A Multi-Object Tracking Method With Adaptive Dual Decoder and Better Motion Affinity
IEEE ACCESS(2024)
摘要
For multi-object tracking (MOT), jointly learning the detector and embedding model (JDE) is one of the mainstream solutions. However, an inherent problem in this architecture arises as the tasks of target detection and appearance feature extraction compete with each other. FairMOT, as a representative method, attempts to address this issue by employing two homogeneous branches, but it overlooks the essential difference between these two tasks. Upon the original network architecture, we propose an adaptive dual decoder structure. Our objective is to separately learn more focused features for the target detection and the appearance feature extraction. Furthermore, we introduce a noise-adaptive Kalman filter based on the width estimation. In the motion information matching stage, we enhance the affinity matrix of motion information by employing an expanded-width strategy, combined with a more accurate overlap measure. We verify the effectiveness of our proposed approach through extensive experiments using the MOT17 dataset.
更多查看译文
关键词
Multi-object tracking,adaptive dual decoder,Kalman filter,motion information association,MOT17
AI 理解论文
溯源树
样例
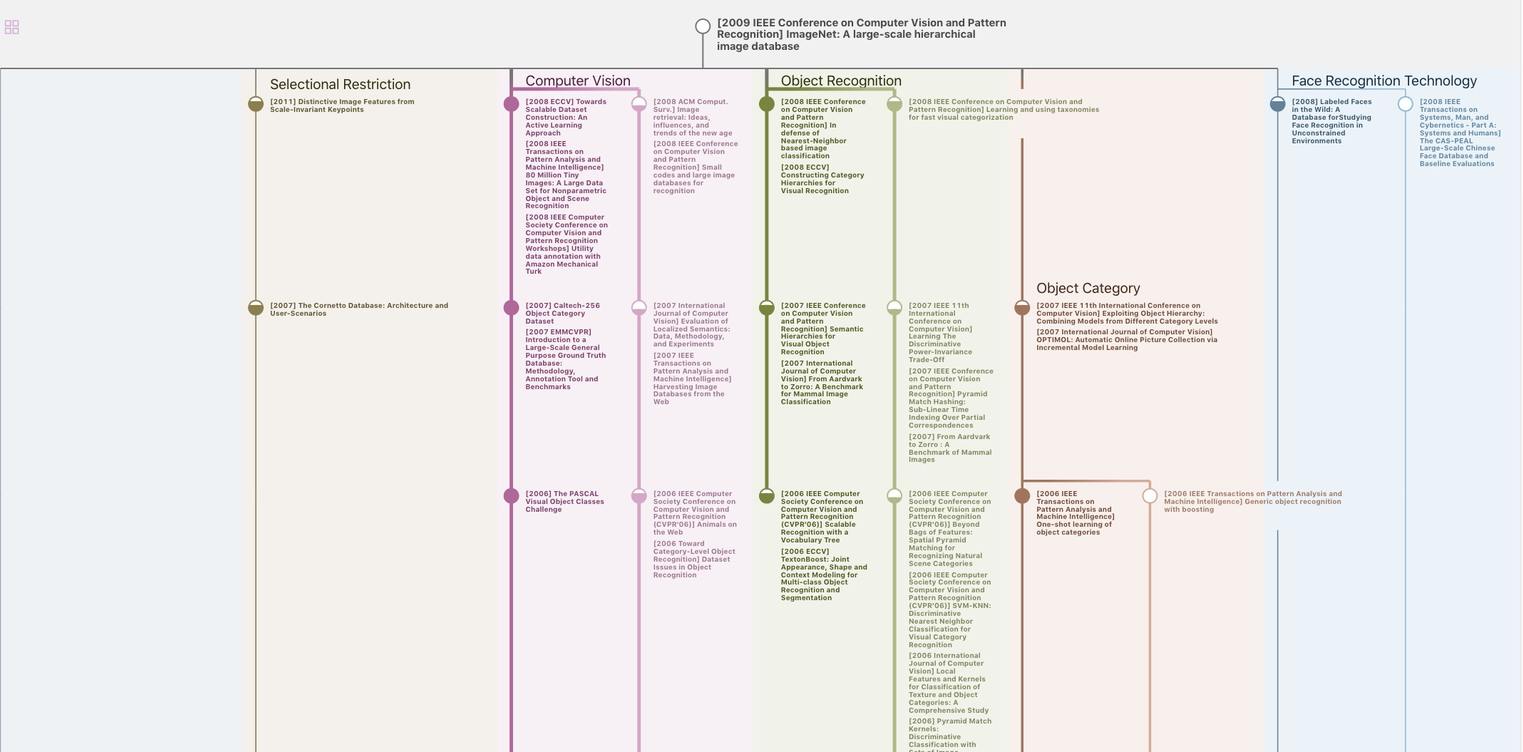
生成溯源树,研究论文发展脉络
Chat Paper
正在生成论文摘要