Exact recovery in Gaussian weighted stochastic block model and planted dense subgraphs: Statistical and algorithmic thresholds
arxiv(2024)
摘要
In this paper, we study the exact recovery problem in the Gaussian weighted
version of the Stochastic block model with two symmetric communities. We
provide the information-theoretic threshold in terms of the signal-to-noise
ratio (SNR) of the model and prove that when SNR <1, no statistical estimator
can exactly recover the community structure with probability bounded away from
zero. On the other hand, we show that when SNR >1, the Maximum likelihood
estimator itself succeeds in exactly recovering the community structure with
probability approaching one. Then, we provide two algorithms for achieving
exact recovery. The Semi-definite relaxation as well as the spectral relaxation
of the Maximum likelihood estimator can recover the community structure down to
the threshold value of 1, establishing the absence of an
information-computation gap for this model.
Next, we compare the problem of community detection with the problem of
recovering a planted densely weighted community within a graph and prove that
the exact recovery of two symmetric communities is a strictly easier problem
than recovering a planted dense subgraph of size half the total number of
nodes, by establishing that when the same SNR< 3/2, no statistical estimator
can exactly recover the planted community with probability bounded away from
zero. More precisely, when 1 < SNR < 3/2 exact recovery of community
detection is possible, both statistically and algorithmically, but it is
impossible to exactly recover the planted community, even statistically, in the
Gaussian weighted model. Finally, we show that when SNR >2, the Maximum
likelihood estimator itself succeeds in exactly recovering the planted
community with probability approaching one. We also prove that the
Semi-definite relaxation of the Maximum likelihood estimator can recover the
planted community structure down to the threshold value of 2.
更多查看译文
AI 理解论文
溯源树
样例
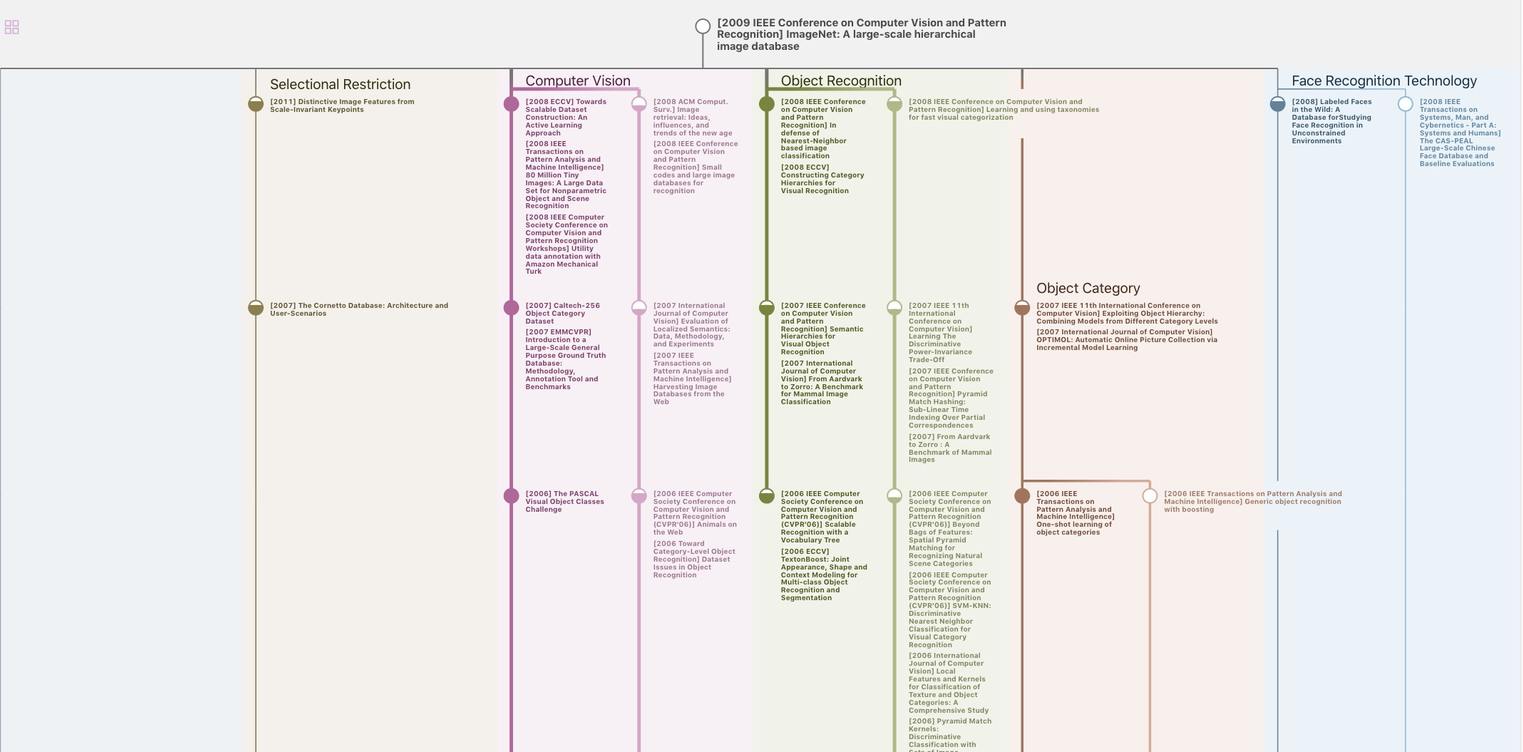
生成溯源树,研究论文发展脉络
Chat Paper
正在生成论文摘要