Load Day-Ahead Automatic Generation Control Reserve Capacity Demand Prediction Based on the Attention-BiLSTM Network Model Optimized by Improved Whale Algorithm
ENERGIES(2024)
摘要
Load forecasting is a research hotspot in academia; in the context of new power systems, the prediction and determination of load reserve capacity is also important. In order to adapt to new forms of power systems, a day-ahead automatic generation control (AGC) reserve capacity demand prediction method based on the Fourier transform and the attention mechanism combined with a bidirectional long and short-term memory neural network model (Attention-BiLSTM) optimized by an improved whale optimization algorithm (IWOA) is proposed. Firstly, based on the response time, Fourier transform is used to refine the distinction between various types of load reserve demand, and the power of the AGC reserve band is calculated using Parseval's theorem to obtain the reserve capacity demand sequence. The maximum mutual information coefficient method is used to explore the relevant influencing factors of the AGC reserve sequence concerning the data characteristics of the AGC reserve sequence. Then, the historical daily AGC reserve demand sequences with relevant features are input into the Attention-BiLSTM prediction model, and the improved whale algorithm is used to automatically find the optimal hyperparameters to obtain better prediction results. Finally, the arithmetic simulation results show that the model proposed in this paper has the best prediction performance with the upper (0.8810) and lower (0.6651) bounds of the coefficient of determination (R2) higher than the other models, and it has the smallest mean absolute percentage error (MAPE) and root mean square error (RMSE).
更多查看译文
关键词
load AGC reserve capacity prediction,improved whale algorithm,long and short-term memory,Fourier transform
AI 理解论文
溯源树
样例
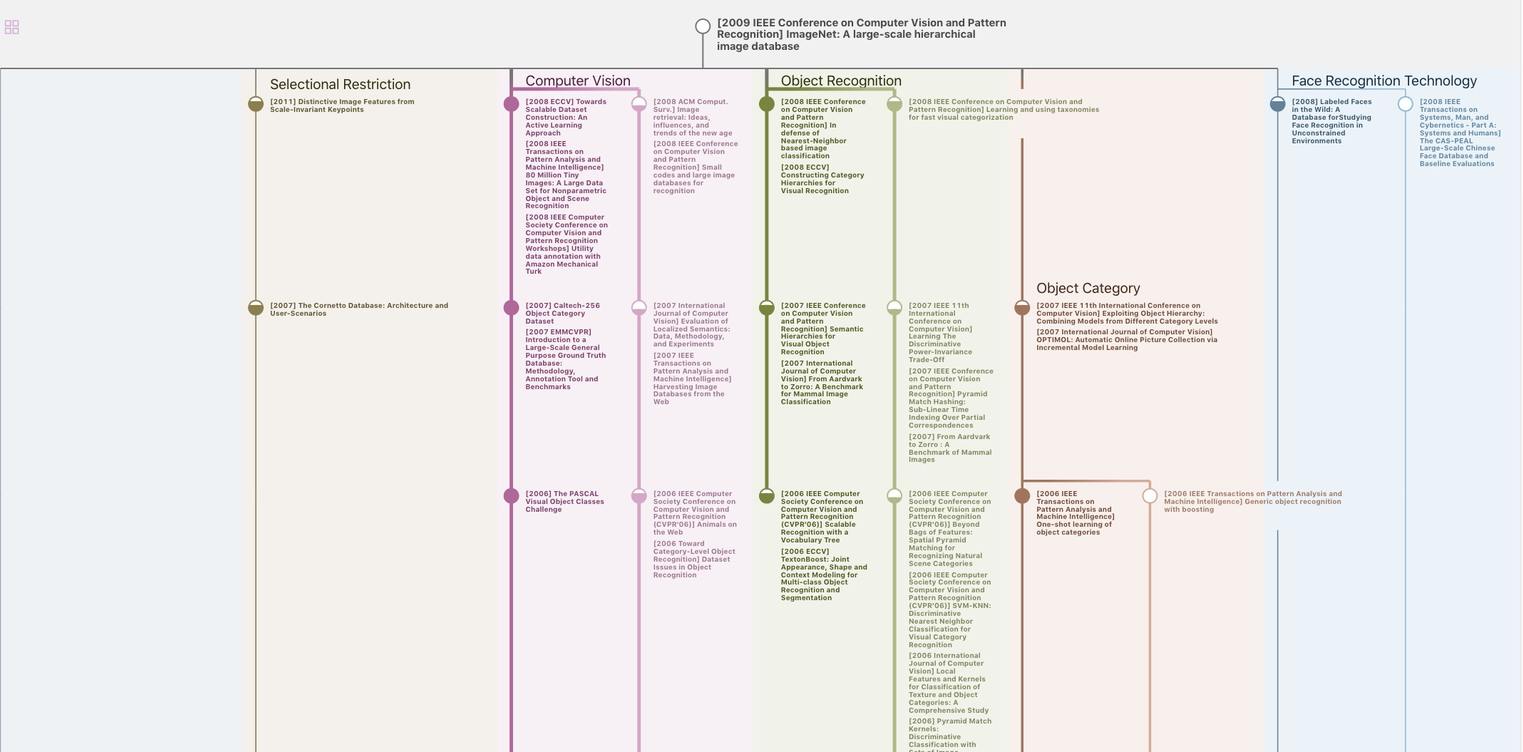
生成溯源树,研究论文发展脉络
Chat Paper
正在生成论文摘要