Internal Model Control Design for Systems Learned by Control Affine Neural Nonlinear Autoregressive Exogenous Models
arXiv (Cornell University)(2024)
Abstract
This paper explores the use of Control Affine Neural Nonlinear AutoRegressiveeXogenous (CA-NNARX) models for nonlinear system identification and model-basedcontrol design. The idea behind this architecture is to match the knowncontrol-affine structure of the system to achieve improved performance.Coherently with recent literature of neural networks for data-driven control,we first analyze the stability properties of CA-NNARX models, devisingsufficient conditions for their incremental Input-to-State Stability(δISS) that can be enforced at the model training stage. The model'sstability property is then leveraged to design a stable Internal Model Control(IMC) architecture. The proposed control scheme is tested on a simulatedQuadruple Tank benchmark system to address the output reference trackingproblem. The results achieved show that (i) the modeling accuracy of CA-NNARXis superior to the one of a standard NNARX model for given weight size andtraining epochs, and (ii) the proposed IMC law provides performance comparableto the ones of a standard Model Predictive Controller (MPC) at a significantlylower computational burden.
MoreTranslated text
Key words
Model-Free Adaptive Control,Nonlinear Systems,Data-Driven Control,Iterative Learning Control,Control Systems
AI Read Science
Must-Reading Tree
Example
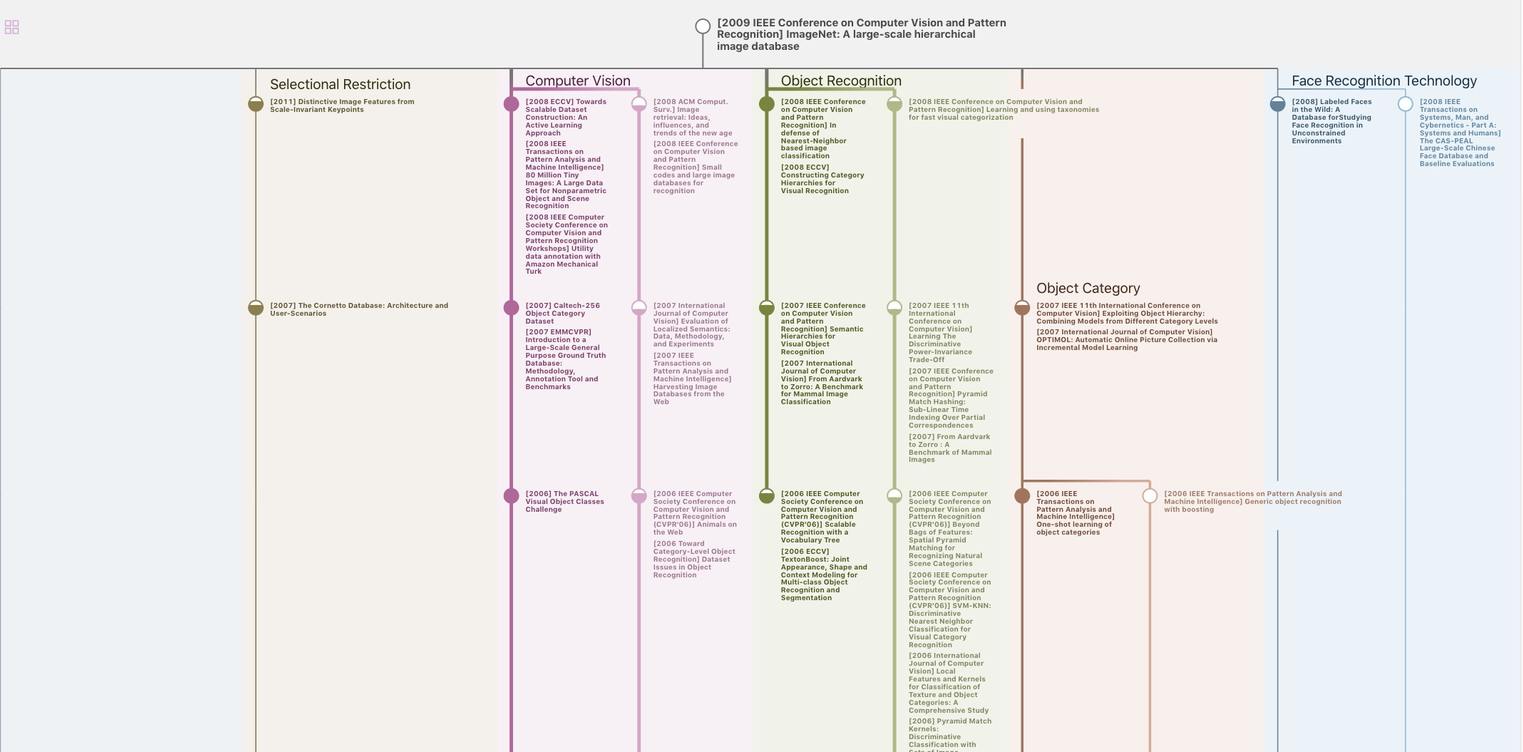
Generate MRT to find the research sequence of this paper
Chat Paper
Summary is being generated by the instructions you defined