Consistent Valid Physically-Realizable Adversarial Attack Against Crowd-flow Prediction Models
IEEE transactions on intelligent transportation systems(2023)
摘要
Recent works have shown that deep learning (DL) models can effectively learn city-wide crowd-flow patterns, which can be used for more effective urban planning and smart city management. However, DL models have been known to perform poorly on inconspicuous adversarial perturbations. Although many works have studied these adversarial perturbations in general, the adversarial vulnerabilities of deep CFP models in particular have remained largely unexplored. In this paper, we perform a rigorous analysis of the adversarial vulnerabilities of DL-based CFP models under multiple threat settings, making three-fold contributions; 1) we propose by formally identifying two novel properties-Consistency and Validity-of the CFP inputs that enable the detection of standard adversarial inputs with 0% false acceptance rate (FAR); 2) we leverage universal adversarial perturbations and an adaptive adversarial loss to present adaptive adversarial attacks to evade defense; 3) we propose, a Consistent, Valid and Physically-realizable adversarial attack, that explicitly inducts the consistency and validity priors in the perturbation generation mechanism. We find out that although the crowd-flow models are vulnerable to adversarial perturbations, it is extremely challenging to simulate these perturbations in physical settings, notably when is in place. We also show that attack considerably outperforms the adaptively modified standard attacks in FAR and adversarial loss metrics. We conclude with useful insights emerging from our work and highlight promising future research directions.
更多查看译文
关键词
Perturbation methods,Standards,Adaptation models,Computer architecture,Analytical models,History,Data models,Deep neural networks,CFP,adversarial ML
AI 理解论文
溯源树
样例
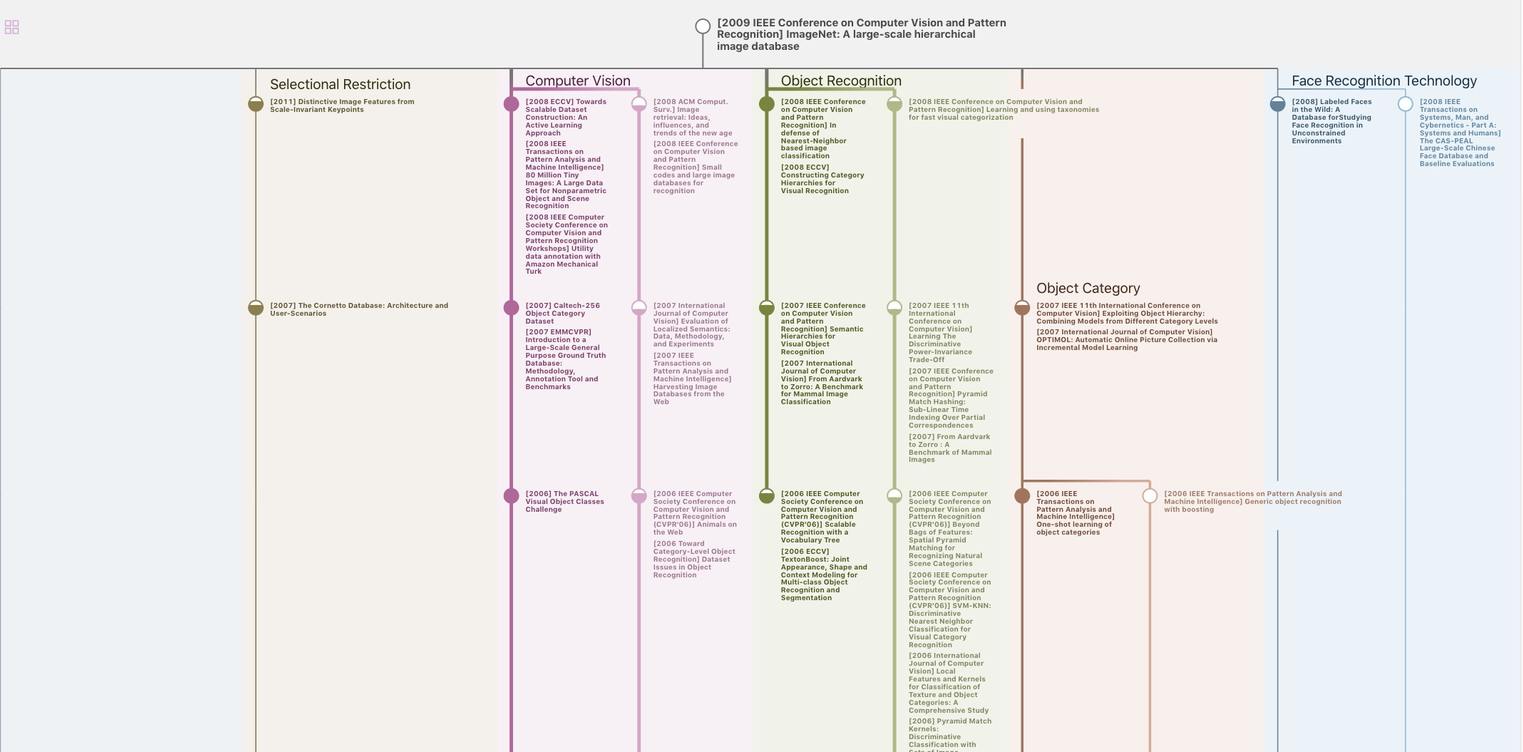
生成溯源树,研究论文发展脉络
Chat Paper
正在生成论文摘要