Tighter Generalisation Bounds via Interpolation
CoRR(2024)
摘要
This paper contains a recipe for deriving new PAC-Bayes generalisation bounds
based on the (f, Γ)-divergence, and, in addition, presents PAC-Bayes
generalisation bounds where we interpolate between a series of probability
divergences (including but not limited to KL, Wasserstein, and total
variation), making the best out of many worlds depending on the posterior
distributions properties. We explore the tightness of these bounds and connect
them to earlier results from statistical learning, which are specific cases. We
also instantiate our bounds as training objectives, yielding non-trivial
guarantees and practical performances.
更多查看译文
AI 理解论文
溯源树
样例
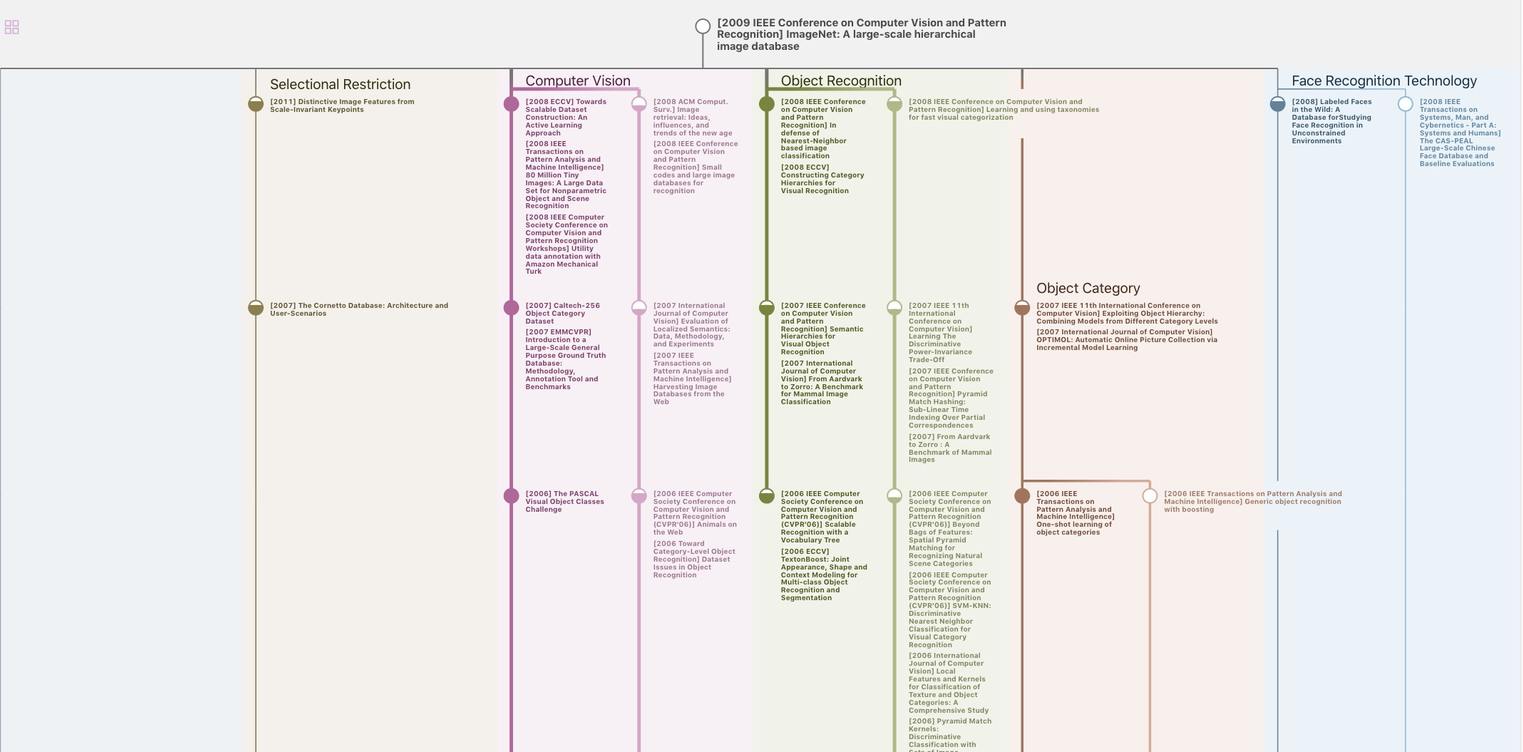
生成溯源树,研究论文发展脉络
Chat Paper
正在生成论文摘要