Leveraging Uncertainty in Collective Opinion Dynamics with Heterogeneity
CoRR(2024)
摘要
Natural and artificial collectives exhibit heterogeneities across different
dimensions, contributing to the complexity of their behavior. We investigate
the effect of two such heterogeneities on collective opinion dynamics:
heterogeneity of the quality of agents' prior information and of centrality in
the network, i.e., the number of immediate neighbors. To study these
heterogeneities, we not only consider them in our model, proposing a novel
network generator with heterogeneous centrality, but also introduce uncertainty
as an additional dimension. By quantifying the uncertainty of each agent, we
provide a mechanism for agents to adaptively weigh their individual against
social information. As uncertainties develop according to the interactions
between agents, they capture information on heterogeneities. Therefore,
uncertainty is a relevant additional observable in the study of complex
collective opinion dynamics that we use to show the bidirectional relationship
of heterogeneous centrality and information. Furthermore, we demonstrate that
uncertainty-driven adaptive weighting leads to increased accuracy and speed of
consensus, especially under heterogeneity, and provide guidelines for avoiding
performance-decreasing errors in uncertainty modeling. These opportunities for
improved performance and observability suggest the importance of uncertainty
both for the study of natural and the design of artificial heterogeneous
systems.
更多查看译文
AI 理解论文
溯源树
样例
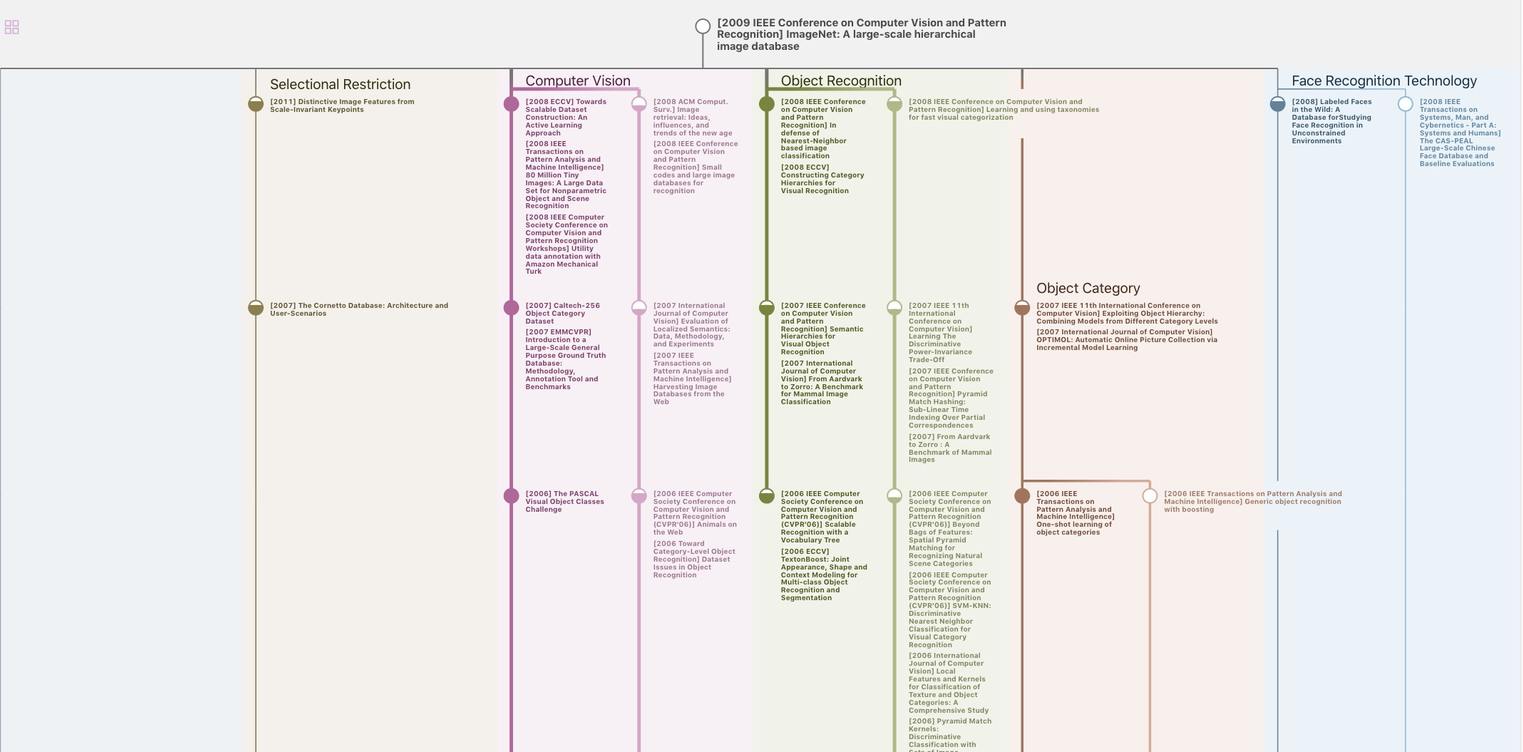
生成溯源树,研究论文发展脉络
Chat Paper
正在生成论文摘要