Debiasing Recommendation with Personal Popularity
WWW 2024(2024)
摘要
Global popularity (GP) bias is the phenomenon that popular items are
recommended much more frequently than they should be, which goes against the
goal of providing personalized recommendations and harms user experience and
recommendation accuracy. Many methods have been proposed to reduce GP bias but
they fail to notice the fundamental problem of GP, i.e., it considers
popularity from a global perspective of all users and uses a
single set of popular items, and thus cannot capture the interests of
individual users. As such, we propose a user-aware version of item popularity
named personal popularity (PP), which identifies different popular
items for each user by considering the users that share similar interests. As
PP models the preferences of individual users, it naturally helps to produce
personalized recommendations and mitigate GP bias. To integrate PP into
recommendation, we design a general personal popularity aware
counterfactual (PPAC) framework, which adapts easily to existing
recommendation models. In particular, PPAC recognizes that PP and GP have both
direct and indirect effects on recommendations and controls direct effects with
counterfactual inference techniques for unbiased recommendations. All codes and
datasets are available at .
更多查看译文
AI 理解论文
溯源树
样例
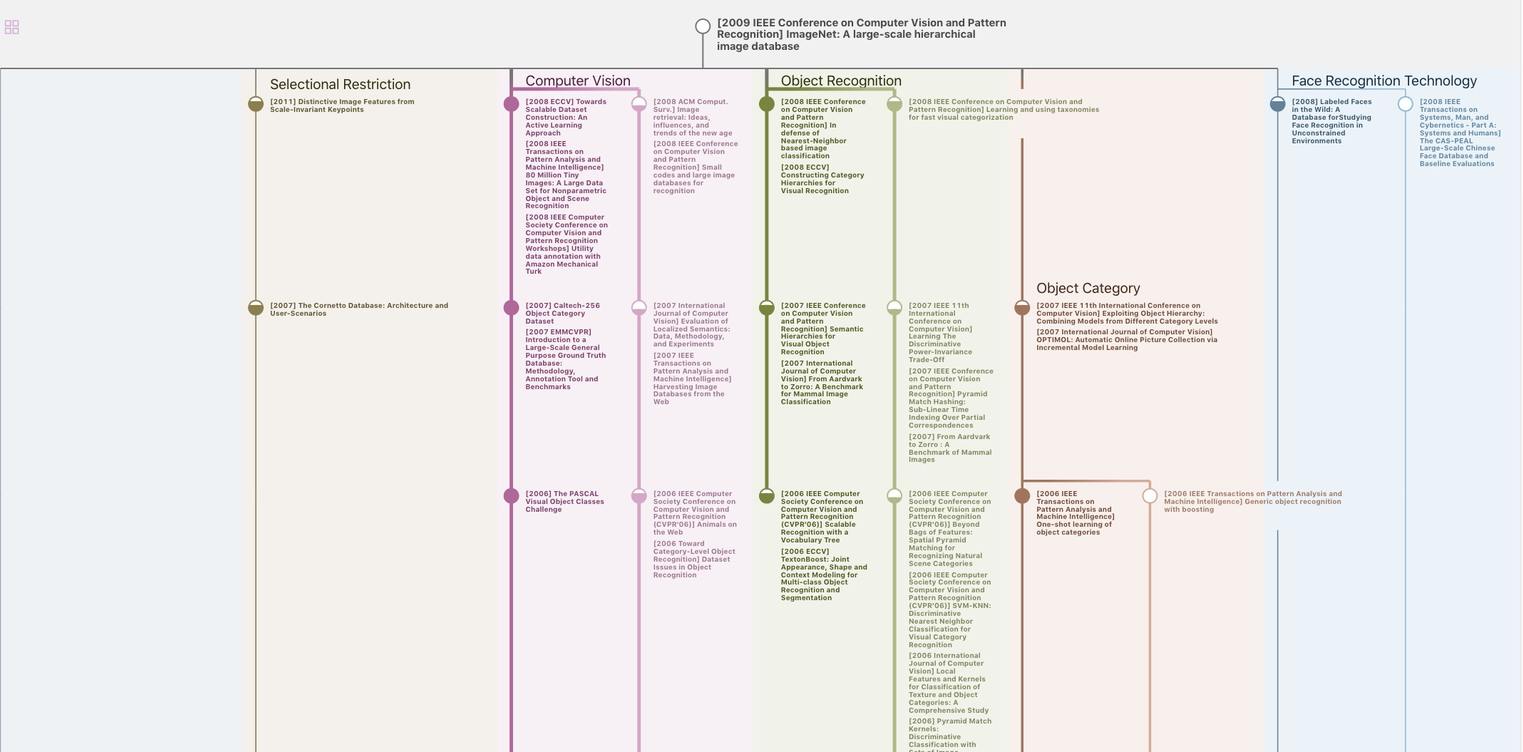
生成溯源树,研究论文发展脉络
Chat Paper
正在生成论文摘要