HierCoop: Hierarchical Cooperative Resource Allocation for Vehicular Communications in Heterogeneous Networks.
International Conference on Communication Technology(2023)
摘要
Heterogeneous Networks (HetNets) can provide intelligent vehicles with high-bandwidth access and support large-scale vehicle-infrastructure cooperation. However, the spectrum allocation of HetNets faces the problem of mutual coupling between macrocell base station (MBS) and intensively deployed small-cell base stations (SBSs) which share spectrum band. While recent research has used Multi-Agent Deep Reinforcement Learning (MADRL) to allocate resources for vehicle-infrastructure cooperation, it has focused solely on intra-cell resource distribution, overlooking inter-cell resource cooperation. Inter-cell resource cooperation in HetN ets faces significant communication overhead due to real-time link state interaction between base stations. Additionally, timely updates to inter-cell resource allocation policies are critical when vehicular communication patterns undergo shifts. In this paper, we propose a hierarchical cooperative resource allocation method (HierCoop) for addressing the aforementioned challenges in vehicular communications in HetN ets. Each base station is modeled as an agent with hierarchical structure which combines coarse-grained inter-cell collaboration policies and fine-grained inner-cell resource allocation. Specifically, the upper layer of each agent is deployed centrally, thus enabling inter-cell collaboration policies to be based on the abstract channel state features extracted from the lower layers instead of the original channel state information. Meanwhile, the lower layer of each agent is deployed locally in base station, which executes actual resource allocation action based on the policy formulated by the upper layer. The experimental results demonstrate that HierCoop can significantly enhances spectral efficiency and communication quality.
更多查看译文
关键词
heterogeneous networks,vehicular communications,resource allocation,hierarchical multi-agent reinforcement learning
AI 理解论文
溯源树
样例
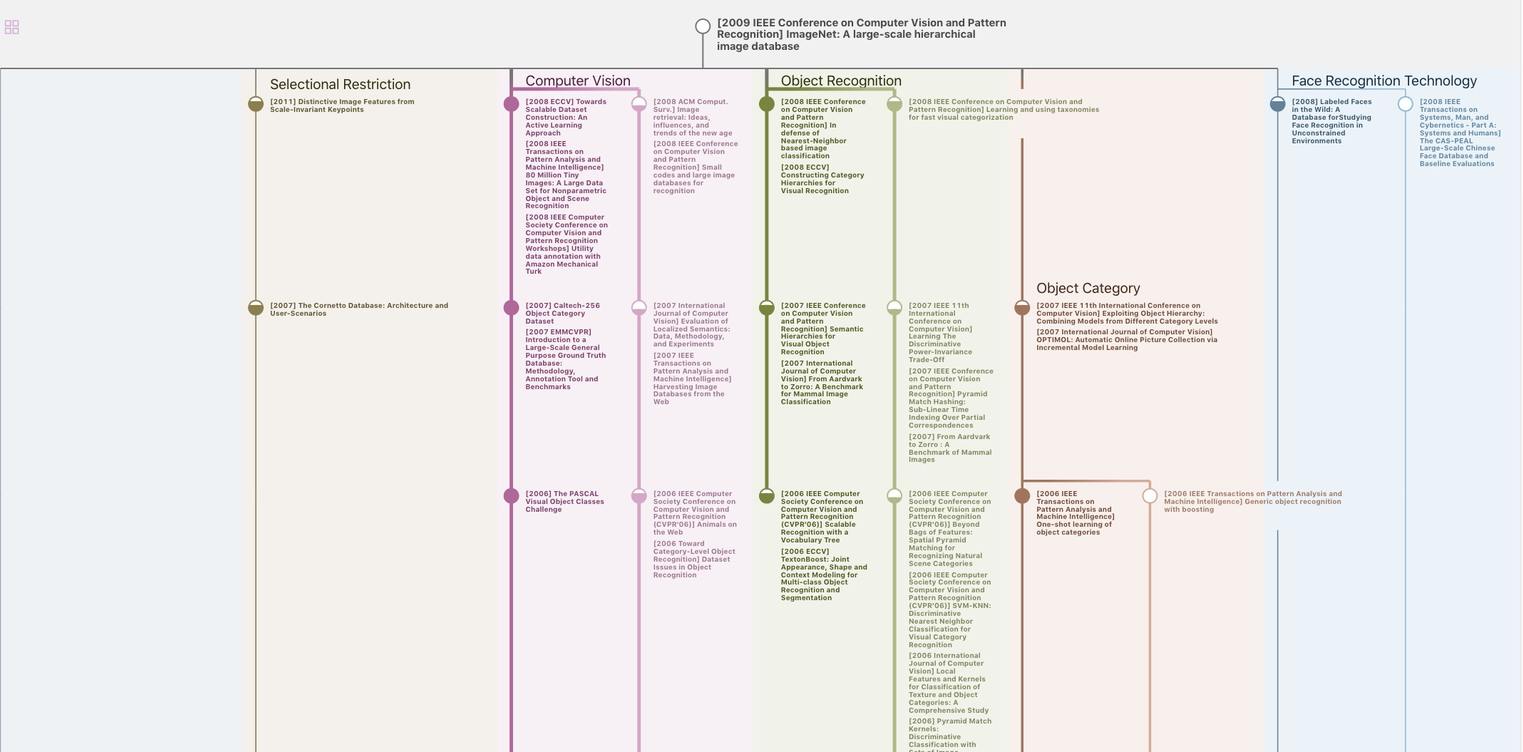
生成溯源树,研究论文发展脉络
Chat Paper
正在生成论文摘要