Personalized Modeling of Alzheimer's Disease Progression Estimates Neurodegeneration Severity from EEG Recordings.
ALZHEIMER'S & DEMENTIA DIAGNOSIS, ASSESSMENT & DISEASE MONITORING(2024)
关键词
Alzheimer's disease,Computational models,Computational neuroscience,EEG,Novel Biomarkers
AI 理解论文
溯源树
样例
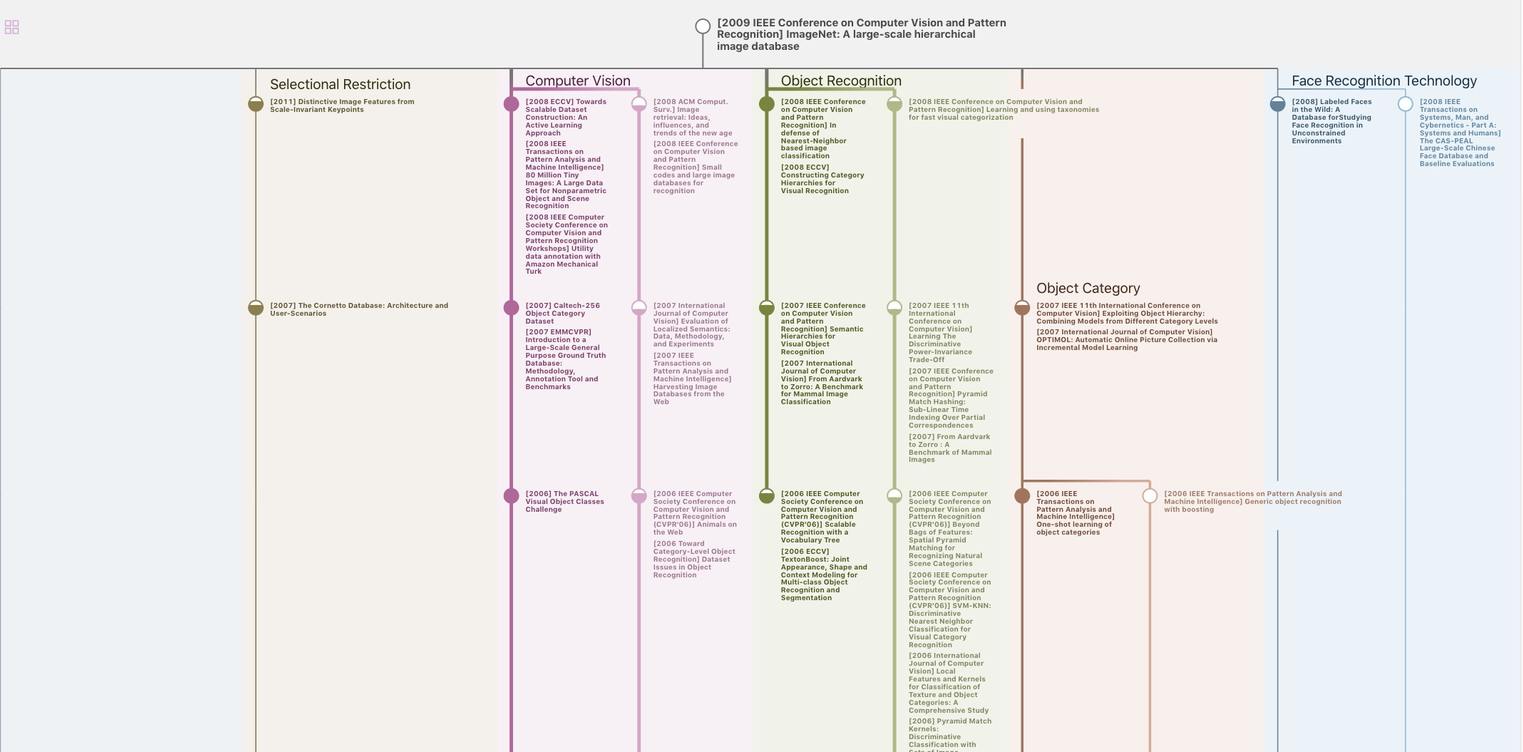
生成溯源树,研究论文发展脉络
Chat Paper
正在生成论文摘要