Mapping Water Clarity in Small Oligotrophic Lakes Using Sentinel-2 Imagery and Machine Learning Methods: A Case Study of Canandaigua Lake in Finger Lakes, New York
IEEE JOURNAL OF SELECTED TOPICS IN APPLIED EARTH OBSERVATIONS AND REMOTE SENSING(2024)
摘要
Optical remote sensing of water quality poses challenges in small oligotrophic lakes due to the diminishing contribution of constituents to the water-leaving radiance as water clarity increases. For monitoring water clarity over such lakes, this study utilizes machine learning models and data from citizen science to develop effective models for retrieving Secchi disk depth (SDD) in Canandaigua Lake, USA. Using Sentinel-2 band ratios as input, we trained random forest (RF), adaptive boosting, extreme gradient boosting, and support vector regression models using spatiotemporally distributed in situ data within 7 days of Senitnel-2 overpass. Each model was optimized using hyperparameter tuning, and cross-validation was used for accuracy assessment to compare the models' effectiveness in retrieving SDD. The results indicate the superior performance of RF with an R (2) of similar to 0.74 and a root mean squared error of similar to 0.72 m. A feature importance analysis for RF indicated the high relevance of the blue and green bands ratio in the estimation of SDD. The RF model was subsequently employed to generate temporal maps for Canandaigua Lake, indicating that water clarity tends to be higher during the early summer months (May and June) but declines during late summer and fall (September and October). This pattern can be closely associated with the increased algal presence in the lake. The spatial variability of the SDD indicated the possibility of greater sediments entering from the southern part of the lake. This study can be expanded to encompass other Finger Lakes, offering a comprehensive understanding of water clarity in these lake systems.
更多查看译文
关键词
Lakes,Monitoring,Water resources,Water quality,Remote sensing,Biological system modeling,Spatial resolution,Freshwater,machine learning (ML),oligotrophic lakes,random forest (RF),remote sensing,Secchi disk depth (SDD),Sentinel-2,water quality
AI 理解论文
溯源树
样例
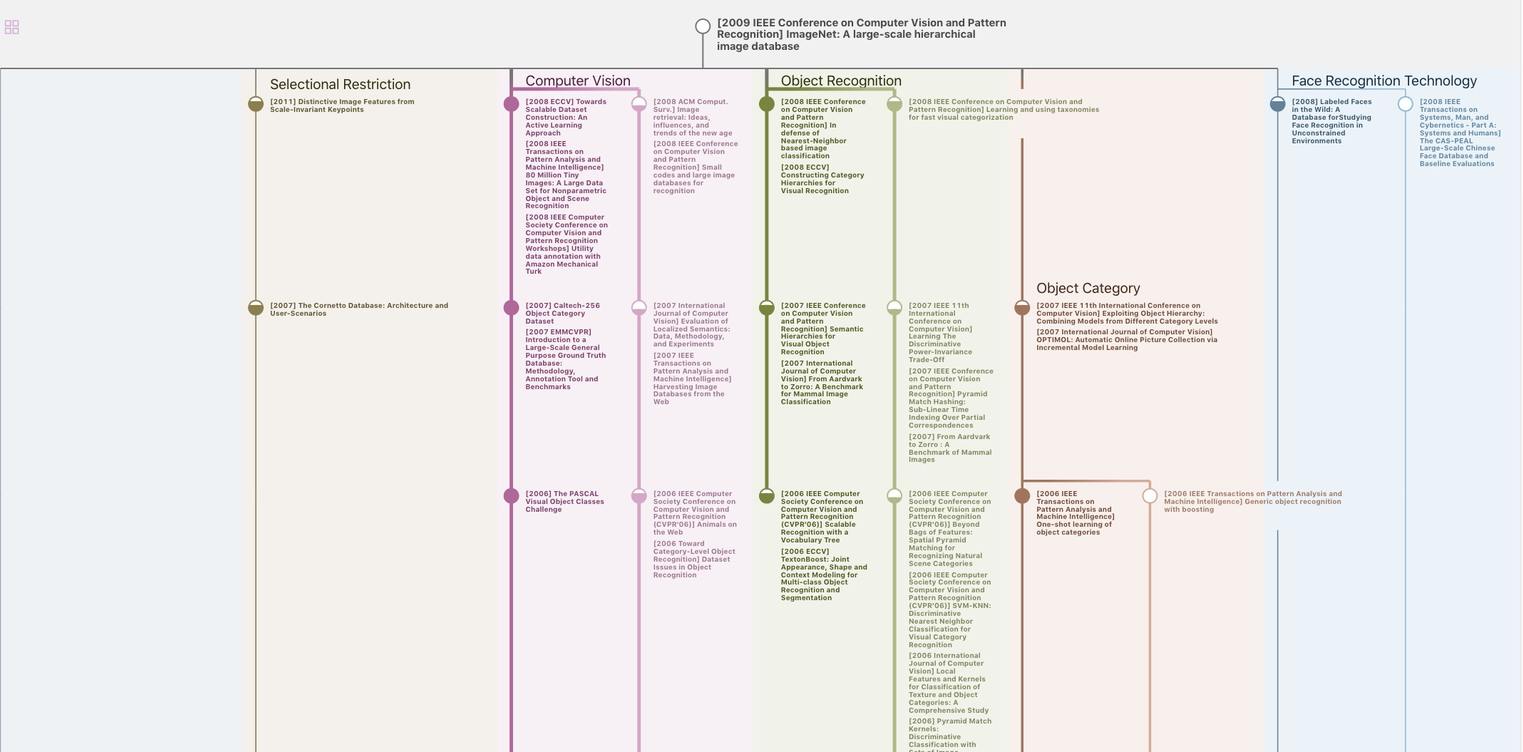
生成溯源树,研究论文发展脉络
Chat Paper
正在生成论文摘要