A Hybrid Approach for Many-Objective Feature Selection in Intrusion Detection on Windows Operating Systems
2023 IEEE CHILEAN Conference on Electrical, Electronics Engineering, Information and Communication Technologies (CHILECON)(2023)
Key words
Machine Learning,Cybersecurity,Feature Selection,Many-objective Optimization,Intrusion Detection
AI Read Science
Must-Reading Tree
Example
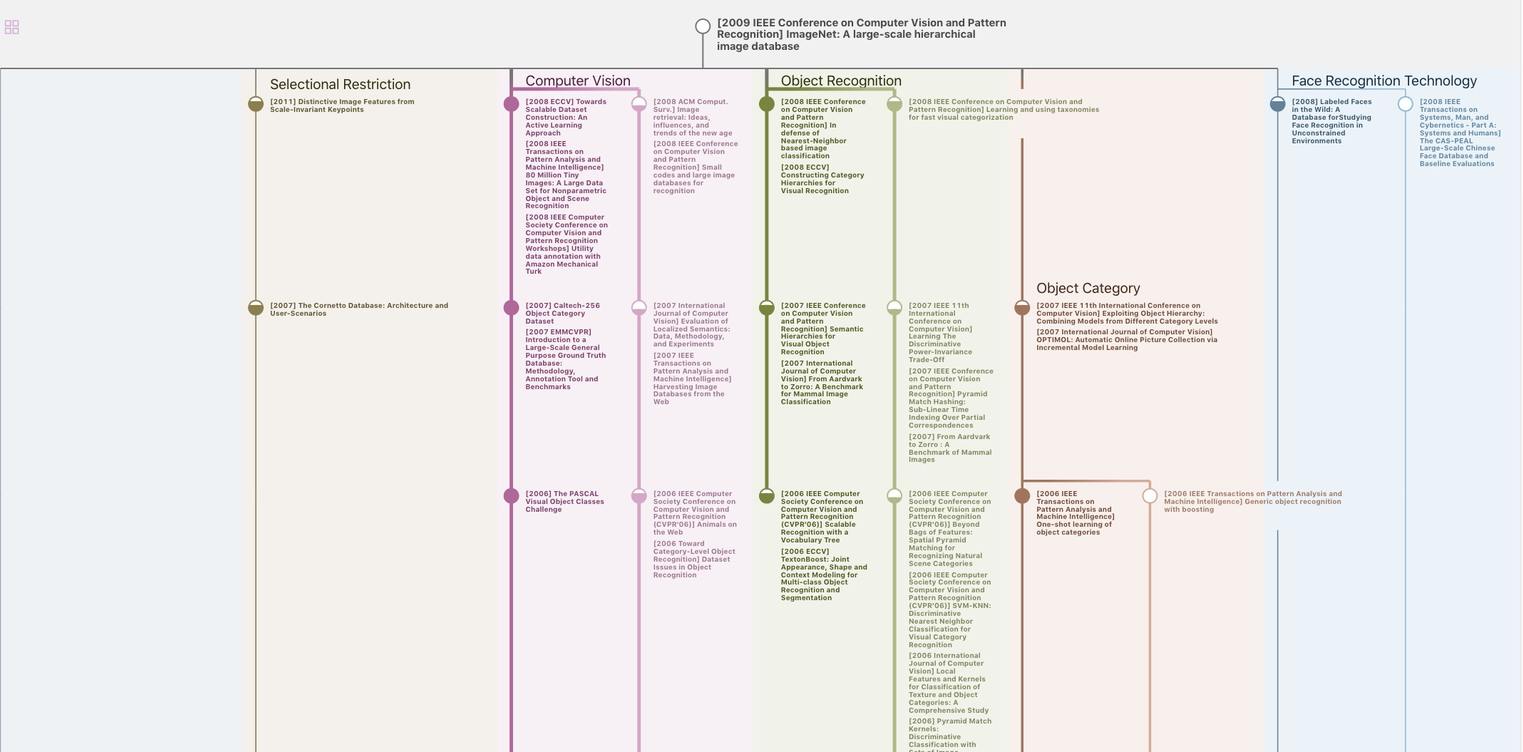
Generate MRT to find the research sequence of this paper
Chat Paper
Summary is being generated by the instructions you defined