Infrared Spectroscopy Based Cordyceps Authenticity Detection and Multi-Classification Tasks by Privacy-Preserving Federated Learning
Microchemical journal(2024)
Abstract
The modeling and analysis of infrared spectrum data have gained significant advantages in the field of rapid detection of herbal medicine. However, extracting valuable insights and patterns from diverse chemical information relies on large-scale datasets and advanced algorithms. Unfortunately, due to privacy concerns, data sharing between different companies is often limited in practice. In such scenarios, federated learning emerges as a novel solution. Federated learning allows different regions or companies to jointly train models without sharing sensitive data, thereby protecting privacy. To address the quality control challenges related to Cordyceps sinensis(C.sinensis), this paper proposes a federated learning and deep learning method based on infrared spectroscopy data of C.sinensis, and then evaluates their performance on the C.sinensis authenticity detection and multi-classification tasks. In the classification tasks of ’part’, ’phase’, ’region’, and ’True or False’ (TorF), the mean MCC of the best model is 0.8805, 0.5865, 0.7004, and 0.9555 respectively. Experimental results show that the method proposed in this paper is an effective C.sinensis quality control method and it also provides solutions and ideas for privacy protection issues involving drug monitoring.
MoreTranslated text
Key words
FTIR,Cordyceps sinensis,Federated learning,Deep learning
AI Read Science
Must-Reading Tree
Example
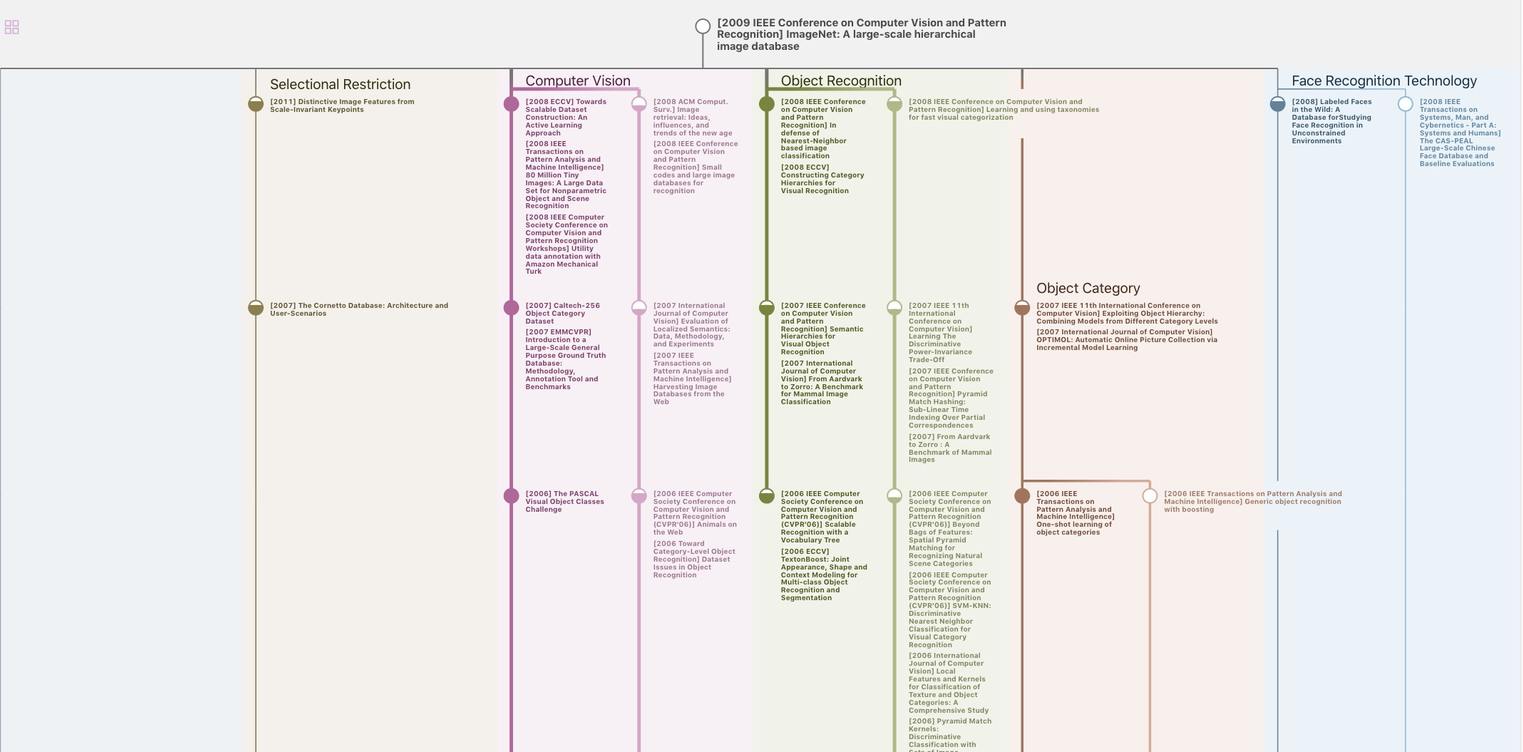
Generate MRT to find the research sequence of this paper
Chat Paper
Summary is being generated by the instructions you defined