Easy-to-Hard Domain Adaptation With Human Interaction for Hyperspectral Image Classification.
IEEE Transactions on Geoscience and Remote Sensing(2024)
摘要
In real-world hyperspectral image classification (HSIC), the limited annotated examples usually lead to an insufficiently trained classifier, which further generates low classification accuracy. To overcome this challenge, domain adaptation (DA) methods have been developed to transfer learnable knowledge from the external HSIs with sufficient labeled examples (i.e., the source domain) to the interested HSIs with scarce labeled examples (i.e., the target domain). Conventional DA approaches often pseudo-label the examples with high classification confidence and then incorporate them into the training process. However, due to the significant domain gap, relying solely on confident examples may not be adequate to achieve satisfactory performance. Therefore, this article proposes an interactive easy-to-hard DA method (IEH-DA) to arrange the adaptation process so that the “easy” examples are adapted ahead of the “hard” ones. In an early stage, the easy examples with high pseudo-labeling confidence are selected for the adversarial learning-based DA. In a later stage, the “hard” examples with high informativity are further selected, and they are interactively labeled by human experts to provide accurate supervision information for adaptation. As a result, the examples in the target domain are used in an easy-to-hard way, which forms a curriculum sequence for orderly model training. Extensive experiments conducted on typical public datasets demonstrate that IEH-DA outperforms other state-of-the-art DA methods for HSIC.
更多查看译文
关键词
Curriculum learning (CL),domain adaptation (DA),example selection,hyperspectral image classification (HSIC)
AI 理解论文
溯源树
样例
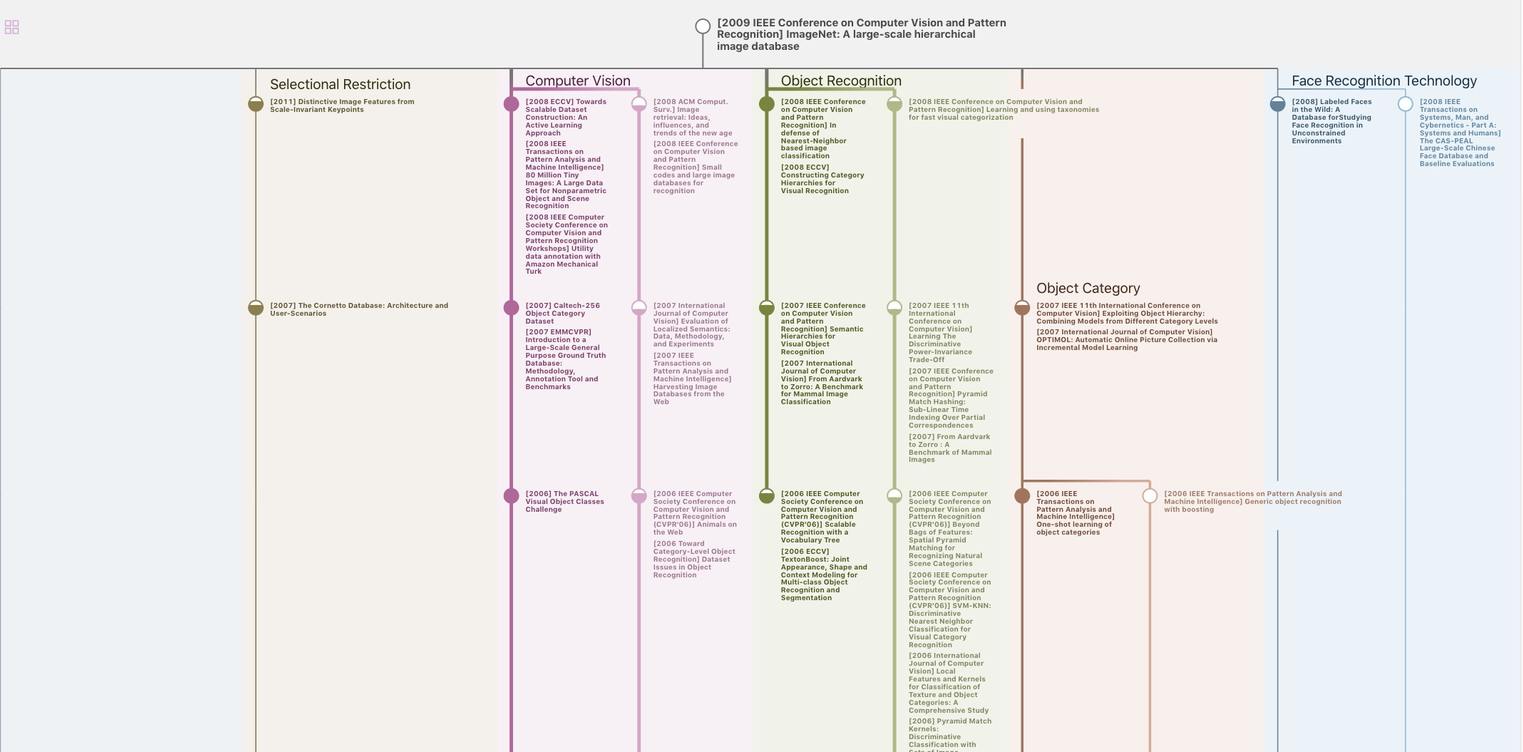
生成溯源树,研究论文发展脉络
Chat Paper
正在生成论文摘要