Enhancing Transvenous Lead Extraction Risk Prediction: Integrating Imaging Biomarkers into Machine Learning Models.
HEART RHYTHM(2024)
摘要
BACKGROUND Machine learning (ML) models have been proposed to predict risk related to transvenous lead extraction (TLE). OBJECTIVE The purpose of this study was to test whether integrating imaging data into an existing ML model increases its ability to predict major adverse events (MAEs; procedure-related major complications and procedure-related deaths) and lengthy procedures (>= 100 minutes). METHODS We hypothesized certain features-(1) lead angulation, (2) coil percentage inside the superior vena cava (SVC), and (3) number of overlapping leads in the SVC-detected from a pre-TLE plain anteroposterior chest radiograph (CXR) would improve prediction of MAE and long procedural times. A deep-learning convolutional neural network was developed to automatically detect these CXR features. RESULTS A total of 1050 cases were included, with 24 MAEs (2.3%) . The neural network was able to detect (1) heart border with 100% accuracy; (2) coils with 98% accuracy; and (3) acute angle in the right ventricle and SVC with 91% and 70% accuracy, respectively. The following features significantly improved MAE prediction: (1) >= 50% coil within the SVC; (2) >= 2 overlapping leads in the SVC; and (3) acute lead angulation. Balanced accuracy (0.74-0.87), sensitivity (68%-83%), specificity (72%-91%), and area under the curve (AUC) (0.767-0.962) all improved with imaging biomarkers. Prediction of lengthy procedures also improved: balanced accuracy (0.76-0.86), sensitivity (75%-85%), specificity (63%-87%), and AUC (0.684-0.913). CONCLUSION Risk prediction tools integrating imaging biomarkers significantly increases the ability of ML models to predict risk of MAE and long procedural time related to TLE.
更多查看译文
关键词
Transvenous lead extraction,Complications,Machine Learning,Arti fi cial intelligence,Risk prediction,Computer vision
AI 理解论文
溯源树
样例
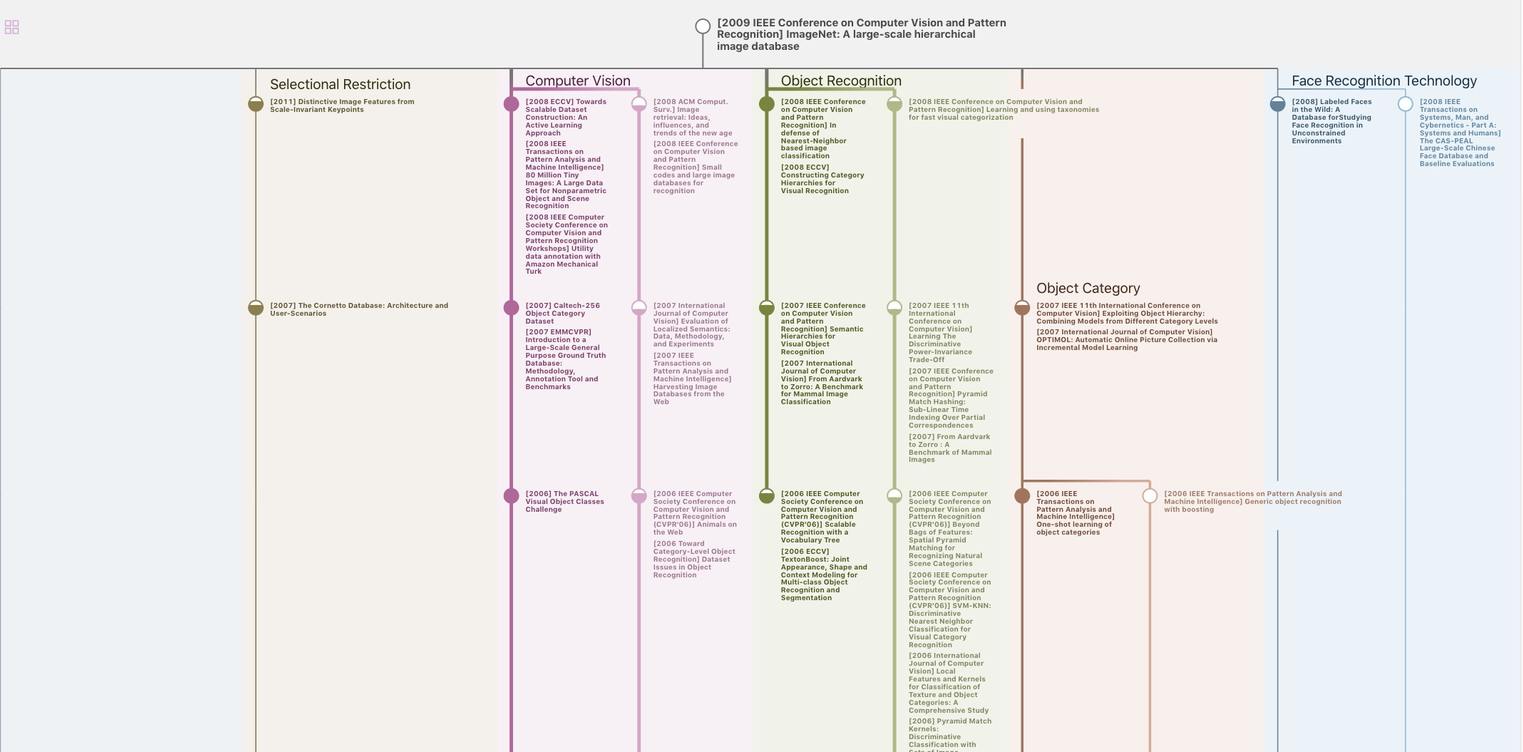
生成溯源树,研究论文发展脉络
Chat Paper
正在生成论文摘要