Process-Informed Subsampling Improves Subseasonal Rainfall Forecasts in Central America
GEOPHYSICAL RESEARCH LETTERS(2024)
摘要
Subseasonal rainfall forecast skill is critical to support preparedness for hydrometeorological extremes. We assess how a process-informed evaluation, which subsamples forecasting model members based on their ability to represent potential predictors of rainfall, can improve monthly rainfall forecasts within Central America in the following month, using Costa Rica and Guatemala as test cases. We generate a constrained ensemble mean by subsampling 130 members from five dynamic forecasting models in the C3S multimodel ensemble based on their representation of both (a) zonal wind direction and (b) Pacific and Atlantic sea surface temperatures (SSTs), at the time of initialization. Our results show in multiple months and locations increased mean squared error skill by 0.4 and improved detection rates of rainfall extremes. This method is transferrable to other regions driven by slowly-changing processes. Process-informed subsampling is successful because it identifies members that fail to represent the entire rainfall distribution when wind/SST error increases. Subseasonal rainfall forecasts provide alerts multiple weeks ahead. These forecasts present an opportunity to facilitate anticipatory actions yet are often unreliable to use when preparing for extreme weather. We develop a method to optimize rainfall forecasts by selecting individual members from a large ensemble of dynamic forecasting model outputs based on their ability to represent potential predictors of rainfall. We test our method on monthly rainfall forecasts within Central America in the following month, using Costa Rica and Guatemala as key test cases. We select members from five contributing models of the C3S multimodel ensemble using regional predictors, including wind direction and sea surface temperatures (SSTs). Our results show improvements in the detection of low and high rainfall extremes. This method is transferrable to other regions driven by slowly-changing processes like SSTs and is beneficial for operational forecasters who can leverage regional expertise of relevant rainfall-generating processes to subsample better performing ensemble members for their regions. Subsampling members using sea surface temperatures and zonal wind improves subseasonal ensemble rainfall forecasts in Central AmericaIn multiple months and locations mean squared error skill increases by 0.4 and extreme rainfall skill improves by 0.5 (Heidke skill)Process-informed subsampling is useful because the models' representation of rainfall degrades as process error increases
更多查看译文
关键词
rainfall,forecast,Central America,subseasonal,extreme weather,ensemble
AI 理解论文
溯源树
样例
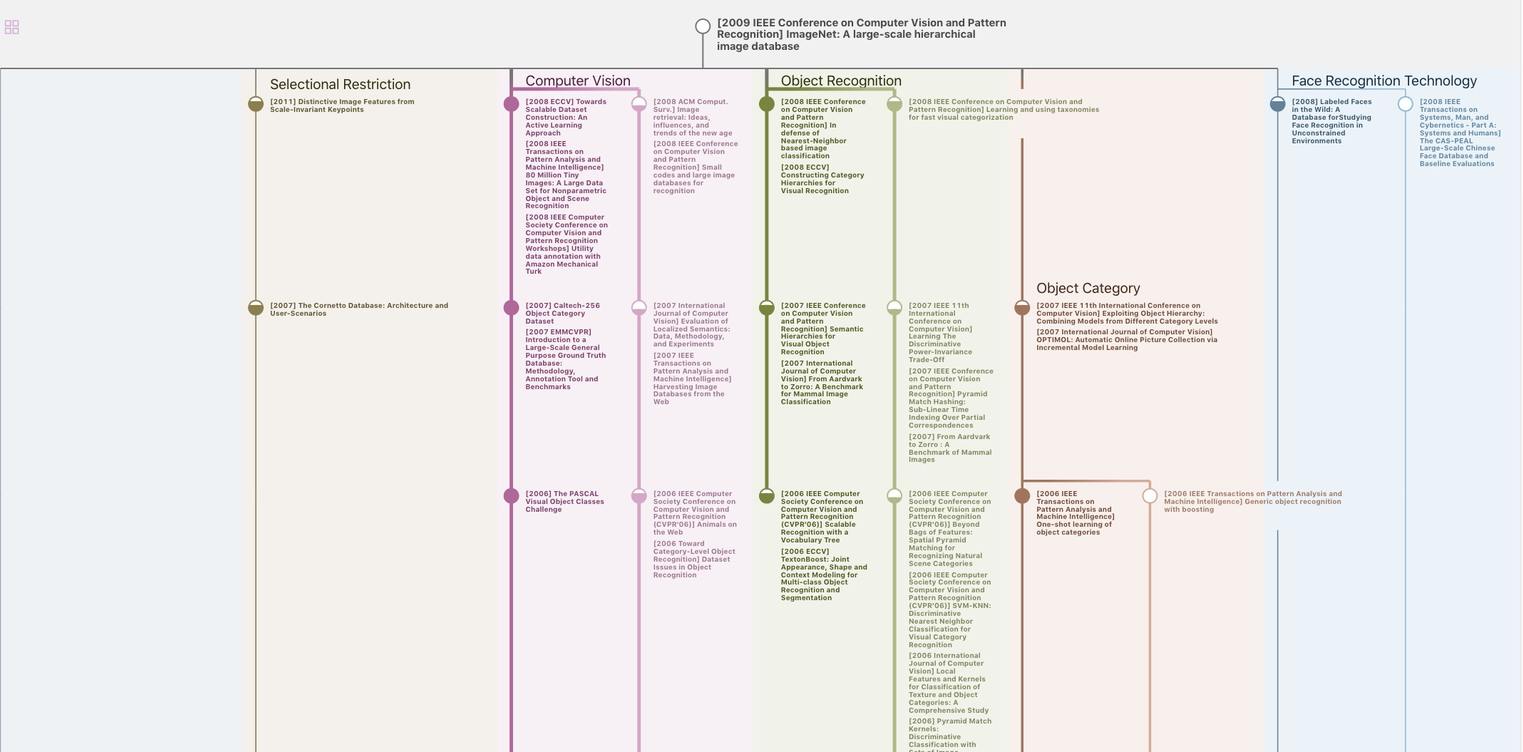
生成溯源树,研究论文发展脉络
Chat Paper
正在生成论文摘要