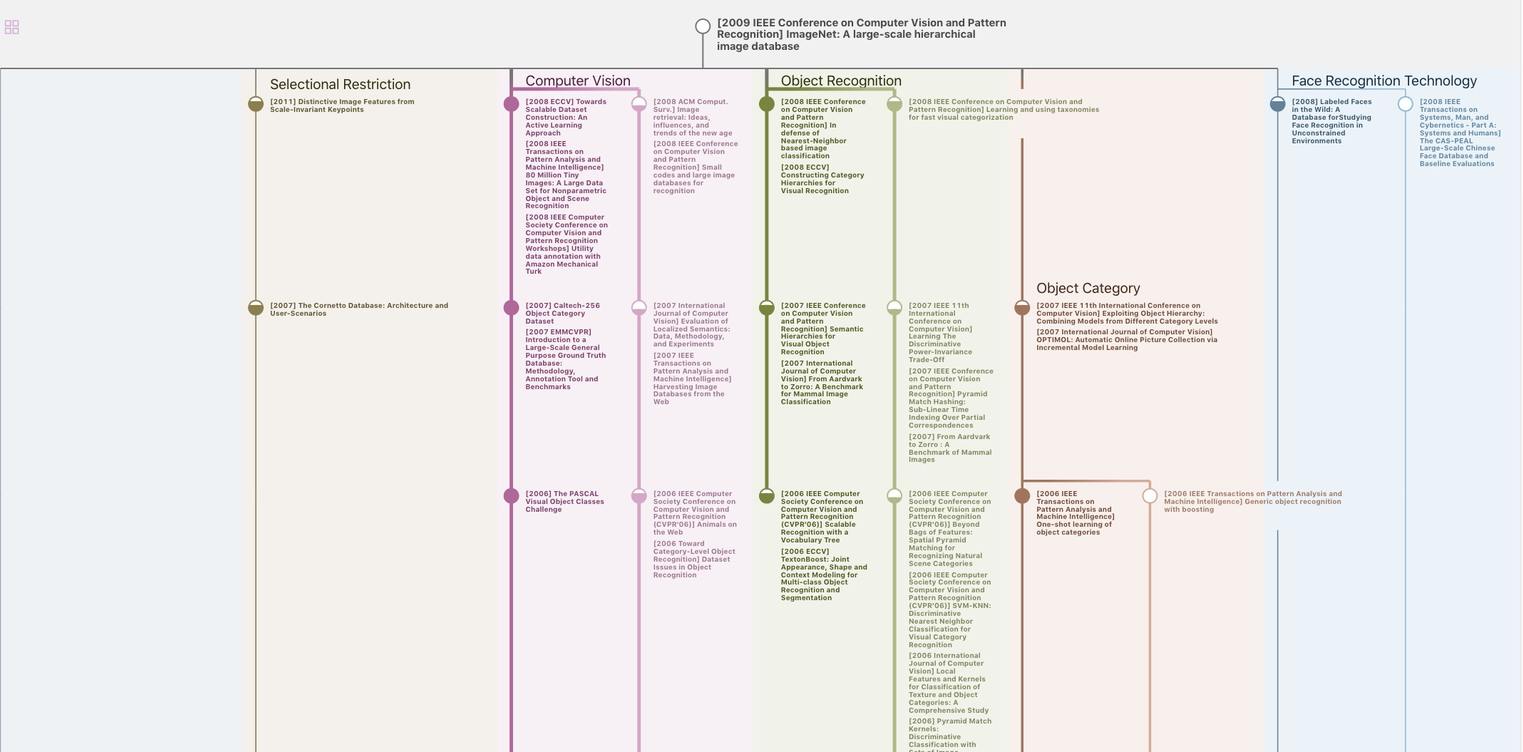
生成溯源树,研究论文发展脉络
Chat Paper
正在生成论文摘要