Navigating Complexity: Toward Lossless Graph Condensation Via Expanding Window Matching.
ICML 2024(2024)
Abstract
Graph condensation aims to reduce the size of a large-scale graph dataset bysynthesizing a compact counterpart without sacrificing the performance of GraphNeural Networks (GNNs) trained on it, which has shed light on reducing thecomputational cost for training GNNs. Nevertheless, existing methods often fallshort of accurately replicating the original graph for certain datasets,thereby failing to achieve the objective of lossless condensation. Tounderstand this phenomenon, we investigate the potential reasons and revealthat the previous state-of-the-art trajectory matching method provides biasedand restricted supervision signals from the original graph when optimizing thecondensed one. This significantly limits both the scale and efficacy of thecondensed graph. In this paper, we make the first attempt towardlossless graph condensation by bridging the previously neglectedsupervision signals. Specifically, we employ a curriculum learning strategy totrain expert trajectories with more diverse supervision signals from theoriginal graph, and then effectively transfer the information into thecondensed graph with expanding window matching. Moreover, we design a lossfunction to further extract knowledge from the expert trajectories. Theoreticalanalysis justifies the design of our method and extensive experiments verifyits superiority across different datasets. Code is released athttps://github.com/NUS-HPC-AI-Lab/GEOM.
MoreTranslated text
AI Read Science
Must-Reading Tree
Example
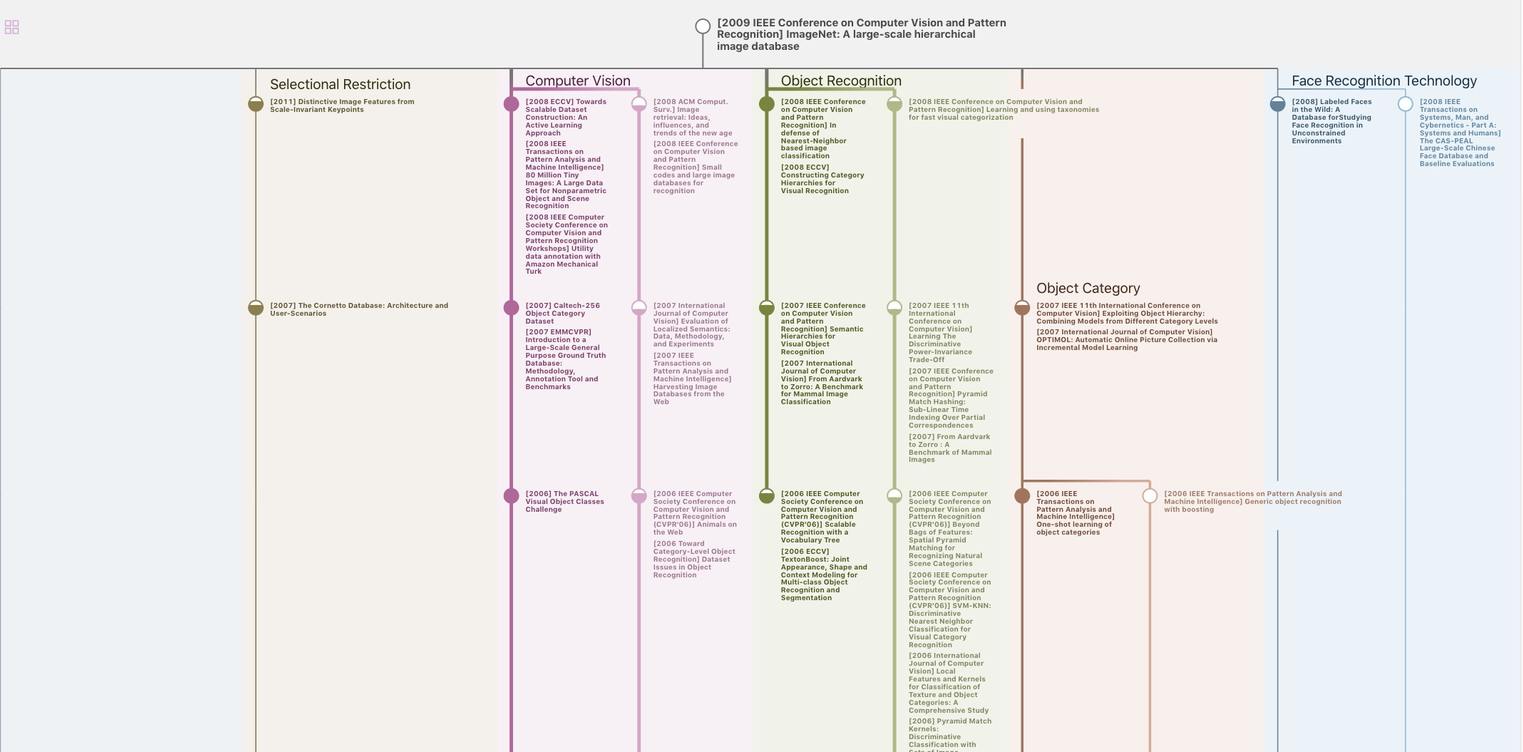
Generate MRT to find the research sequence of this paper
Chat Paper
Summary is being generated by the instructions you defined