An Observing System Simulation Experiment Analysis of How Well Geostationary Satellite Trace-Gas Observations Constrain NOx Emissions in the US
JOURNAL OF GEOPHYSICAL RESEARCH-ATMOSPHERES(2024)
Abstract
We investigate the benefit of assimilating high spatial-temporal resolution nitrogen dioxide (NO2) measurements from a geostationary (GEO) instrument such as Tropospheric Emissions: Monitoring of Pollution (TEMPO) versus a low-earth orbit (LEO) platform like TROPOspheric Monitoring Instrument (TROPOMI) on the inverse modeling of nitrogen oxides (NOx) emissions. We generated synthetic TEMPO and TROPOMI NO2 measurements based on emissions from the COVID-19 lockdown period. Starting with emissions levels prior to the lockdown, we use the Weather Research and Forecasting Model coupled with Chemistry/Data Assimilation Research Testbed (WRF-Chem/DART) to assimilate these pseudo-observations in Observing System Simulation Experiments to adjust NOx emissions and quantify how well the assimilation of TEMPO versus TROPOMI measurements recovers the lockdown-induced emissions changes. We find that NOx emission biases can be ameliorated using half as many simulation days when assimilating GEO observations, and the estimated NOx emissions in 23 out of 29 major urban regions in the US are more accurate. The root mean square error and coefficient of determination of posterior NOx emissions are reduced by 12.5%-41.5% and 1.5%-17.1%, respectively, across different regions. We conduct sensitivity experiments that use different data assimilation (DA) configurations to assimilate synthetic GEO observations. Results demonstrate that the temporal width of the DA window introduces -10% to -20% biases in the emissions inversion and constraining both NOx concentrations and emissions simultaneously yields the most accurate NOx emissions estimates. Our work serves as a valuable reference on how to appropriately assimilate GEO observations for constraining NOx emissions in future studies.
MoreTranslated text
AI Read Science
Must-Reading Tree
Example
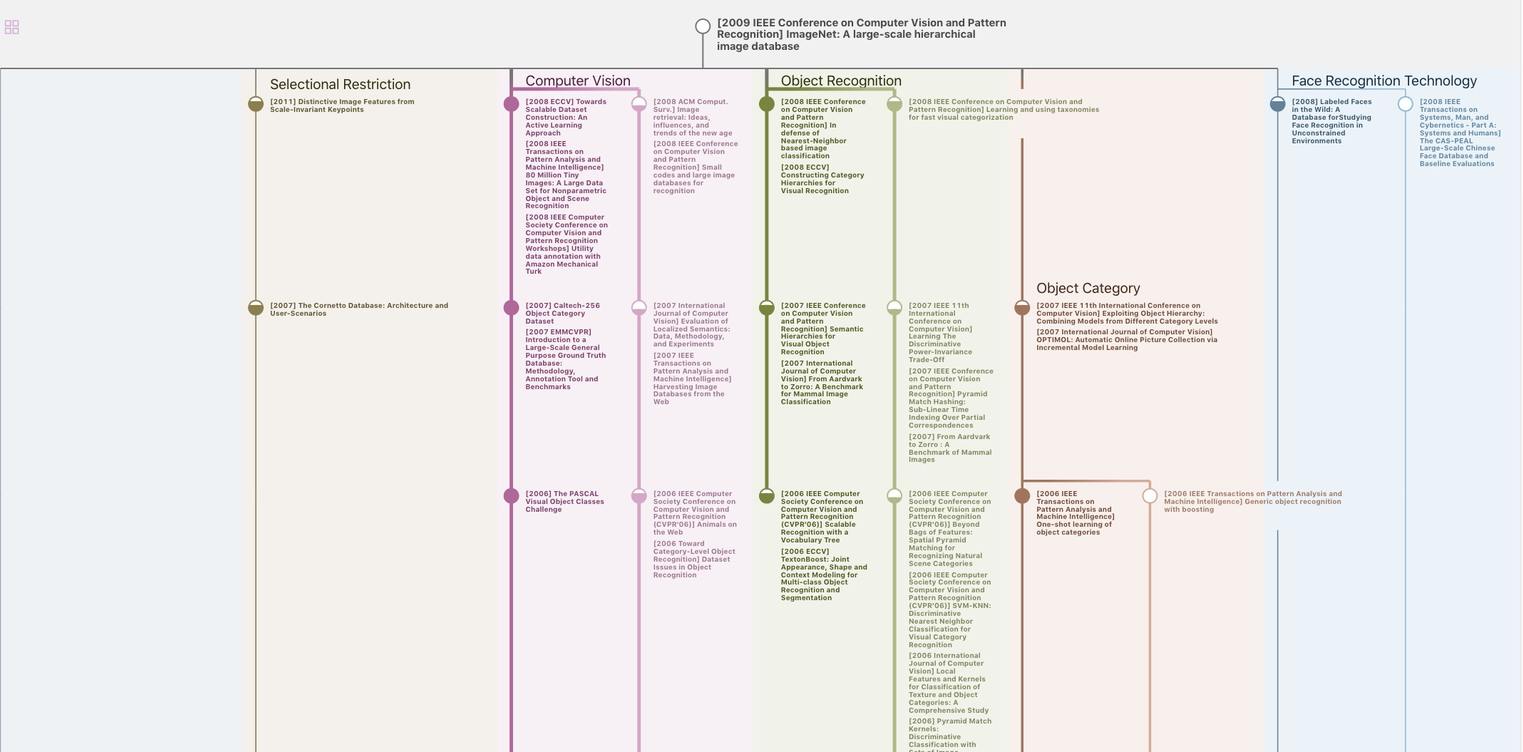
Generate MRT to find the research sequence of this paper
Chat Paper
Summary is being generated by the instructions you defined