A Theoretical Framework for Unsupervised Land Cover Change Detection in Dense Satellite Image Time Series
IMAGE AND SIGNAL PROCESSING FOR REMOTE SENSING XXIX(2023)
Abstract
This paper addresses the complex task of detecting and characterizing changes in dense Satellite Image Time Series (SITS). Although Change Vector Analysis (CVA) is widely used for Change Detection (CD), it has limitations due to missing prior information on changes, such as: optimal spectral channels and change timing. Time series data can help overcome these limitations, but working with them is challenging. To address these challenges, the paper introduces a novel framework called Time Series Change Vector Analysis (TSCVA), which builds upon the principles of CVA. In TSCVA, the paper redefines CVA in the time series feature space and introduces new definitions for change in time series magnitude and direction. This allows for a detailed analysis of change components in the time and spectrum domain within the SITS, enabling unsupervised CD. We utilize the expectation-maximization algorithm to estimate parameters of statistical distributions for change and nochange classes. The effectiveness of the proposed TSCVA method is evaluated using Sentinel-2 time series data. The results, both quantitative and qualitative, confirm the robustness of this approach in effectively addressing the CD problem in dense SITS.
MoreTranslated text
Key words
Remote Sensing,Multi-Temporal,Multi-spectral,Unsupervised Change Detection (CD),Satellite Image Time Series (SITS),Change Vector Analysis (CVA)
AI Read Science
Must-Reading Tree
Example
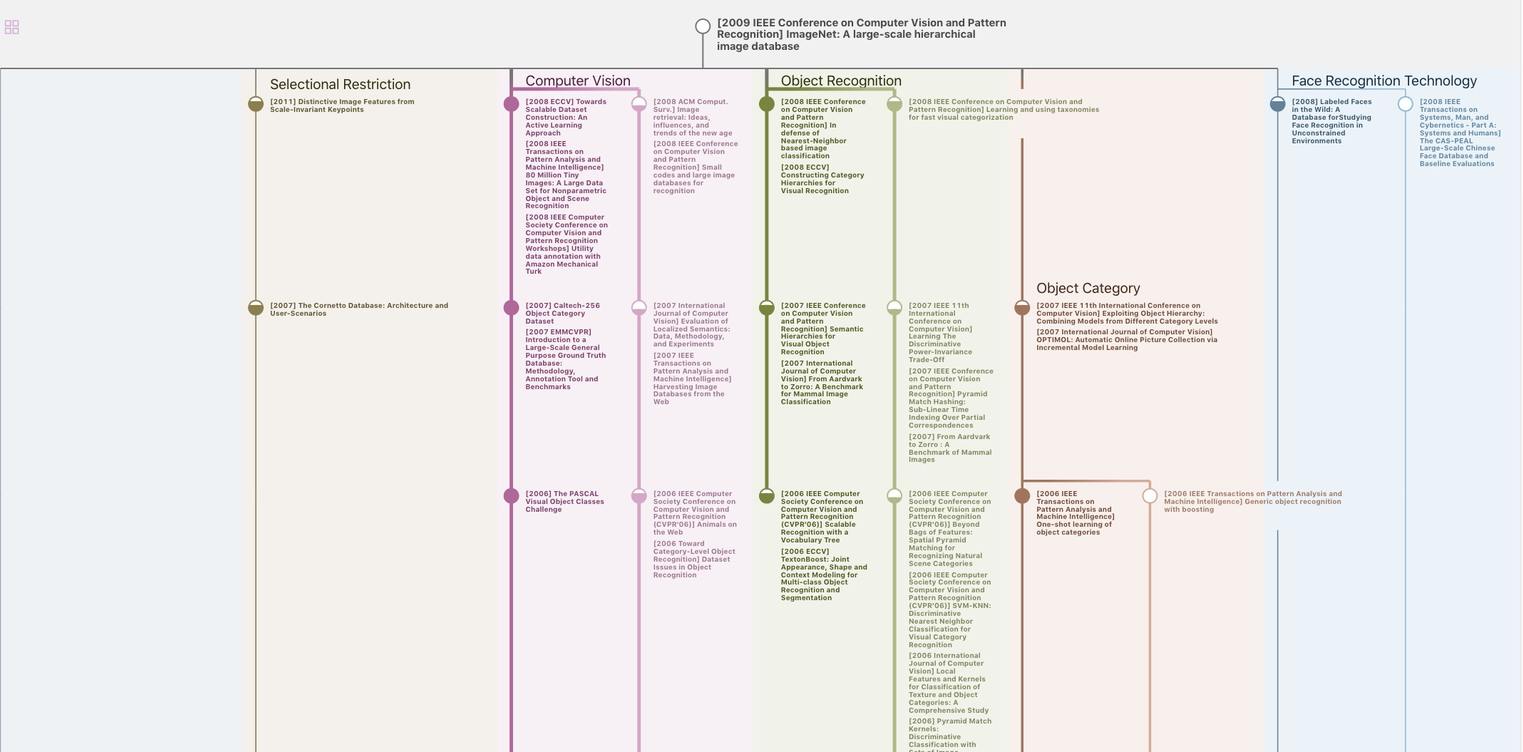
Generate MRT to find the research sequence of this paper
Chat Paper
Summary is being generated by the instructions you defined