Approximate Kernel Learning Uncertainty Set for Robust Combinatorial Optimization
INFORMS JOURNAL ON COMPUTING(2024)
摘要
Support vector clustering (SVC) has been proposed in the literature as a datadriven approach to build uncertainty sets in robust optimization. Unfortunately, the resulting SVC-based uncertainty sets induces a large number of additional variables and constraints in the robust counterpart of mathematical formulations. We propose a two-phase method to approximate the resulting uncertainty sets and overcome these tractability issues. This method is controlled by a parameter defining a trade-off between the quality of the approximation and the complexity of the robust models formulated. We evaluate the approximation method on three distinct, well-known optimization problems. Experimental results show that the approximated uncertainty set leads to solutions that are comparable to those obtained with the classic SVC-based uncertainty set with a significant reduction of the computation time.
更多查看译文
关键词
data-driven,robust optimization,machine learning,mixed-integer linear programming
AI 理解论文
溯源树
样例
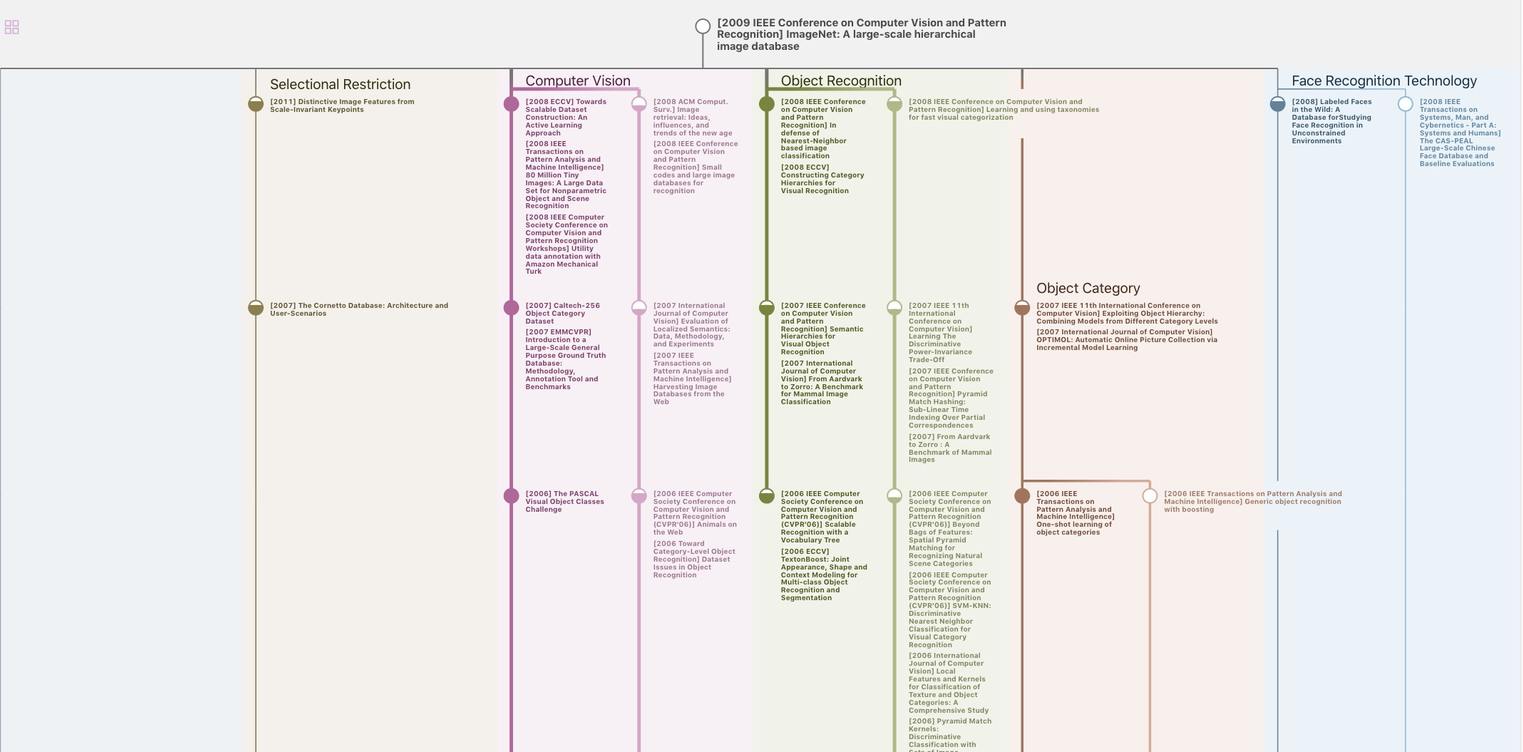
生成溯源树,研究论文发展脉络
Chat Paper
正在生成论文摘要