Rethinking Polyp Segmentation from an Out-of-distribution Perspective.
Deleted Journal(2024)
摘要
Unlike existing fully-supervised approaches, we rethink colorectal polyp segmentation from an out-of-distribution perspective with a simple but effective self-supervised learning approach. We leverage the ability of masked autoencoders-self-supervised vision transformers trained on a reconstruction task-to learn in-distribution representations, here, the distribution of healthy colon images. We then perform out-of-distribution reconstruction and inference, with feature space standardisation to align the latent distribution of the diverse abnormal samples with the statistics of the healthy samples. We generate per-pixel anomaly scores for each image by calculating the difference between the input and reconstructed images and use this signal for out-of-distribution (i.e., polyp) segmentation. Experimental results on six benchmarks show that our model has excellent segmentation performance and generalises across datasets. Our code is publicly available at https://github.com/GewelsJI/Polyp-OOD.
更多查看译文
关键词
Polyp segmentation,anomaly segmentation,out-of-distribution segmentation,masked autoencoder,abdomen
AI 理解论文
溯源树
样例
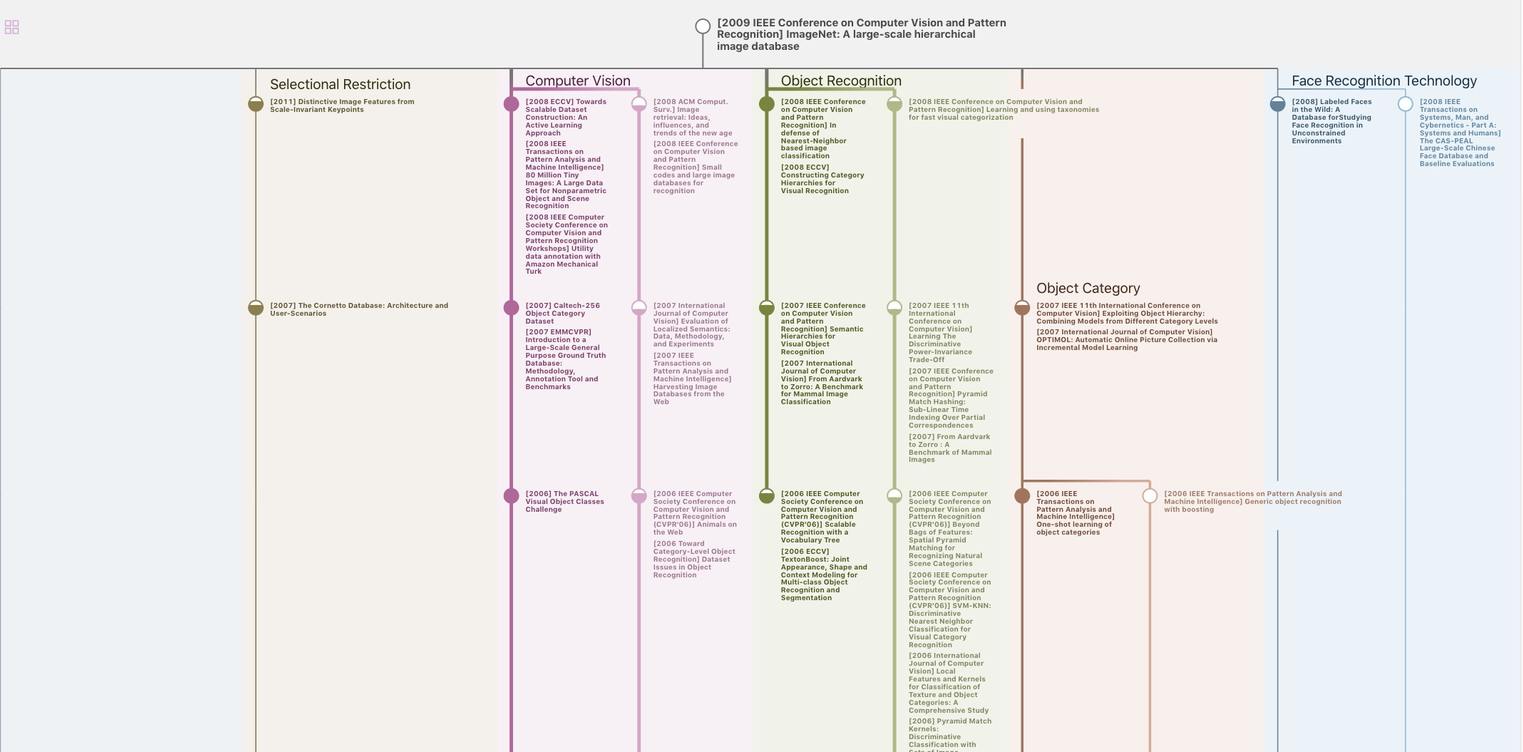
生成溯源树,研究论文发展脉络
Chat Paper
正在生成论文摘要