N − K Static Security Assessment for Power Transmission System Planning Using Machine Learning
ENERGIES(2024)
摘要
This paper presents a methodology for static security assessment of transmission network planning using machine learning (ML). The objective is to accelerate the probabilistic risk assessment of the Hydro-Quebec (HQ) TransÉnergie transmission grid. The model takes the expected power supply and the status of the elements in a N−k contingency scenario as inputs. The output is the reliability metric Expecting Load Shedding Cost (ELSC). To train and test the regression model, stochastic data are performed, resulting in a set of N−k and k=1,2,3 contingency scenarios used as inputs. Subsequently, the output is computed for each scenario by performing load shedding using an optimal power flow algorithm, with the objective function of minimizing ELSC. Experimental results on the well-known IEEE-39 bus test system and PEGASE-1354 system demonstrate the potential of the proposed methodology in generalizing ELSC during an N−k contingency. For up to k=3 the coefficient of determination R2 obtained was close to 98% for both case studies, achieving a speed-up of over four orders of magnitude with the use of a Multilayer Perceptron (MLP). This approach and its results have not been addressed in the literature, making this methodology a contribution to the state of the art.
更多查看译文
关键词
load shedding optimal power flow,machine learning,static security assessment,transmission system planning
AI 理解论文
溯源树
样例
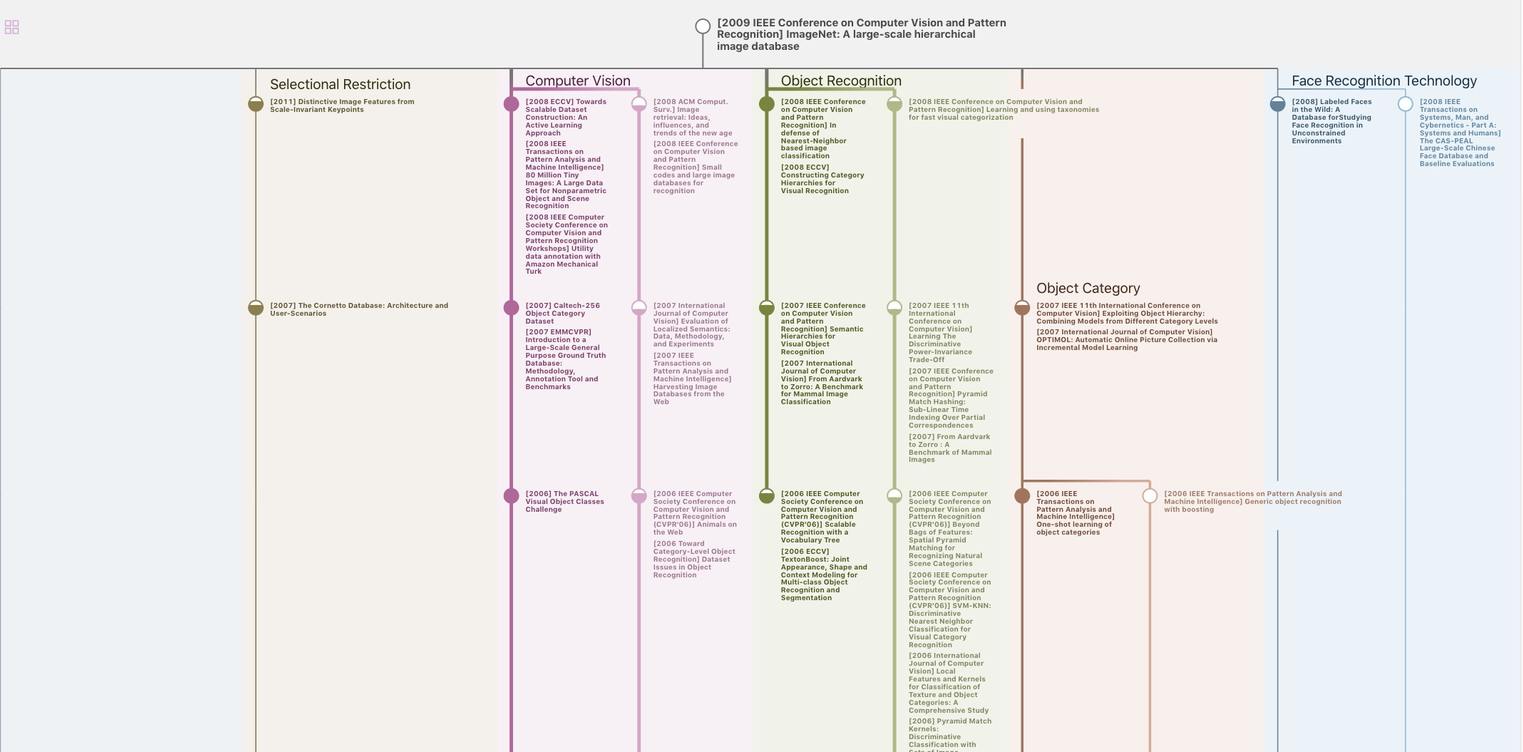
生成溯源树,研究论文发展脉络
Chat Paper
正在生成论文摘要