Simplifying Hyperparameter Derivation for Integration Neural Networks Using Information Criterion.
IEEE/SICE International Symposium on System Integration(2024)
摘要
When optimal design is performed using simulation, attempts have been made to construct highly accurate approximators using machine learning to address the conflicting issues of simulation accuracy and time. In response, an integration neural network (INN and INN2), which combines deductive and inductive knowledge, has been proposed to obtain highly accurate approximate solutions with a small amount of data. However, creating this evaluation data requires much time and effort. Therefore, this study focused on the information criterion, which statistically evaluates the balance between the input data's diversity and the model's accuracy. The possibility of optimizing the hyperparameters using only this information criterion (AIC, BIC) and training data, thus eliminating the need for evaluation data, was investigated. The results showed that INN and INN2 behaved differently. In INN, the appropriate structure using the information content criterion and evaluation data was consistent, and hyperparameter optimization without evaluation data was possible. On the other hand, for INN2, it was found necessary to use evaluation data.
更多查看译文
关键词
Neural Network,Information Criterion,Hyperparameters,Training Data,Accurate Estimation,Accuracy Of Model,Akaike Information Criterion,Bayesian Information Criterion,Hyperparameter Tuning,Small Amount Of Data,Learning Process,Complex Models,Multiple Regression Analysis,Basis Functions,Nonlinear Function,Prediction Error,Structural Optimization,Likelihood Function,Middle Layer,Standard Deviation Error,Proper Structure,Nonlinear Part,Physical Knowledge,Number Of Explanatory Variables,Nonlinear Estimation
AI 理解论文
溯源树
样例
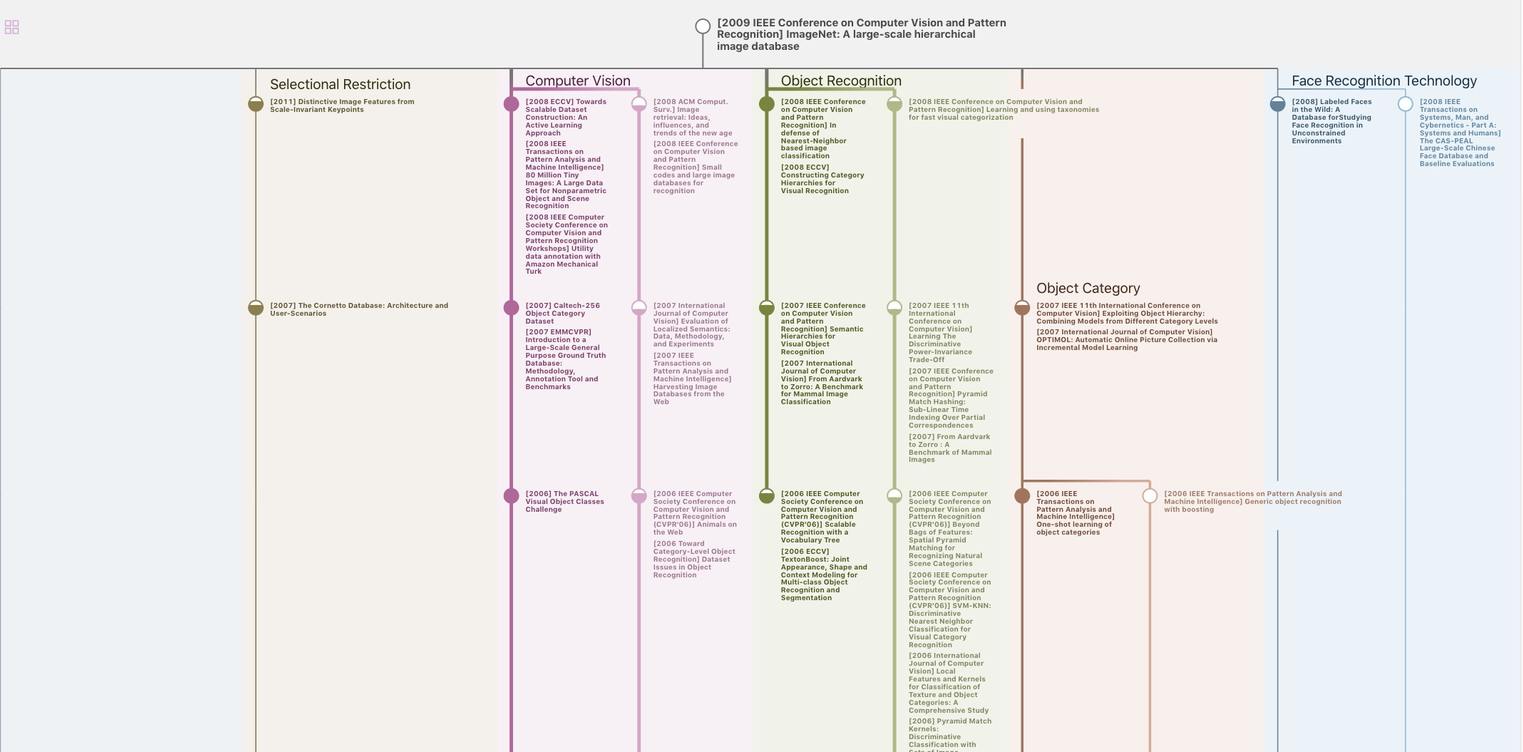
生成溯源树,研究论文发展脉络
Chat Paper
正在生成论文摘要