The Prediction of Crystal Densities of a Big Data Set Using 1D and 2D Structure Features
STRUCTURAL CHEMISTRY(2024)
Abstract
A large data set of over 30 thousand organic compounds containing carbon, nitrogen, oxygen, fluorine, and hydrogen was collected, and the density of each compound was predicted by 1D descriptors derived from its molecular formula and 2D descriptors derived from its constitutional structural features. The 2D structural features are composed of Benson’s groups, corrected groups, and 2D structural features of the whole molecular structures. All the descriptors were extracted by an in-house program in Java with a function to ensure that each atom (or bond) of molecules is represented by Benson’s groups once for atom-based (or bond-based) descriptors. Partial least square (PLS) and random forest (RF) methods were used separately to build models to predict the density. Further, the variable selection of descriptors was performed by variable importance of RF. For partial least square, the combination of the models constructed by descriptors based on the atoms and the bonds achieved the best results in this paper: for the cross-validation of the training set, the Pearson correlation coefficient ( R ) = 0.9270, mean absolute error ( MAE ) = 0.0270 g·cm −3 , and root mean squared error ( RMSE ) = 0.0426 g·cm −3 ; for the prediction of the test set, R = 0.9454, MAE = 0.0263 g·cm −3 , and RMSE = 0.0375 g·cm −3 .
MoreTranslated text
Key words
Density,Quantitative structure–property relationships,Big data set,Partial least squares,Random forest
AI Read Science
Must-Reading Tree
Example
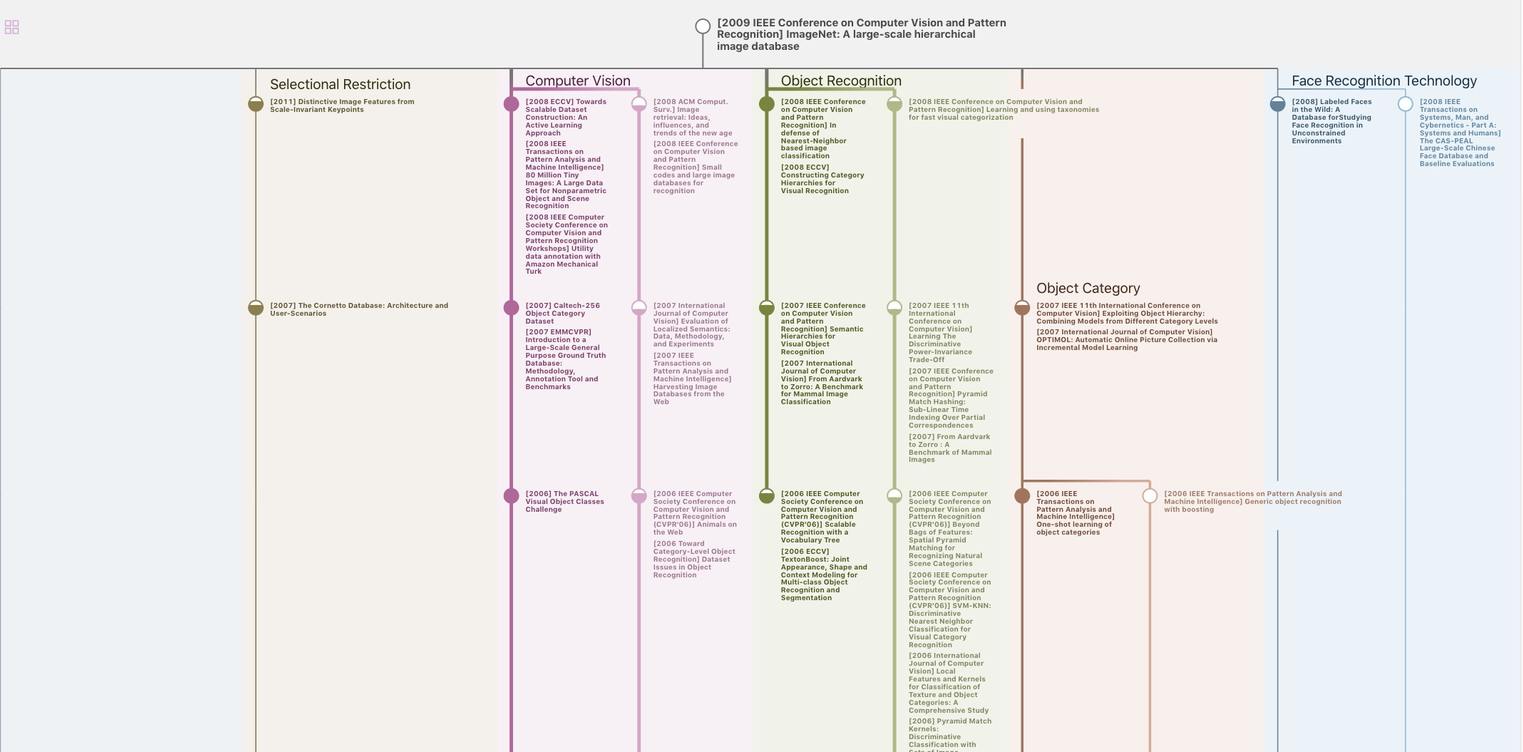
Generate MRT to find the research sequence of this paper
Chat Paper
Summary is being generated by the instructions you defined