A NEW PROXY FOR REGIONAL PALEO‑ARIDITY USING CLUMPED AND TRIPLE OXYGEN ISOTOPES OF MODERN SOIL CARBONATES FROM THE SERENGETI ECOSYSTEM, TANZANIA
Abstracts with programs - Geological Society of America(2020)
Abstract
Paleosol carbonates are used extensively in the rock record to reconstruct vegetation using carbon isotopes, but poor constraints on temperature and soil evaporation has limited the use of oxygen isotopes in these reconstructions. Advances in carbonate clumped isotope thermometry allow for independent controls on temperature (T ), but proxies for evaporation remain elusive. The sensitivity of OOO distributions to kinetic fractionation makes it possible to track evaporation using triple oxygen isotope distributions (∆ʹ O). Recent work on lacustrine carbonates shows that ∆ʹ O is sensitive to evaporation, but little is known about variation of ∆ʹ O in soil carbonates or their potential to track evaporation. Here we report isotopic data from modern soil carbonates collected from 11 sites along a transect in the Serengeti Ecosystem where Mean Annual Precipitation (MAP) and Aridity Index (AI = MAP/PET) range from 499 to 846 mm yr and 0.33 to 0.55, respectively. δ C values range from ‐2.7 to 1.8‰ and reflect C grasslands. δ O values vary by ~8‰ along the transect and increase with increased aridity (lower MAP, higher AI). Mean T from these soil carbonates is 23 ± 4 ̊C and is within error of mean annual air temperature (MAAT) or 1-2 ̊C warmer at all sites. T does not vary significantly across sites or with depth, and soil temperature measurements and modeling suggest that this invariability is likely due to small changes in temperature at the equator. Using T temperatures, reconstructed soil water δ O values are up to 6‰ greater than δ O values of local precipitation and springs, indicating considerable evaporation in the soils. The ∆ʹ O values of these soil carbonates range from -161 to -111 per meg and decrease with increasing aridity and increasing δ O. This clear trend in ∆ʹ O values across an aridity gradient supports Monday, 26 October 2020 3:30 PM 3:45 PM GSA e-Attend Platform Meeting Rooms ∆47 18 17 16 17 17 17
MoreTranslated text
求助PDF
上传PDF
View via Publisher
AI Read Science
AI Summary
AI Summary is the key point extracted automatically understanding the full text of the paper, including the background, methods, results, conclusions, icons and other key content, so that you can get the outline of the paper at a glance.
Example
Background
Key content
Introduction
Methods
Results
Related work
Fund
Key content
- Pretraining has recently greatly promoted the development of natural language processing (NLP)
- We show that M6 outperforms the baselines in multimodal downstream tasks, and the large M6 with 10 parameters can reach a better performance
- We propose a method called M6 that is able to process information of multiple modalities and perform both single-modal and cross-modal understanding and generation
- The model is scaled to large model with 10 billion parameters with sophisticated deployment, and the 10 -parameter M6-large is the largest pretrained model in Chinese
- Experimental results show that our proposed M6 outperforms the baseline in a number of downstream tasks concerning both single modality and multiple modalities We will continue the pretraining of extremely large models by increasing data to explore the limit of its performance
Upload PDF to Generate Summary
Must-Reading Tree
Example
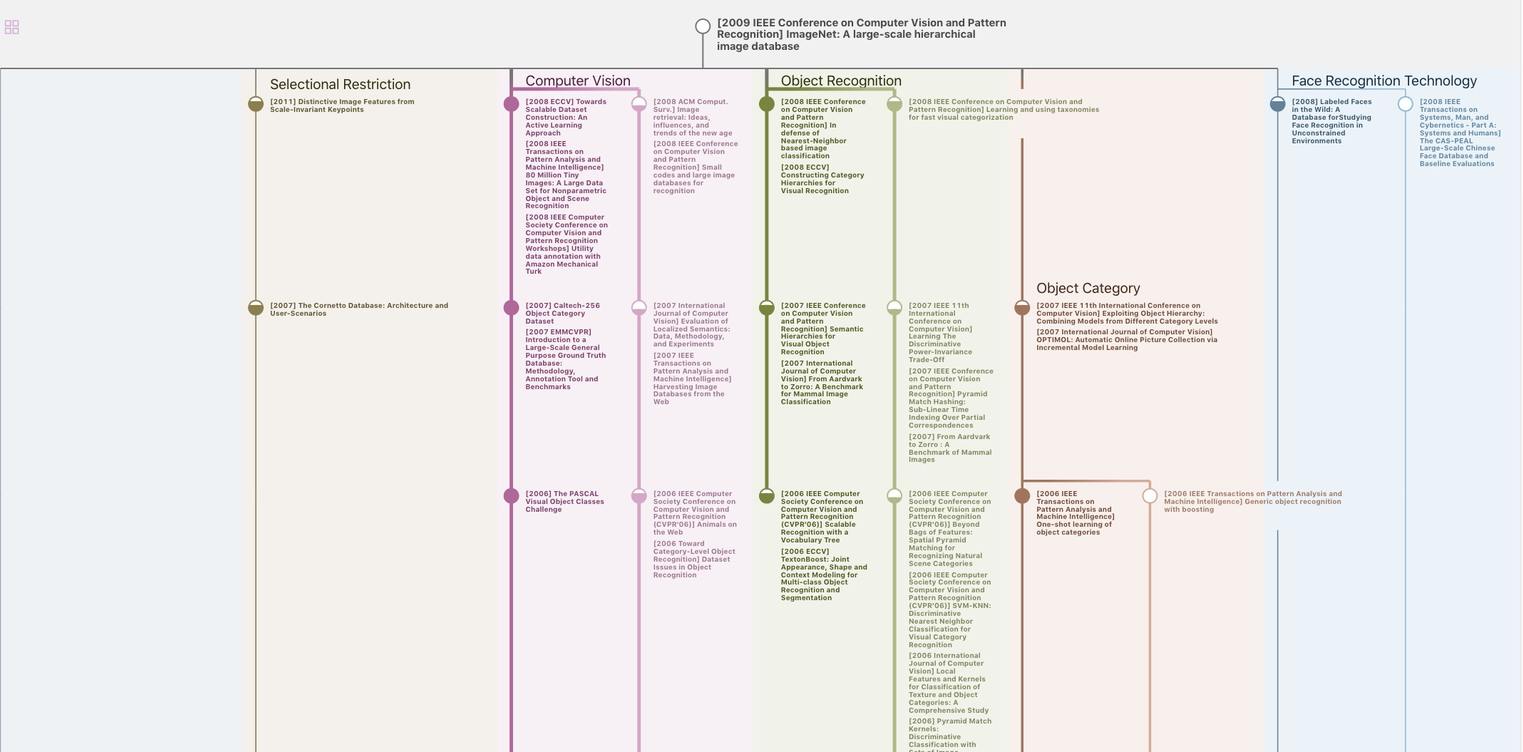
Generate MRT to find the research sequence of this paper
Data Disclaimer
The page data are from open Internet sources, cooperative publishers and automatic analysis results through AI technology. We do not make any commitments and guarantees for the validity, accuracy, correctness, reliability, completeness and timeliness of the page data. If you have any questions, please contact us by email: report@aminer.cn
Chat Paper
GPU is busy, summary generation fails
Rerequest