Assessing the Brittleness of Safety Alignment Via Pruning and Low-Rank Modifications
ICML 2024(2024)
摘要
Large language models (LLMs) show inherent brittleness in their safetymechanisms, as evidenced by their susceptibility to jailbreaking and evennon-malicious fine-tuning. This study explores this brittleness of safetyalignment by leveraging pruning and low-rank modifications. We develop methodsto identify critical regions that are vital for safety guardrails, and that aredisentangled from utility-relevant regions at both the neuron and rank levels.Surprisingly, the isolated regions we find are sparse, comprising about 3%at the parameter level and 2.5% at the rank level. Removing these regionscompromises safety without significantly impacting utility, corroborating theinherent brittleness of the model's safety mechanisms. Moreover, we show thatLLMs remain vulnerable to low-cost fine-tuning attacks even when modificationsto the safety-critical regions are restricted. These findings underscore theurgent need for more robust safety strategies in LLMs.
更多查看译文
AI 理解论文
溯源树
样例
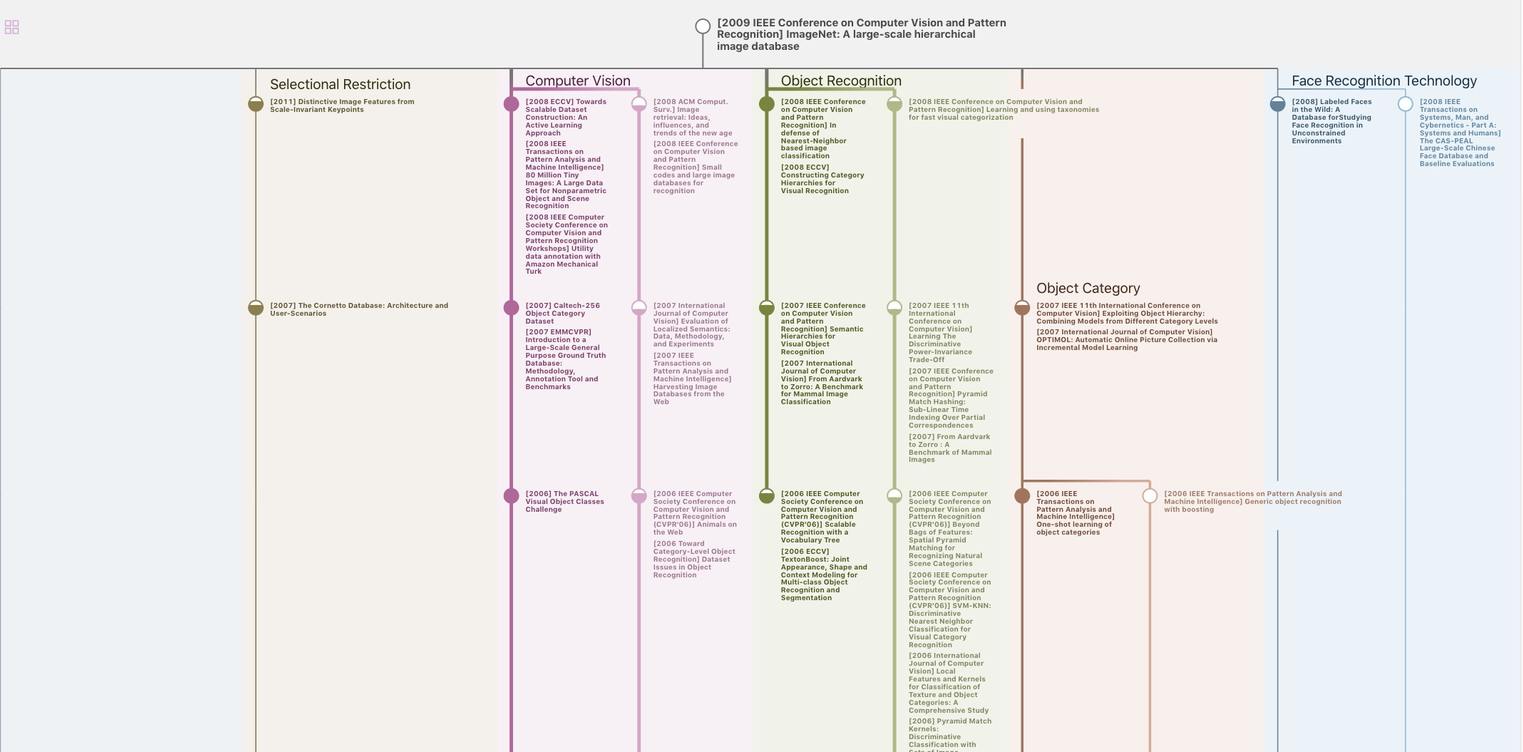
生成溯源树,研究论文发展脉络
Chat Paper
正在生成论文摘要