Generative Representational Instruction Tuning
arXiv (Cornell University)(2024)
摘要
All text-based language problems can be reduced to either generation orembedding. Current models only perform well at one or the other. We introducegenerative representational instruction tuning (GRIT) whereby a large languagemodel is trained to handle both generative and embedding tasks bydistinguishing between them through instructions. Compared to other openmodels, our resulting GritLM 7B sets a new state of the art on the Massive TextEmbedding Benchmark (MTEB) and outperforms all models up to its size on a rangeof generative tasks. By scaling up further, GritLM 8x7B outperforms all opengenerative language models that we tried while still being among the bestembedding models. Notably, we find that GRIT matches training on onlygenerative or embedding data, thus we can unify both at no performance loss.Among other benefits, the unification via GRIT speeds up Retrieval-AugmentedGeneration (RAG) by > 60retrieval and generation models. Models, code, etc. are freely available athttps://github.com/ContextualAI/gritlm.
更多查看译文
关键词
Collaborative Learning,Cooperative Learning
AI 理解论文
溯源树
样例
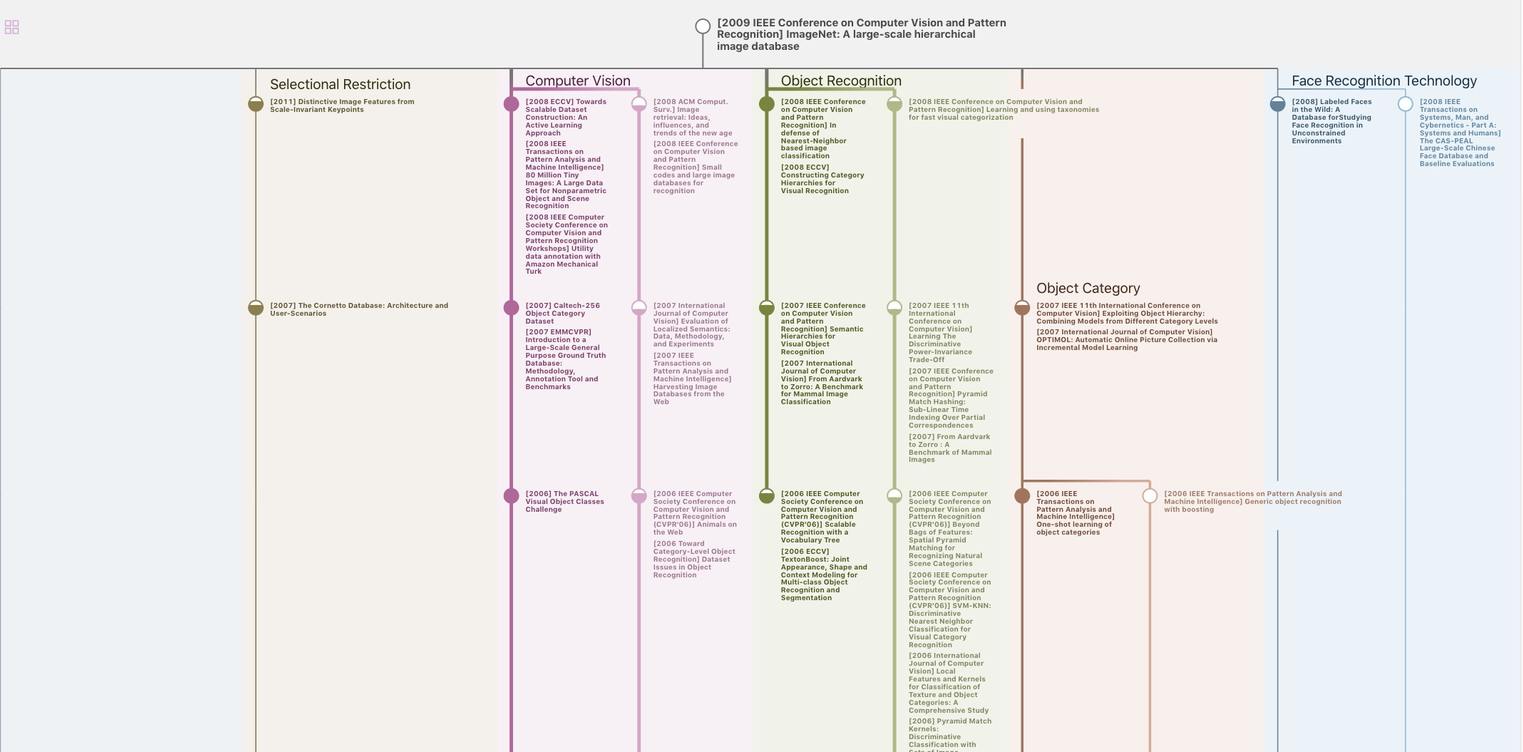
生成溯源树,研究论文发展脉络
Chat Paper
正在生成论文摘要