Attentive Learning Facilitates Generalization of Neural Networks.
IEEE transactions on neural networks and learning systems(2024)
摘要
This article studies the generalization of neural networks (NNs) by examining how a network changes when trained on a training sample with or without out-of-distribution (OoD) examples. If the network’s predictions are less influenced by fitting OoD examples, then the network learns attentively from the clean training set. A new notion, dataset-distraction stability , is proposed to measure the influence. Extensive CIFAR-10/100 experiments on the different VGG, ResNet, WideResNet, ViT architectures, and optimizers show a negative correlation between the dataset-distraction stability and generalizability. With the distraction stability, we decompose the learning process on the training set $\mathcal{S}$ into multiple learning processes on the subsets of $\mathcal{S}$ drawn from simpler distributions, i.e., distributions of smaller intrinsic dimensions (IDs), and furthermore, a tighter generalization bound is derived. Through attentive learning, miraculous generalization in deep learning can be explained and novel algorithms can also be designed.
更多查看译文
关键词
Deep learning generalization,explainable artificial intelligence (AI),learning mechanism,neural networks (NNs)
AI 理解论文
溯源树
样例
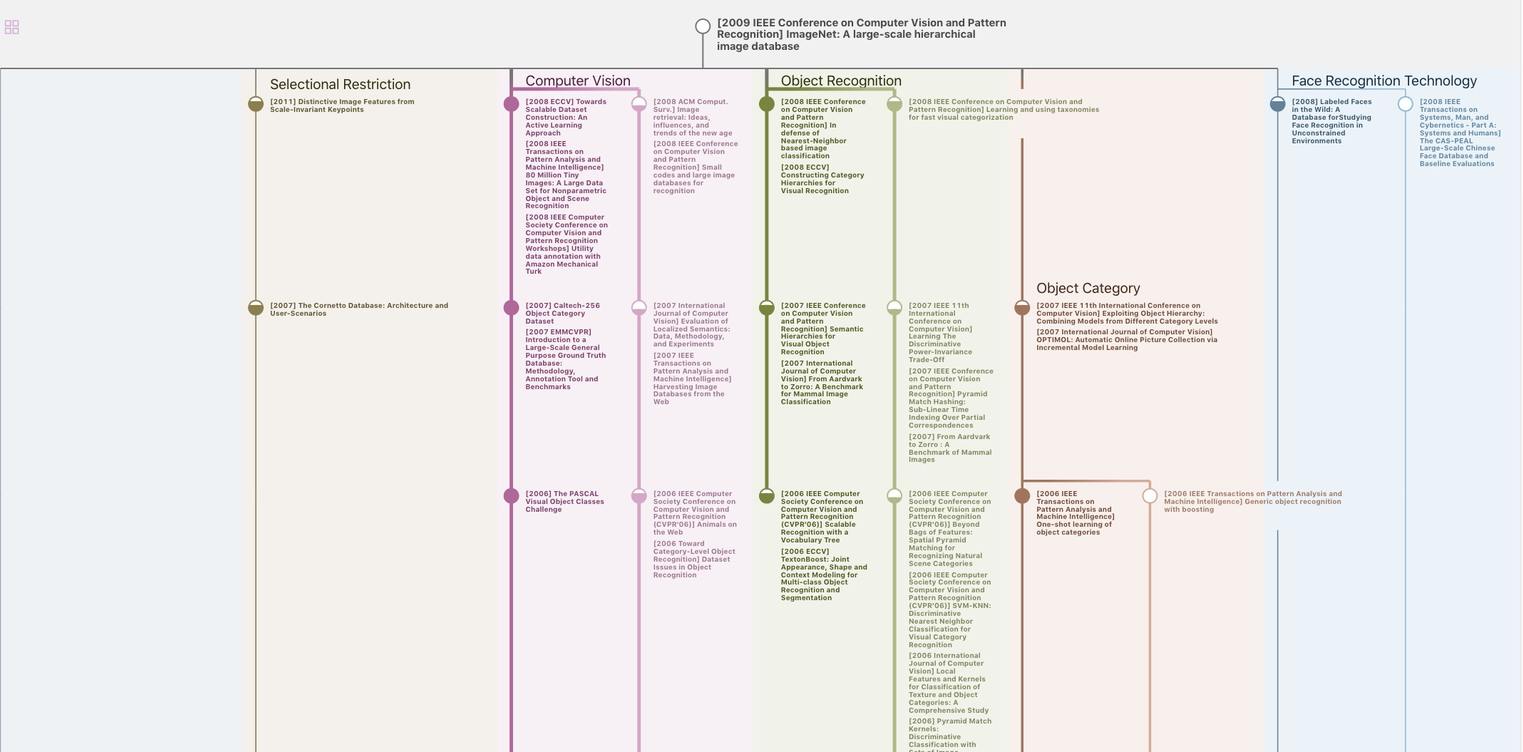
生成溯源树,研究论文发展脉络
Chat Paper
正在生成论文摘要