Online Content-Based Sequential Recommendation Considering Multimodal Contrastive Representation and Dynamic Preferences
Neural Computing and Applications(2024)
摘要
The online content, including live streaming and short videos, provides abundant visual and textual product information to users, which offers insights into users’ multiple and changeable preferences toward product style, brand, color, etc. These dynamic preferences are helpful to the product recommender system to extract users’ purchasing patterns. However, existing sequential recommendation fails to combine with items’ multimodal characteristics and dynamic preferences. This paper proposes the O nline C ontent- B ased S equence R ecommendation method (named OCBSR), where dynamic preferences and multimodal representation such as visual images, textual descriptions, and item-ID of products are leveraged with convolutional autoencoder to map multimodal data into a unified latent space. We devise a multimodal transformer mechanism to aggregate the multimodal representation. A novel contrastive infomax loss is constructed through contrastive prototype representations and noise-contrastive sequences. The loss enhances the correlation between single-modality and multimodality preferences and learns the contrastive representation. Then, users’ dynamic preferences are extracted from the contrastive representation in each time step using the gated recurrent unit with skip connections. Experiments are conducted on two real-life multimodal datasets, H&M and KuaiRec . The hit ratio (HR) and normalized discounted cumulative gain (NDCG) results indicate that our approach noticeably outperforms the current advanced recommenders. The HR increases by above 20% and 25% on H&M and KuaiRec datasets, respectively; the NDCG rises about 12% and 24% on H&M and KuaiRec datasets, respectively. The ablation study verifies each module’s effectiveness. This research contributes to online content literature and multimodal sequential recommendation in practice.
更多查看译文
关键词
Sequential recommendation,Multimodal learning,Dynamic preferences,Transformer mechanism,Contrastive learning,Online content and services
AI 理解论文
溯源树
样例
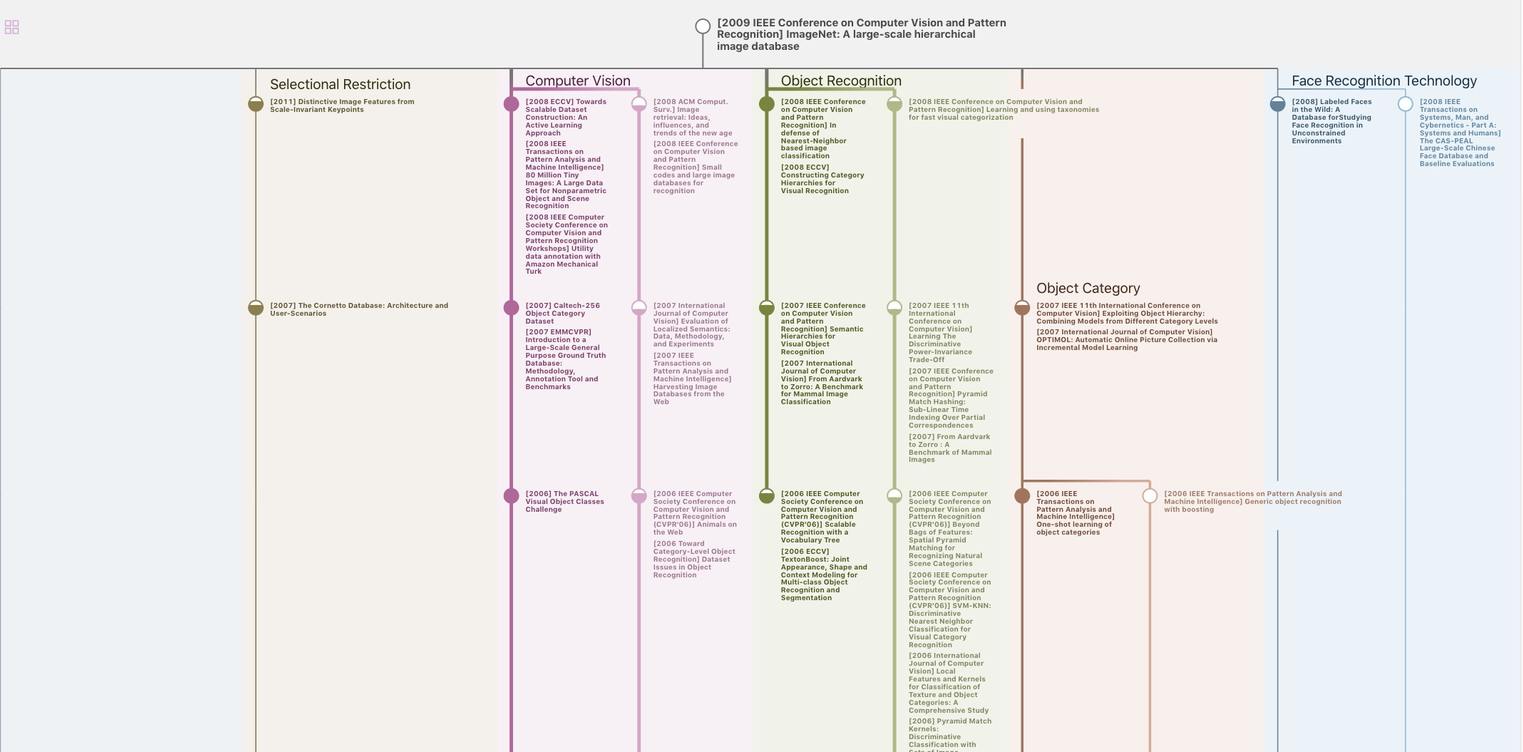
生成溯源树,研究论文发展脉络
Chat Paper
正在生成论文摘要