Studying the Impact of Quantum-Specific Hyperparameters on Hybrid Quantum-Classical Neural Networks
arxiv(2024)
摘要
In current noisy intermediate-scale quantum devices, hybrid quantum-classical
neural networks (HQNNs) represent a promising solution that combines the
strengths of classical machine learning with quantum computing capabilities.
Compared to classical deep neural networks (DNNs), HQNNs present an additional
set of hyperparameters, which are specific to quantum circuits. These
quantum-specific hyperparameters, such as quantum layers depth, number of
qubits, type of entanglement, type of encoding, number of shots, and
measurement observables, can be tuned to modify the behavior of the HQNNs and
their capabilities to learn the given task. In this paper, we investigate the
impact of these variations on different HQNN models for image classification
tasks, implemented on both Qiskit and PennyLane frameworks. We aim to uncover
intuitive and counter-intuitive learning patterns of HQNN models within
granular levels of controlled quantum perturbations, to form a sound basis for
their correlation to accuracy. The outcome of our study opens new avenues for
designing efficient HQNN algorithms and builds a foundational base for
comprehending and identifying tunable hyperparameters of HQNN models that can
lead to useful implementation and usage.
更多查看译文
AI 理解论文
溯源树
样例
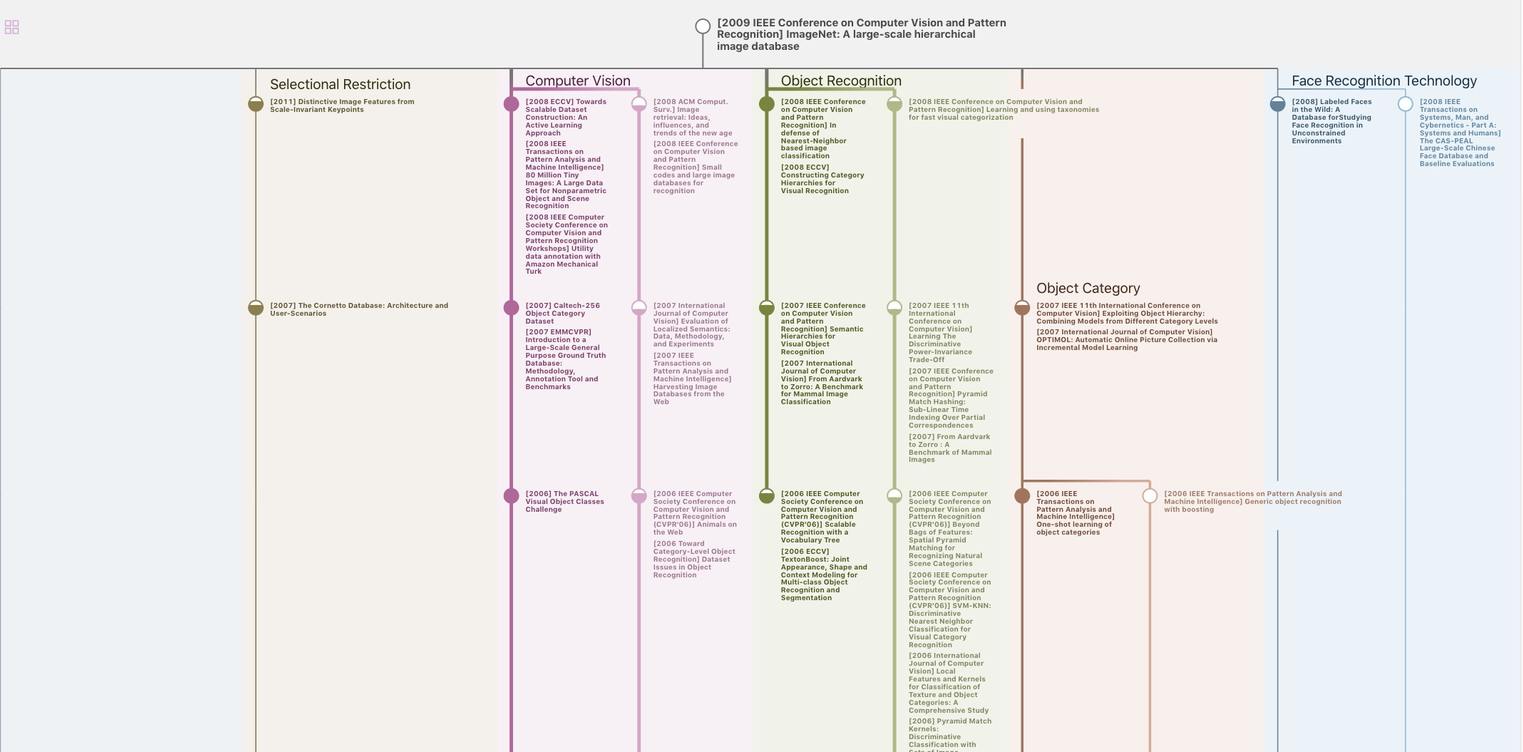
生成溯源树,研究论文发展脉络
Chat Paper
正在生成论文摘要